ICACT20230149 Slide.17
[Big slide for presentation]
|
Chrome Text-to-Speach Click!! |
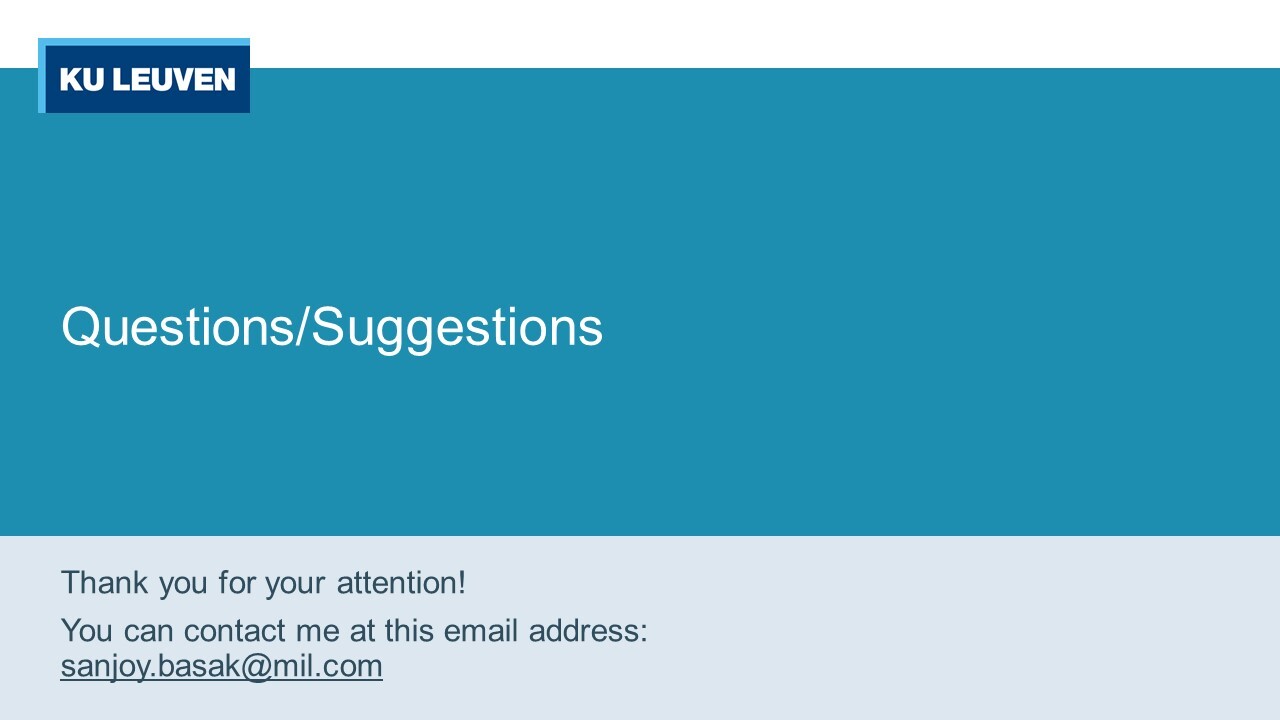 |
This concludes my presentation. Thank you very much all for your kind attention. If you have any questions, you can ask me after the presentation or send me an email at this email address. Thank you!
|
ICACT20230149 Slide.16
[Big slide for presentation]
|
Chrome Text-to-Speach Click!! |
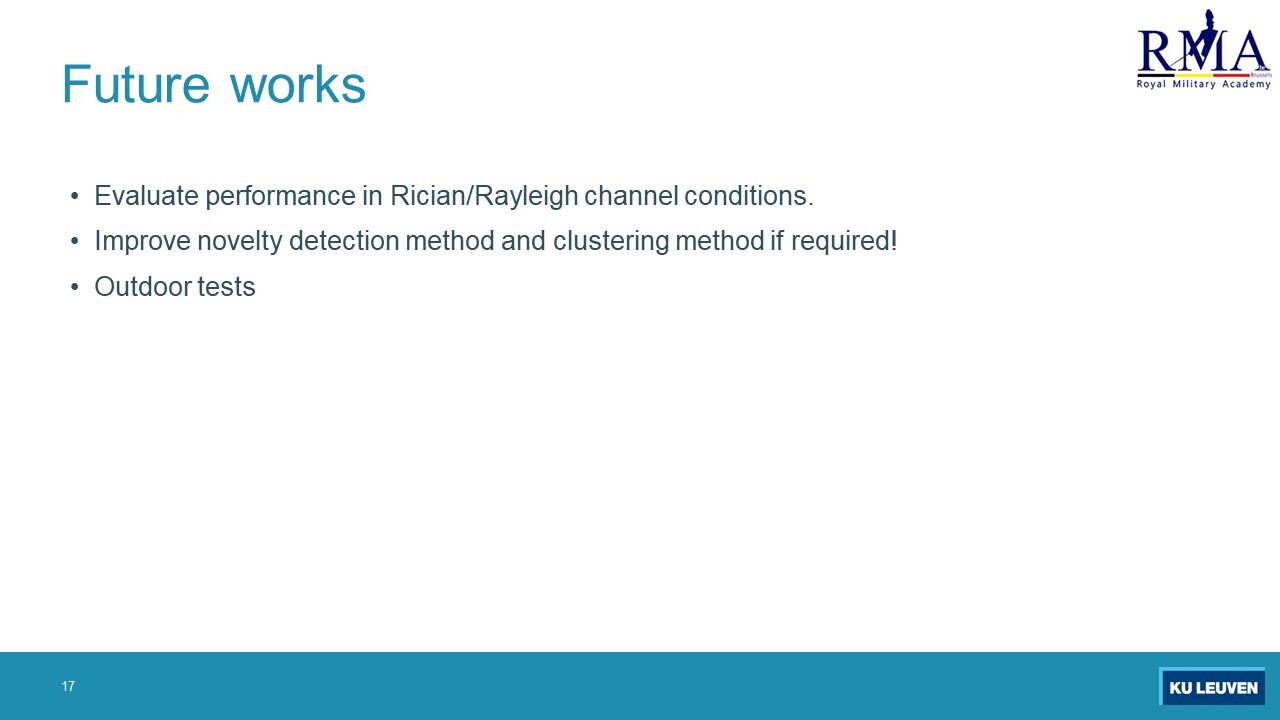 |
In the future, we are going to evaluate the novelty detection performance in real-life Rician/Rayleigh channel