ICACT20230219 Slide.00
[Big Slide]
[YouTube] |
Chrome Click!! |
 |
Hello everyone, today we would like to present our paper to be accepted in ICACT2023 which will be organized in South Korea, from Feb 19-22. The paper title is "Tracking Risks from Multi-path TDoA-based Localization in Wireless Communications" The authors are Lan-Huong Nguyen, Van-Linh Nguyen, Yu-Hao Liu I am Van-Linh Nguyen, the presenter. |
ICACT20230219 Slide.01
[Big Slide]
[YouTube] |
Chrome Click!! |
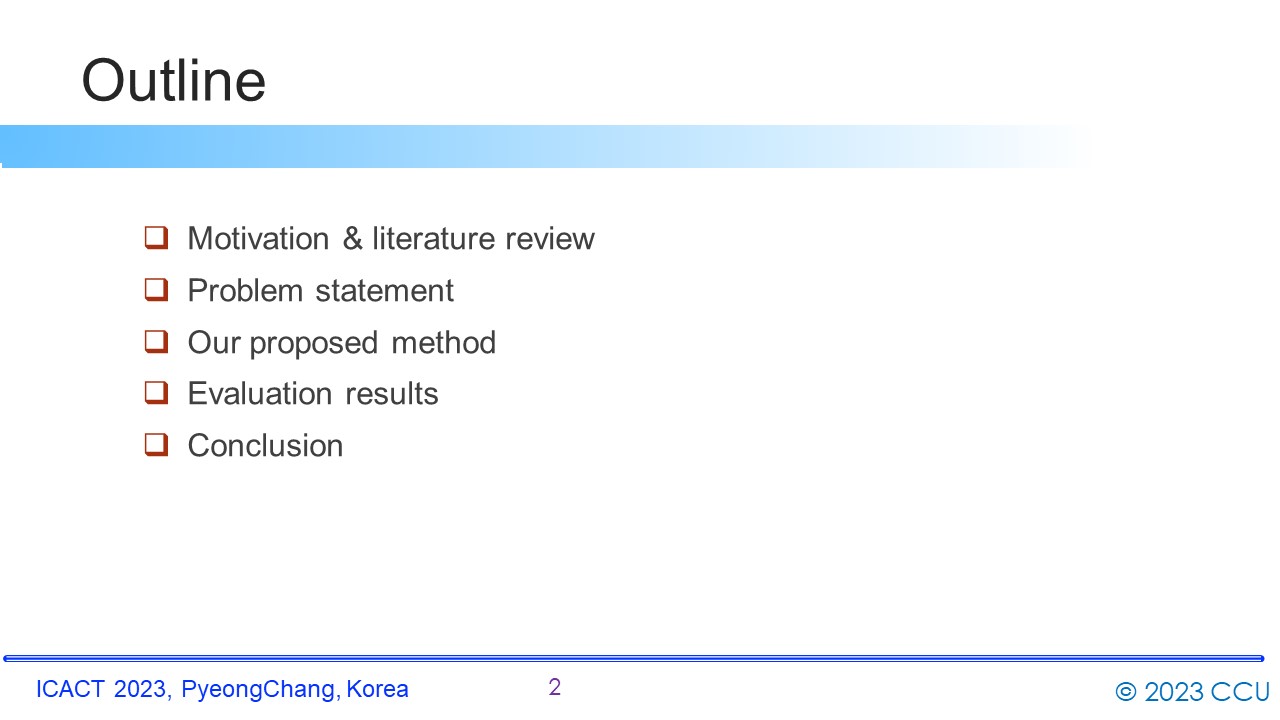 |
The presentation includes five parts: Motivation & literature review; Problem statement; Our proposed method; Evaluation results; Conclusion
|
ICACT20230219 Slide.02
[Big Slide]
[YouTube] |
Chrome Click!! |
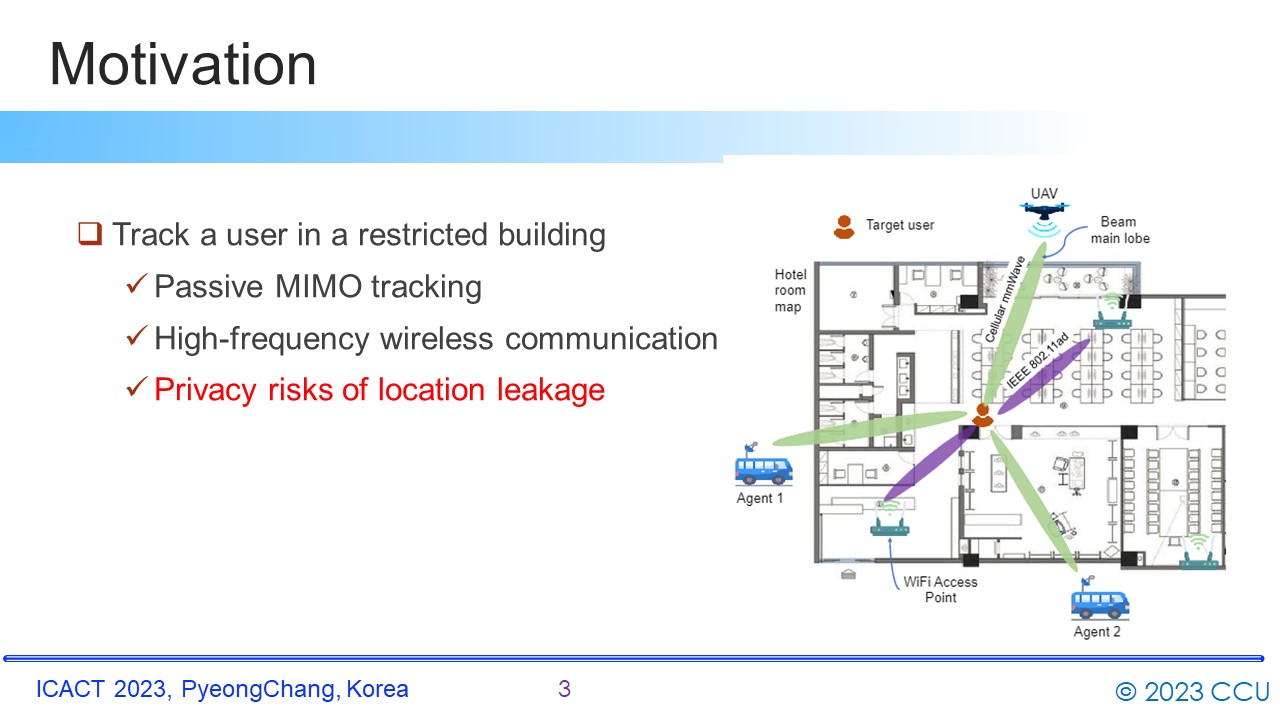 |
Our motivation is to demonstrate the high privacy risks of location leakage from passive signal-based tracking, even in a restricted building. |