conditions. Furthermore, we will perform outdoor tests for the evaluation. We are also investigating other novelty detection and clustering method to see if we can further improve the performance.
|
ICACT20230149 Slide.15
[Big slide for presentation]
|
Chrome Text-to-Speach Click!! |
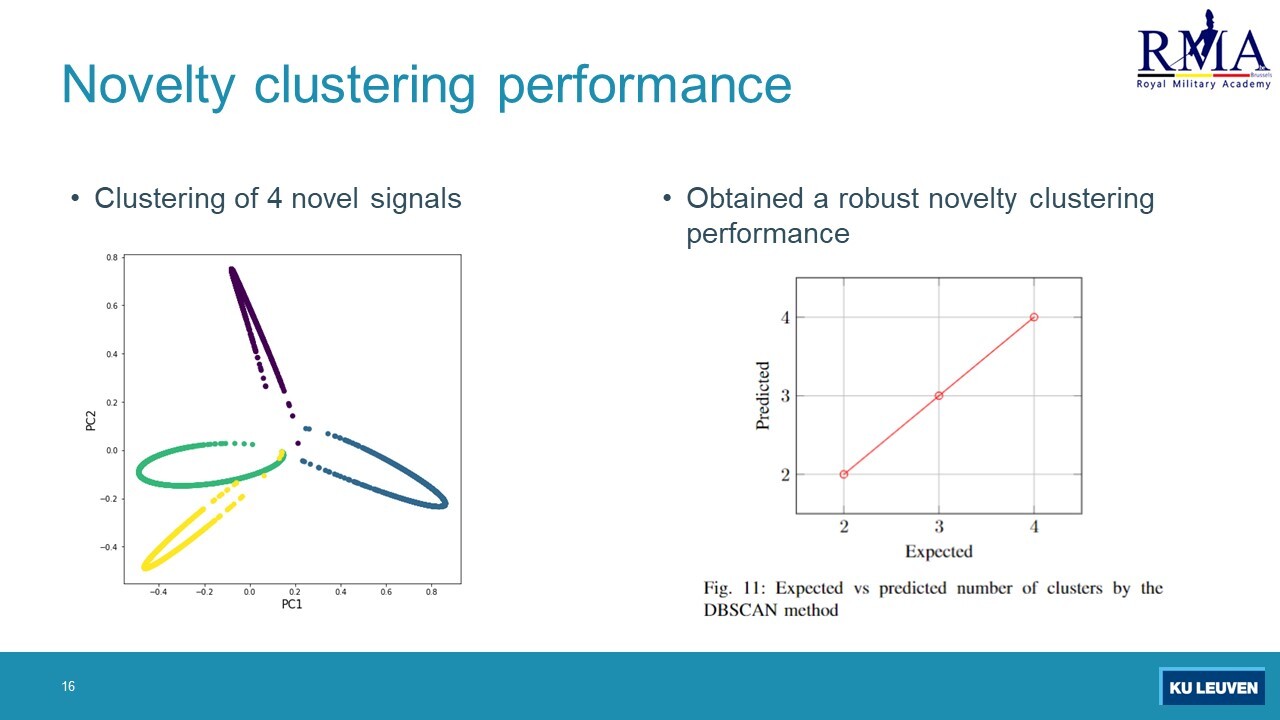 |
In this slide, I showed the novelty clustering performance. This is the principal component analysis (PCA) plot. From the figure, it can be clearly understood that the unseen drone signals can be easily clustered using a simple clustering algorithm.