ICACT20230219 Slide.03
[Big Slide]
|
Chrome Click!! |
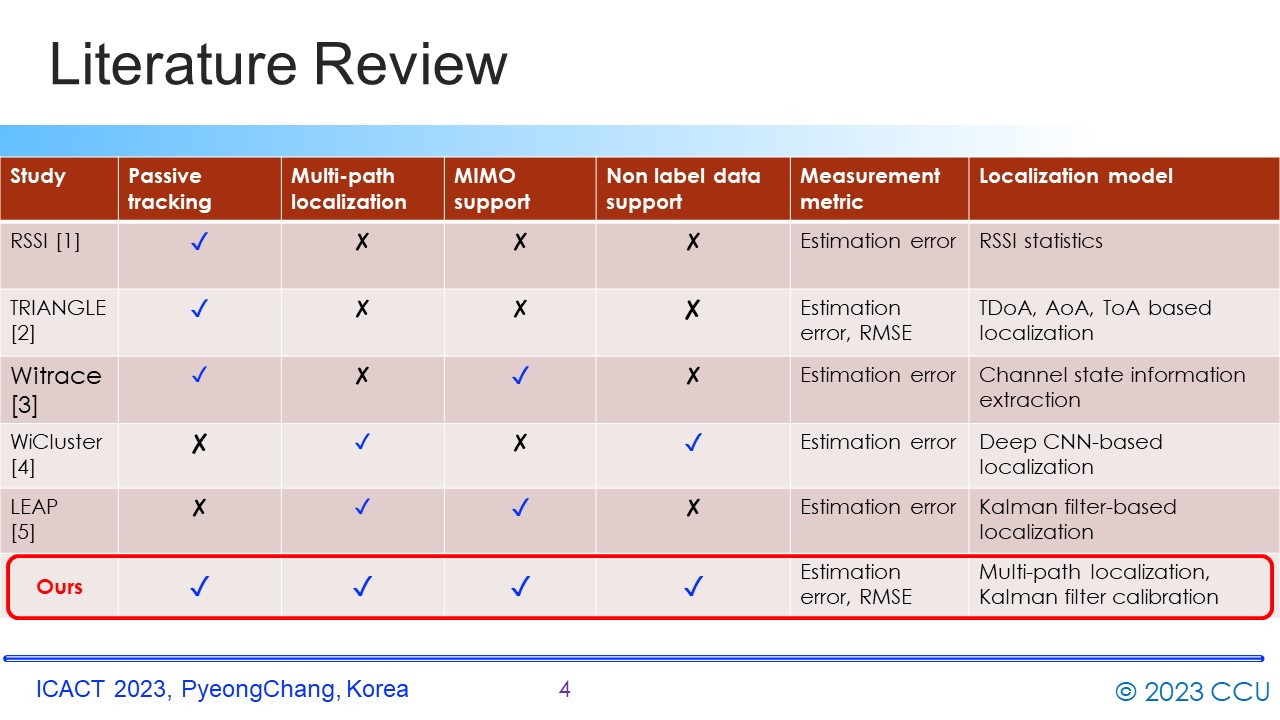 |
Through the literature review, we found that our method differs from the other studies in supporting all features: multi-path localization for MIMO communications, even with non-labeled data. Our model also supports Kalman filter calibration to enhance the tracking performance
|
ICACT20230219 Slide.04
[Big Slide]
|
Chrome Click!! |
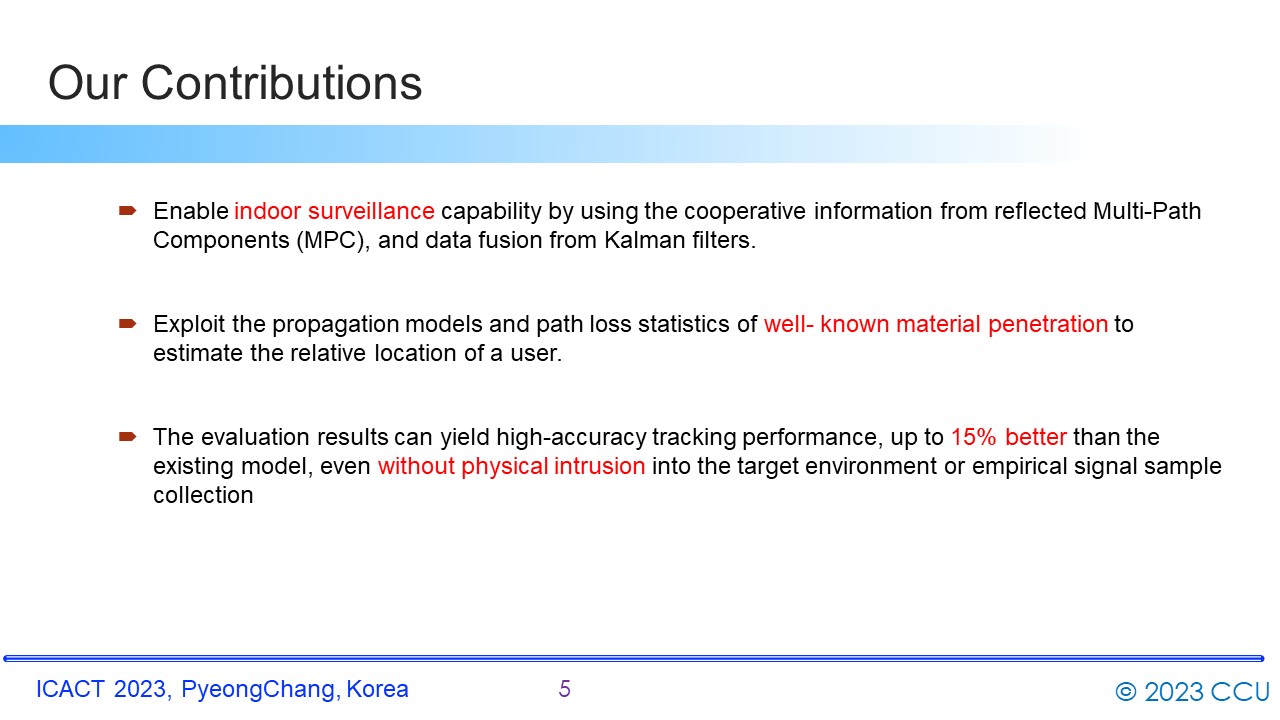 |
Our contributions include:
1. Enable indoor surveillance capability by using the cooperative information from reflected Multi-Path Components (MPC), and data fusion from Kalman filters.
2. Exploit the propagation models and path loss statistics of well-known material penetration to estimate the relative location of a user.
3. The evaluation results can yield high-accuracy tracking performance, up to 15% better than the existing model, even without physical intrusion into the target environment or empirical signal sample collection
|
ICACT20230219 Slide.05
[Big Slide]
|
Chrome Click!! |
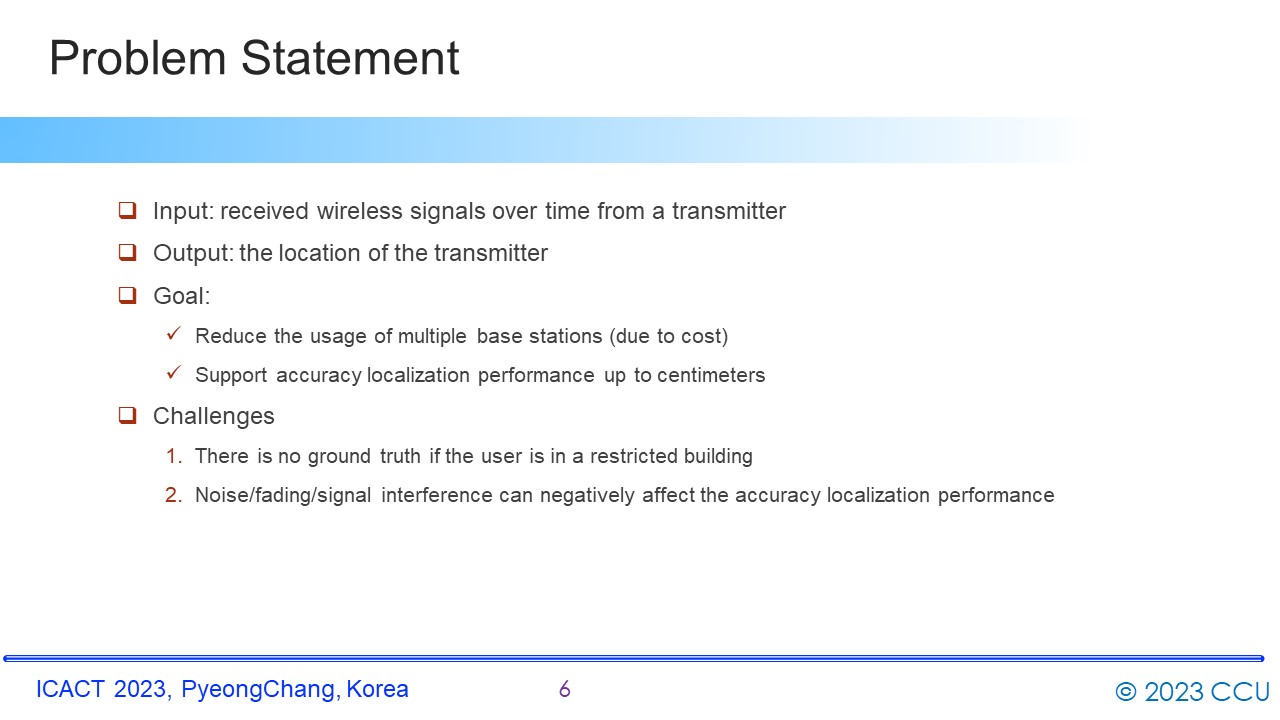 |
In our problem:
The inputs are received wireless signals over time from a transmitter
The output is the location of the transmitter
However, the challenges are: (1) There is no ground truth if the user is in a restricted building; (2) Noise/fading/signal interference can negatively affect the accuracy localization performance
|
ICACT20230219 Slide.06
[Big Slide]
|
Chrome Click!! |
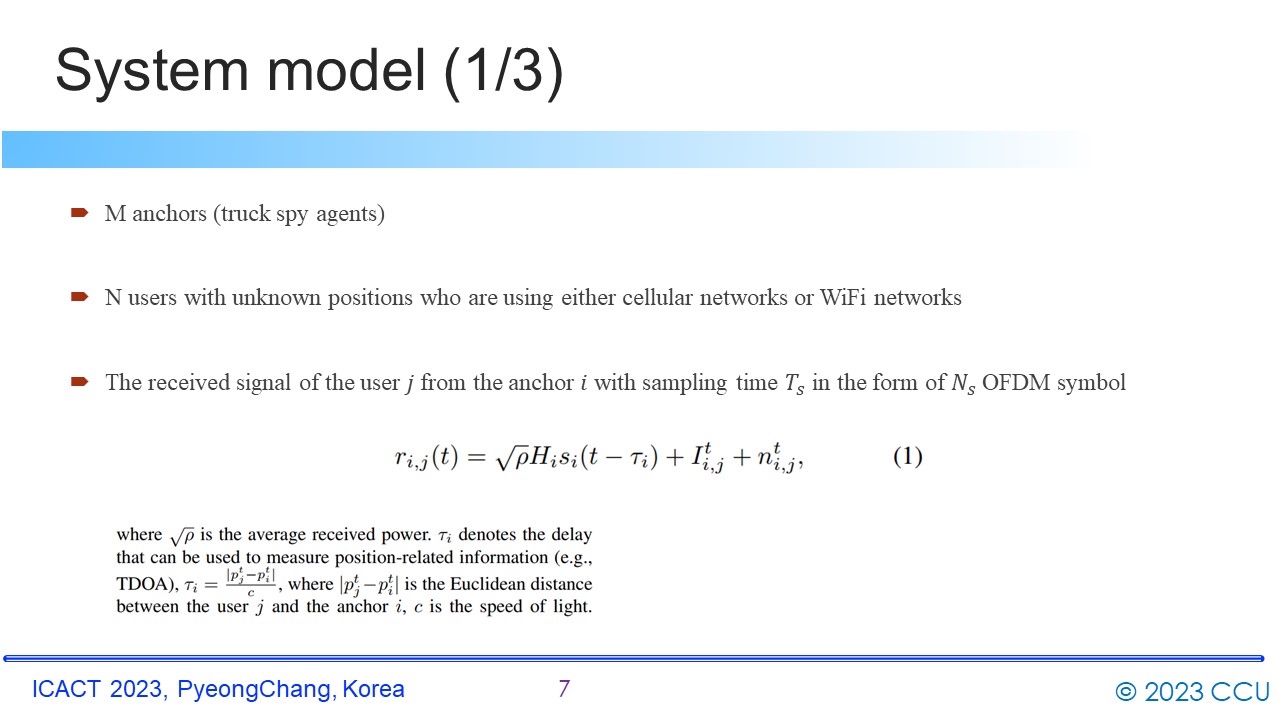 |
There are M anchors (spy agents) and N users. The goal is to determine a special user j in N users.
The received signal of the user π from the anchor π with sampling time π_π in the form of π_π OFDM symbol as formula (1)
|
ICACT20230219 Slide.07
[Big Slide]
|
Chrome Click!! |
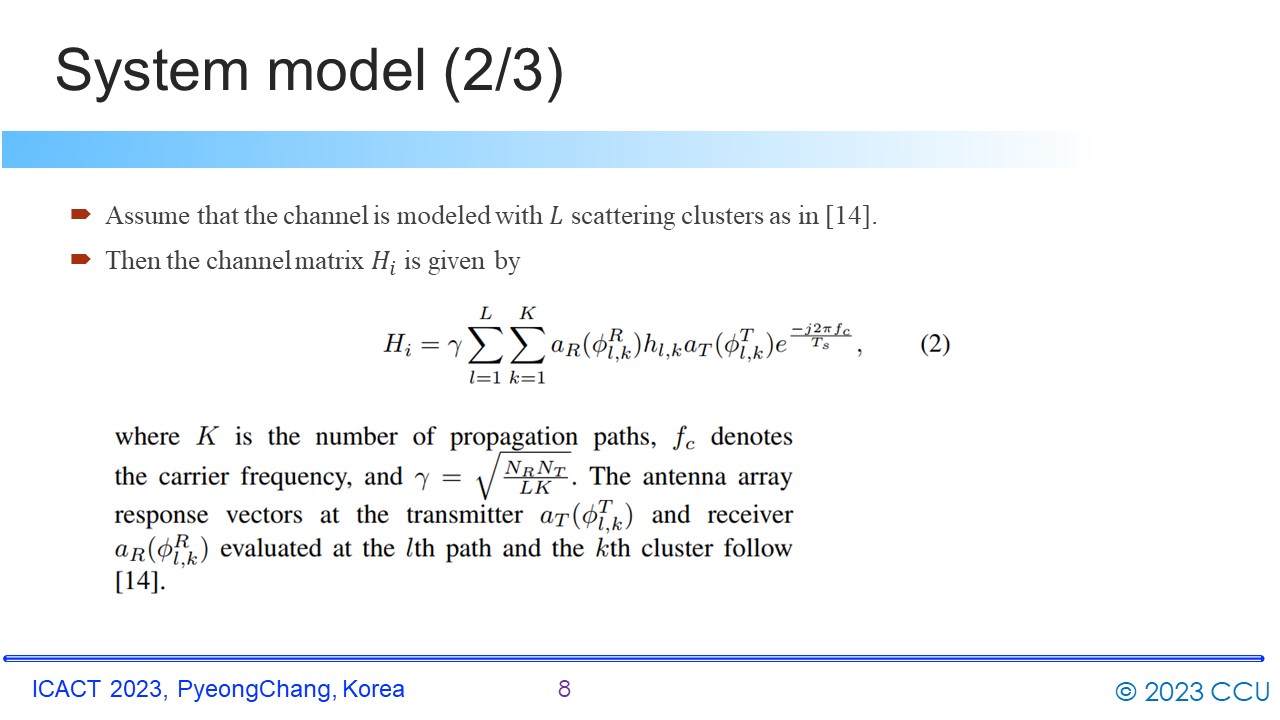 |
Then the channel matrix π»_π with L scattering clusters and K propagation path is modeled as Formula 2
|
ICACT20230219 Slide.08
[Big Slide]
|
Chrome Click!! |
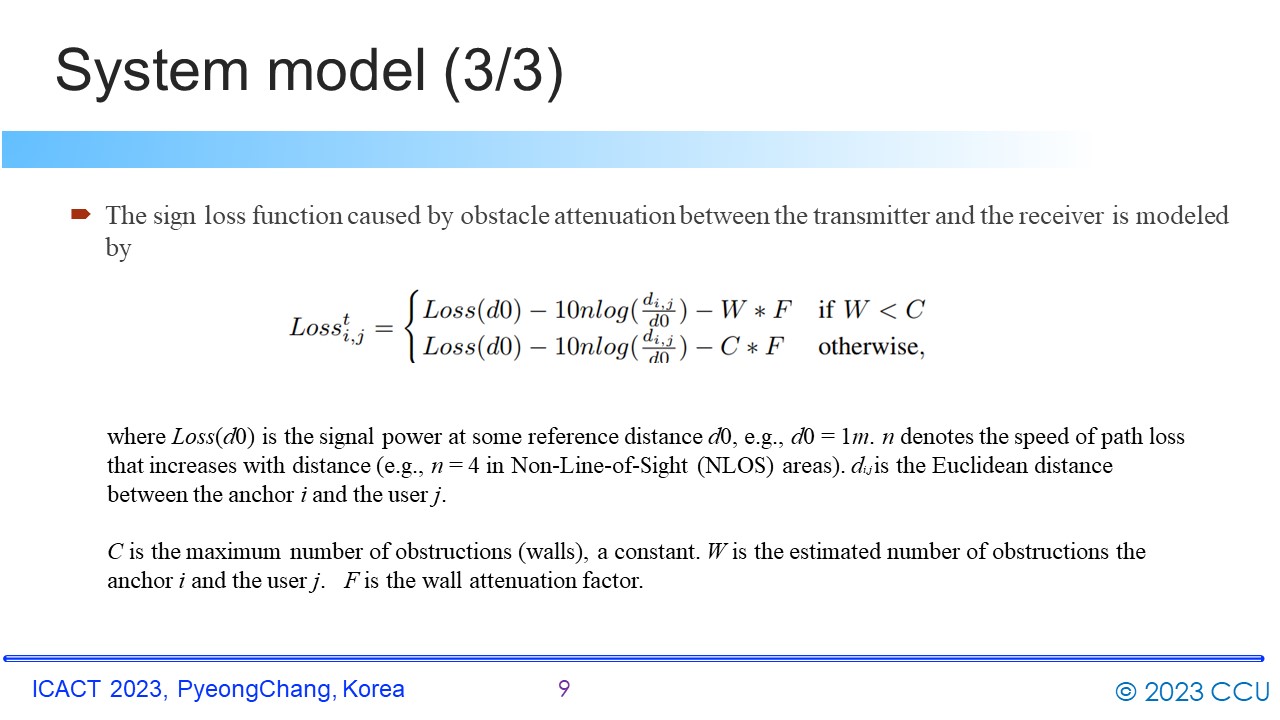 |
The sign loss function caused by obstacle attenuation between the transmitter and the receiver is modeled as Formula on the figure
|
ICACT20230219 Slide.09
[Big Slide]
|
Chrome Click!! |
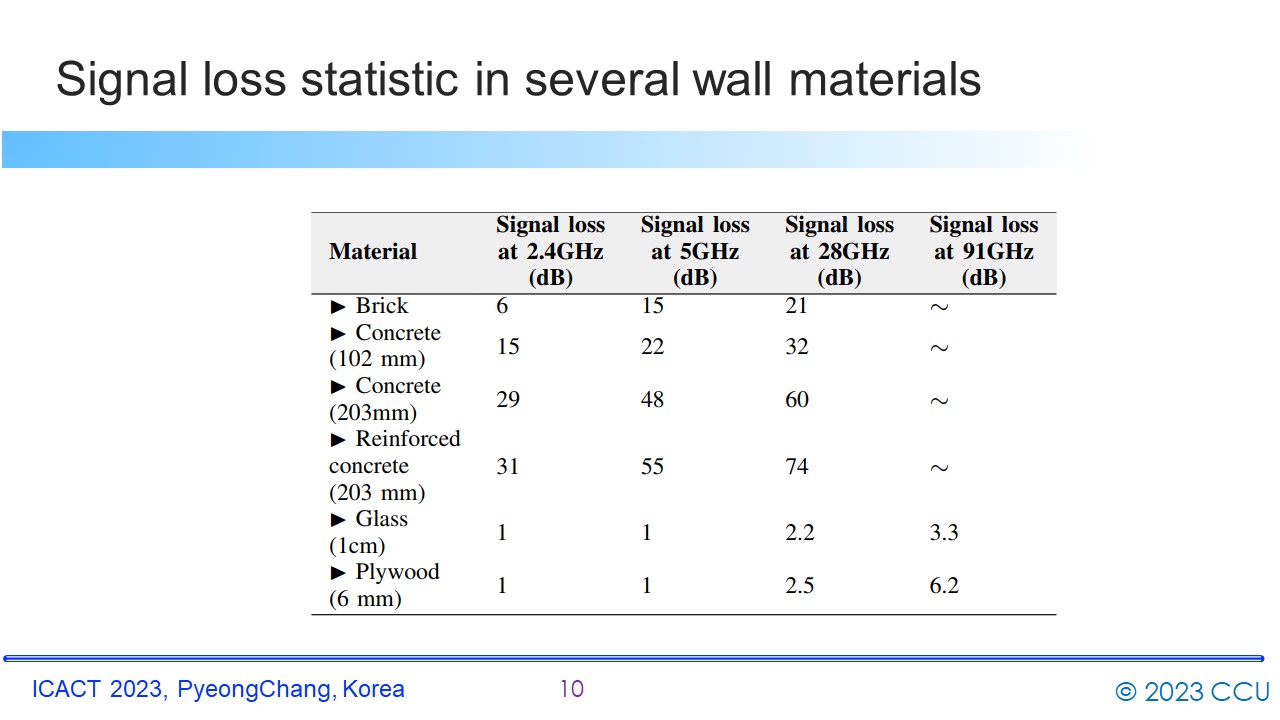 |
Here are the statistical values for signal loss when testing with several wall materials. Accordingly, the thicker concrete walls can prevent the signal go through significantly. |
ICACT20230219 Slide.10
[Big Slide]
|
Chrome Click!! |
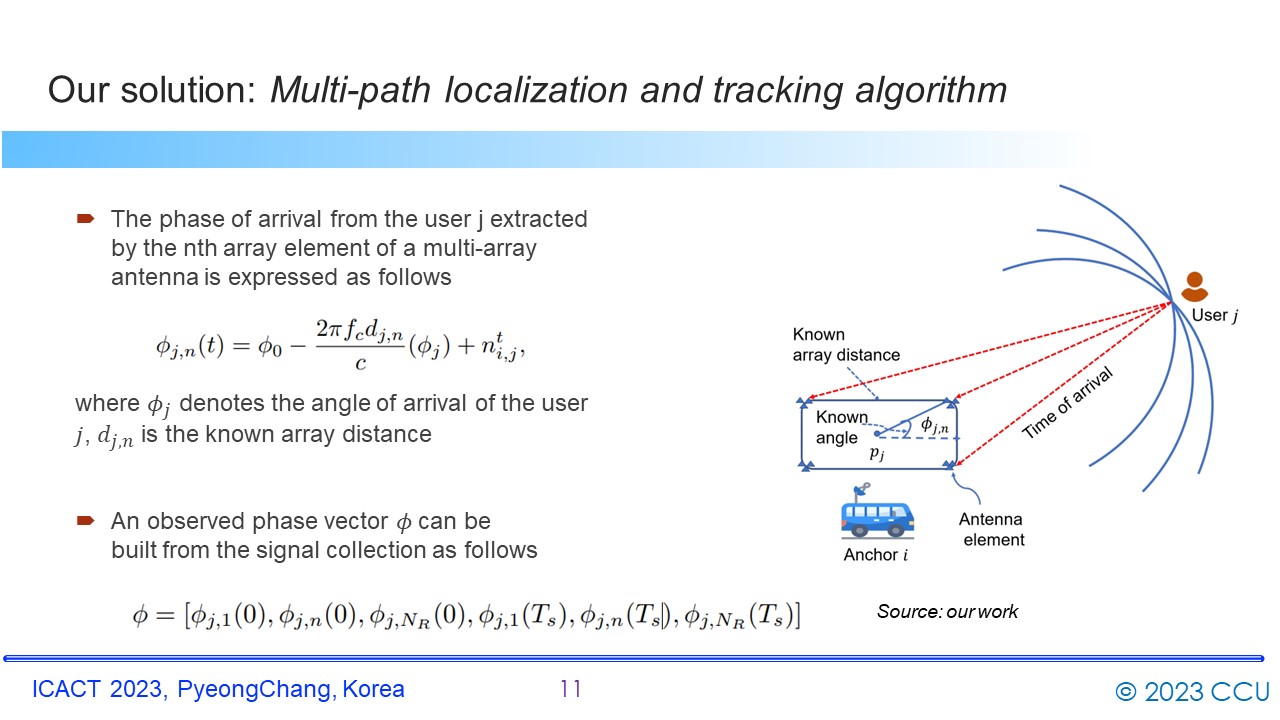 |
Our solution is to extract the phase of arrival or time of arrival of received signal beams and monitor them over time.
Based on the training data, we can determine the relative location of a user |
ICACT20230219 Slide.11
[Big Slide]
|
Chrome Click!! |
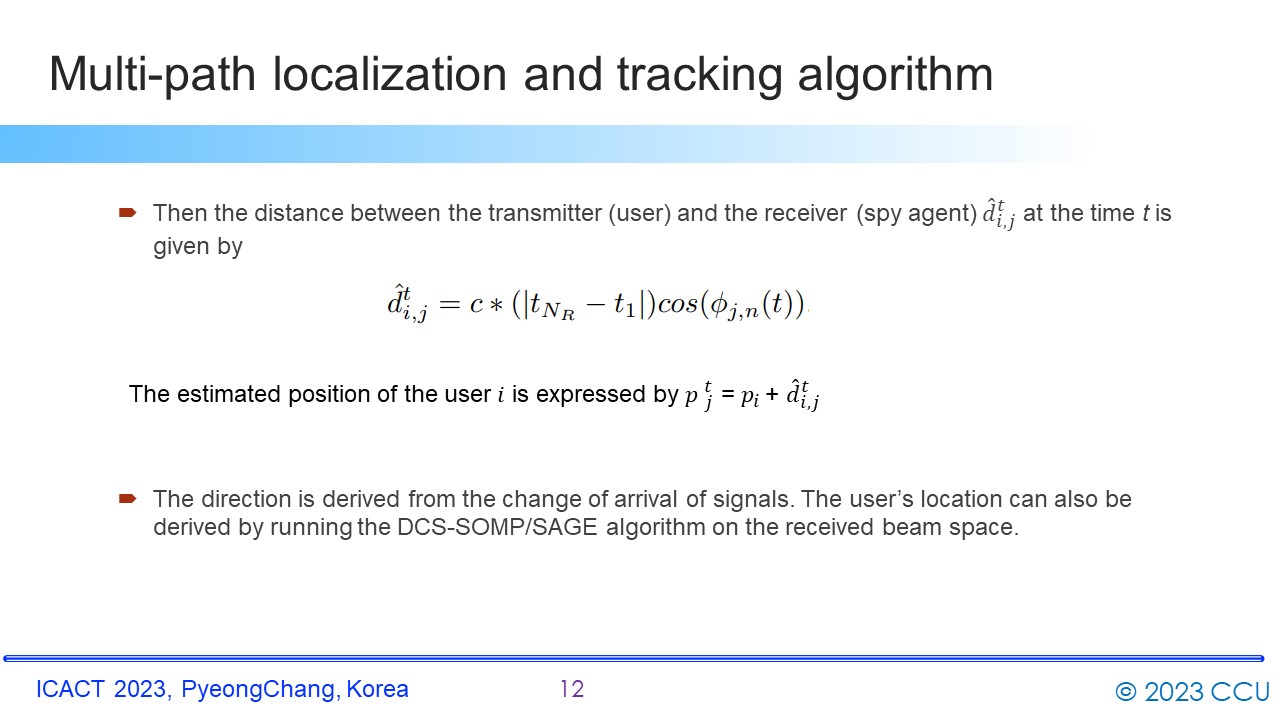 |
TDoA and AoA can be derived from the change of arrival of signals. The userβs location can also be derived by running the DCS-SOMP/SAGE algorithm on the received beam space.
|
ICACT20230219 Slide.12
[Big Slide]
|
Chrome Click!! |
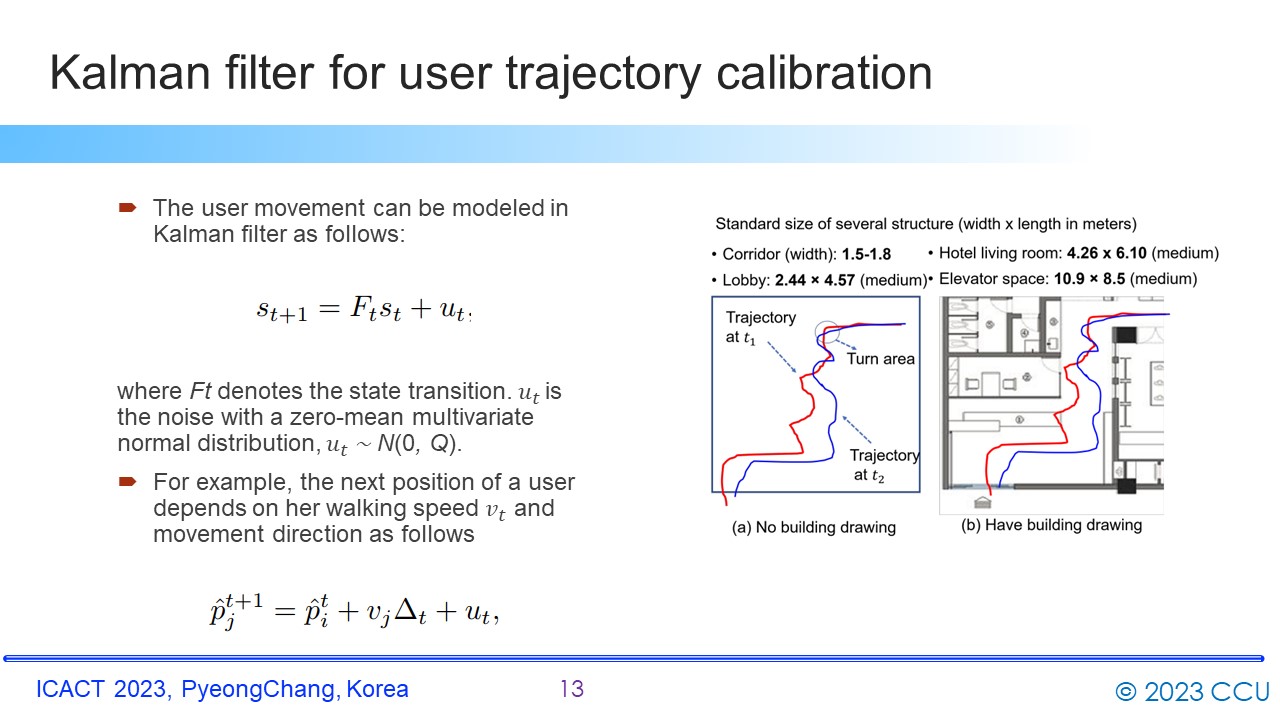 |
To address the outlier points, we use Kalman filter to correlate the consistency of the predicted data points by applying motion models to predict the next position of the user based on the past position.
For example, the next position of a user depends on her walking speed π£_π‘ and movement direction as shown in the formula at the bottom of the figure.
|
ICACT20230219 Slide.13
[Big Slide]
|
Chrome Click!! |
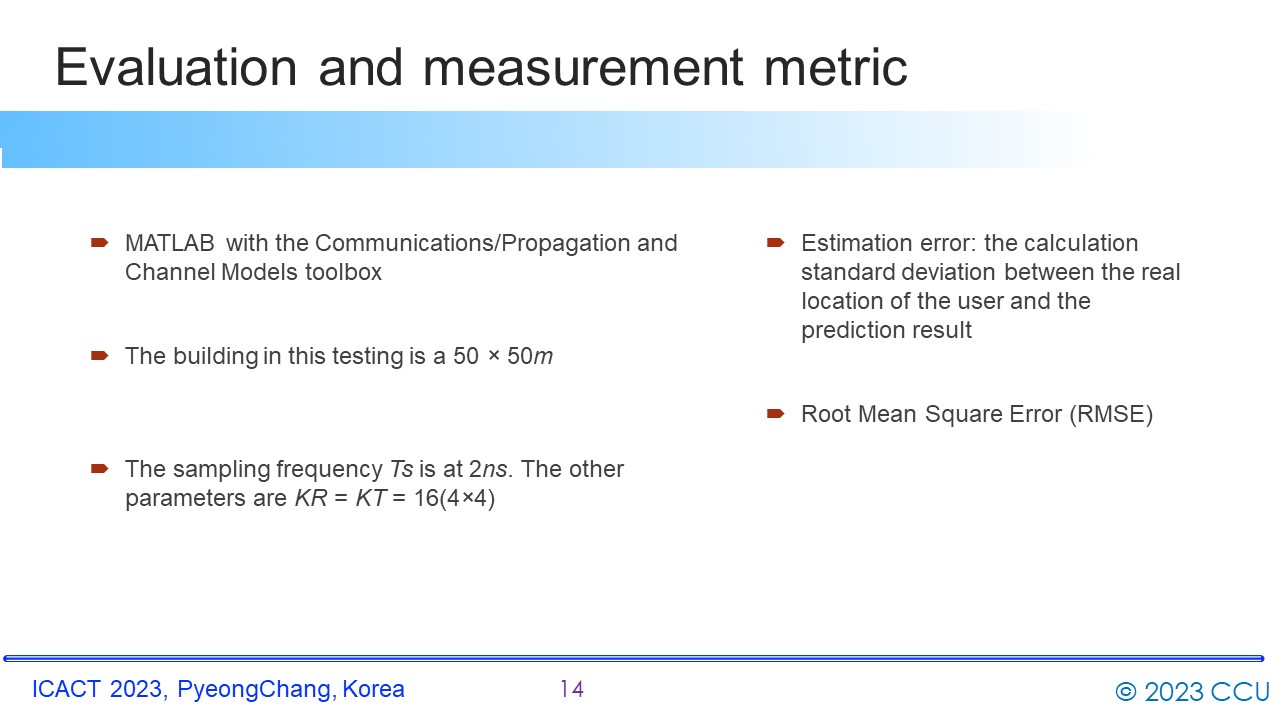 |
We evaluate the proposed model with MATLAB Communications/Propagation and Channel Models toolbox
The building in this testing is a 50 Γ 50m
The sampling frequency Ts is at 2ns. The otherparameters are number of antenna array at the transmitter and the receiver is equal = KR = KT = 16(4Γ4)
We use Root Mean Square Error (RMSE) as the main estimation error measurement metric.
|
ICACT20230219 Slide.14
[Big Slide]
|
Chrome Click!! |
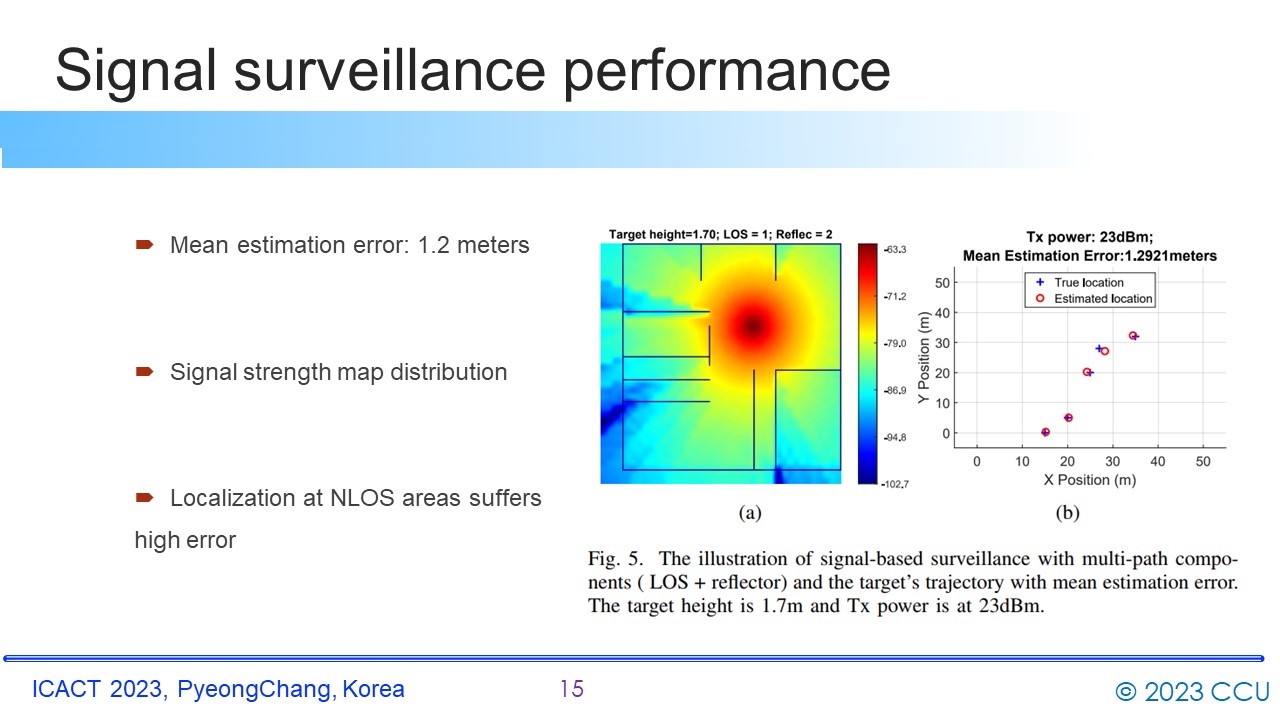 |
Mean estimation error for tracking is around 1.2 meters.
Also, as shown in Figure 5.b, localization at NLOS areas suffers
a high error. The signal strength distribution and the relative position of the transmitter is shown in Figure 5.a
|
ICACT20230219 Slide.15
[Big Slide]
|
Chrome Click!! |
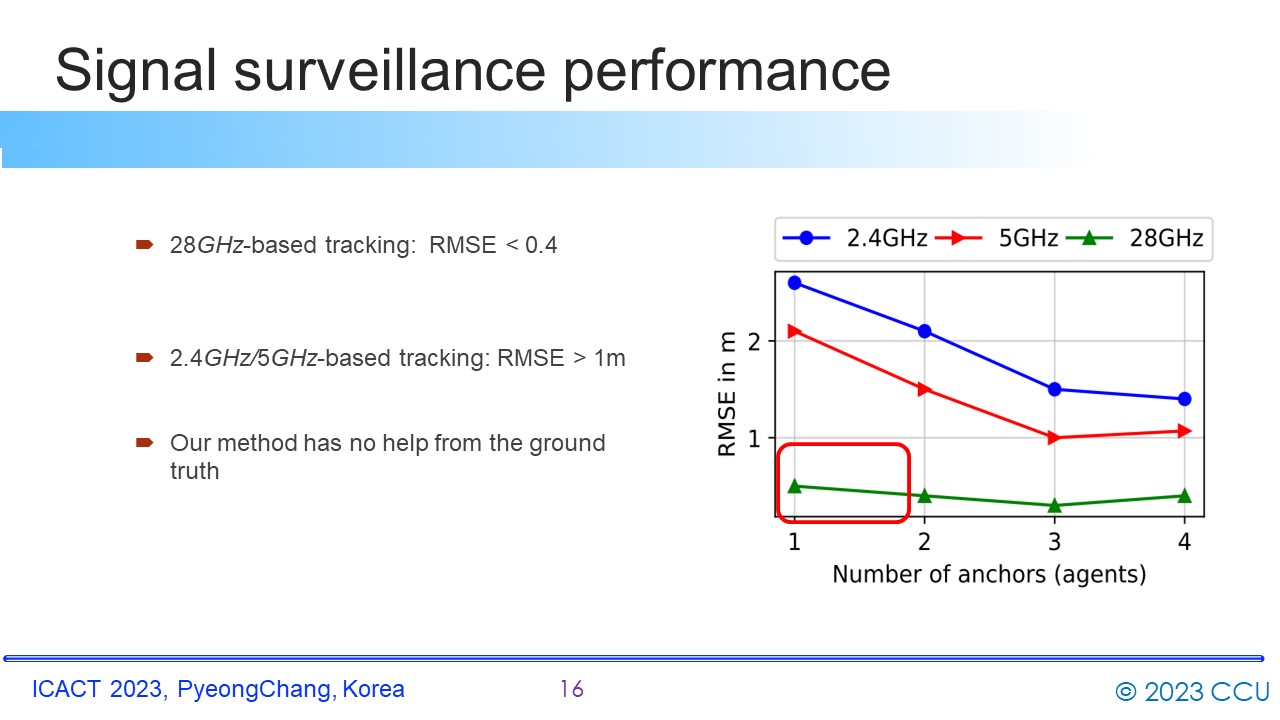 |
High-frequency wireless communications like 28GHz often support higher localization performance, e.g., RMSE < 0.4. This is because the directional information from this type is rich and easy to classify.
Note that our method has no help from the ground truth so the estimation error is much higher than in some fingerprinted-based localization methods.
|
ICACT20230219 Slide.16
[Big Slide]
|
Chrome Click!! |
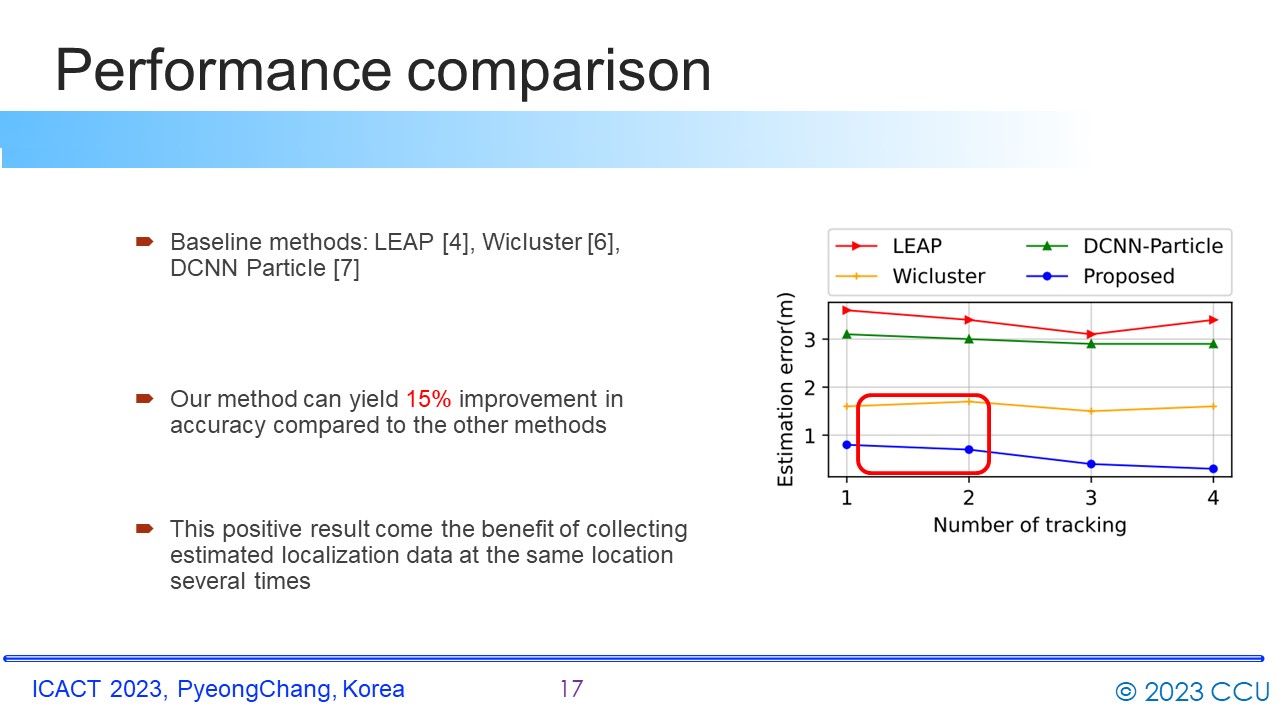 |
We use three Baseline methods: LEAP [4], Wicluster [6], DCNN Particle [7] to compare the performance.
According to the results, we found that our method can yield 15% improvement in accuracy compared to the other methods. This positive result comes the benefit of collecting estimated localization data at the same location several times
|
ICACT20230219 Slide.17
[Big Slide]
|
Chrome Click!! |
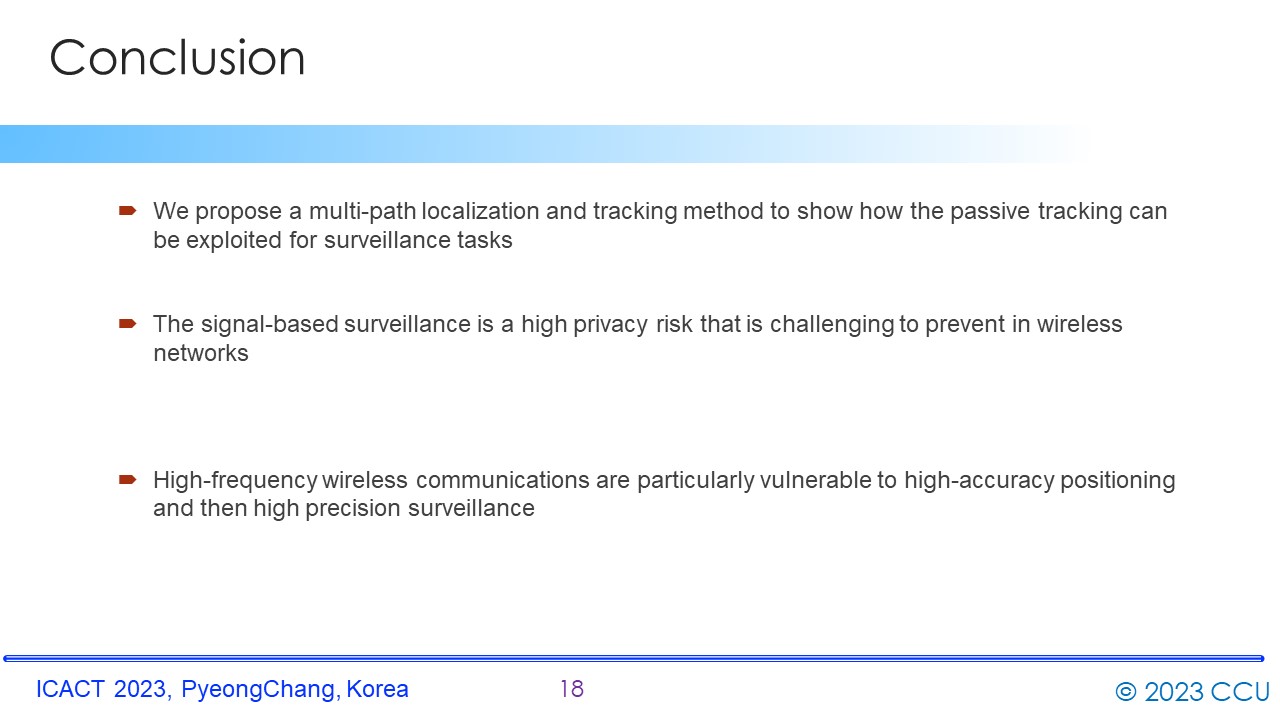 |
In conclusion,
We propose a multi-path localization and tracking method to show how passive tracking can be exploited for surveillance tasks.
The signal-based surveillance is a high privacy risk that is challenging to prevent in wireless networks.
High-frequency wireless communications are particularly vulnerable to high-accuracy positioning and then high precision surveillance
|
ICACT20230219 Slide.18
[Big Slide]
|
Chrome Click!! |
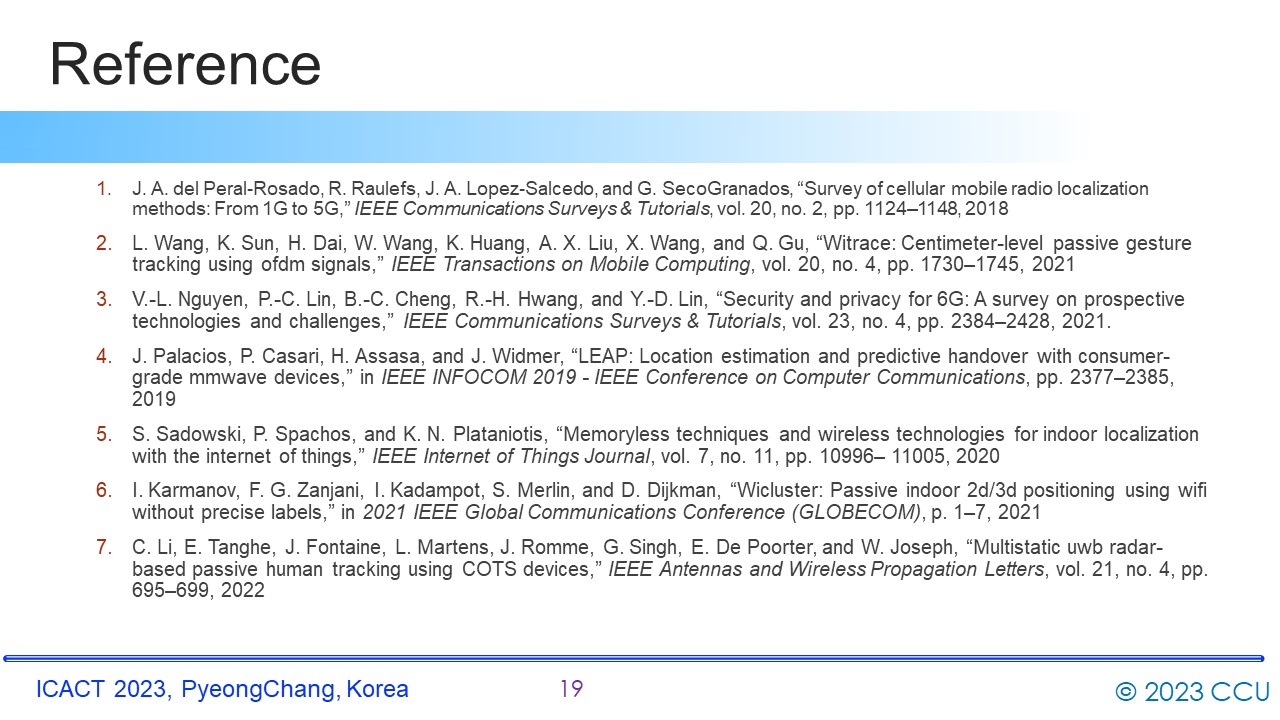 |
Here are our references,
Thank you everyone for watching. |