As I mentioned before, we used the DBSCAN algorithm for clustering. With dBscan we obtained a robust clustering performance. The expected and predicted numbers of clusters are plotted in this figure. We varied the number of unseen signals to evaluate the clustering performance. As you can see from the figure, we obtained a robust novel signal clustering performance.
|
ICACT20230149 Slide.14
[Big slide for presentation]
|
Chrome Text-to-Speach Click!! |
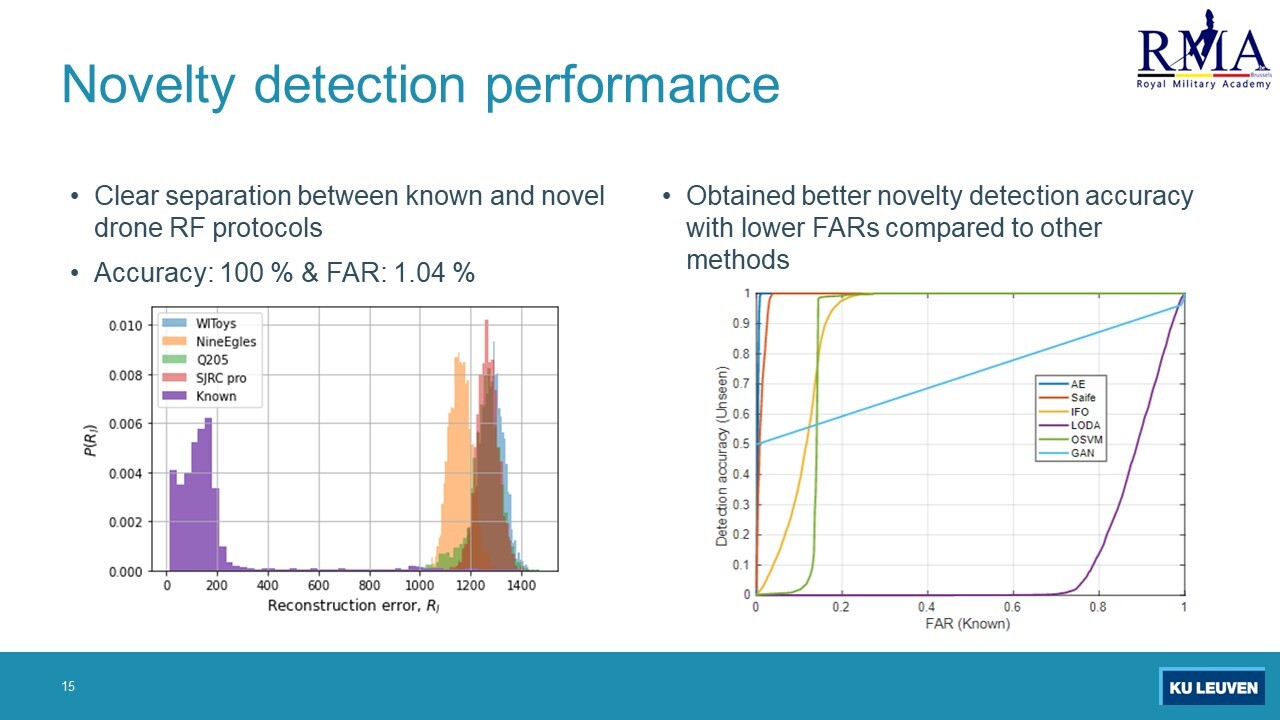 |
Here we plotted the distribution/PDF plot of the reconstruction error to show the novelty detection performance. As you can see we obtained a clear separation between the reconstruction error of known and novel signals. We used an n-sigma thresholding approach. We determine the threshold from the mean and standard deviation of the reconstruction error of the known signals. This simple approach worked quite well for novelty detection in this case.
We also compared the novelty detection performance of our framework against the SoA algorithms such as Saife, GAN (generative adversarial network), OSVM (one class SVM), IFO (isolation forest), and LODA (Lightweight on-line detector of anomalies). Saife provided a close novelty detection performance compared to our AE-based model. Saife is also a reconstruction-based method. With our proposed framework, we obtained 100% novelty detection accuracy at 1.04% FAR on the known signals whereas Saife provided 3.81% FAR. The IFO and OSVM provided similar performances. The GAN and Loda provided worse performance compared to other frameworks.
|
ICACT20230149 Slide.13
[Big slide for presentation]
|
Chrome Text-to-Speach Click!! |
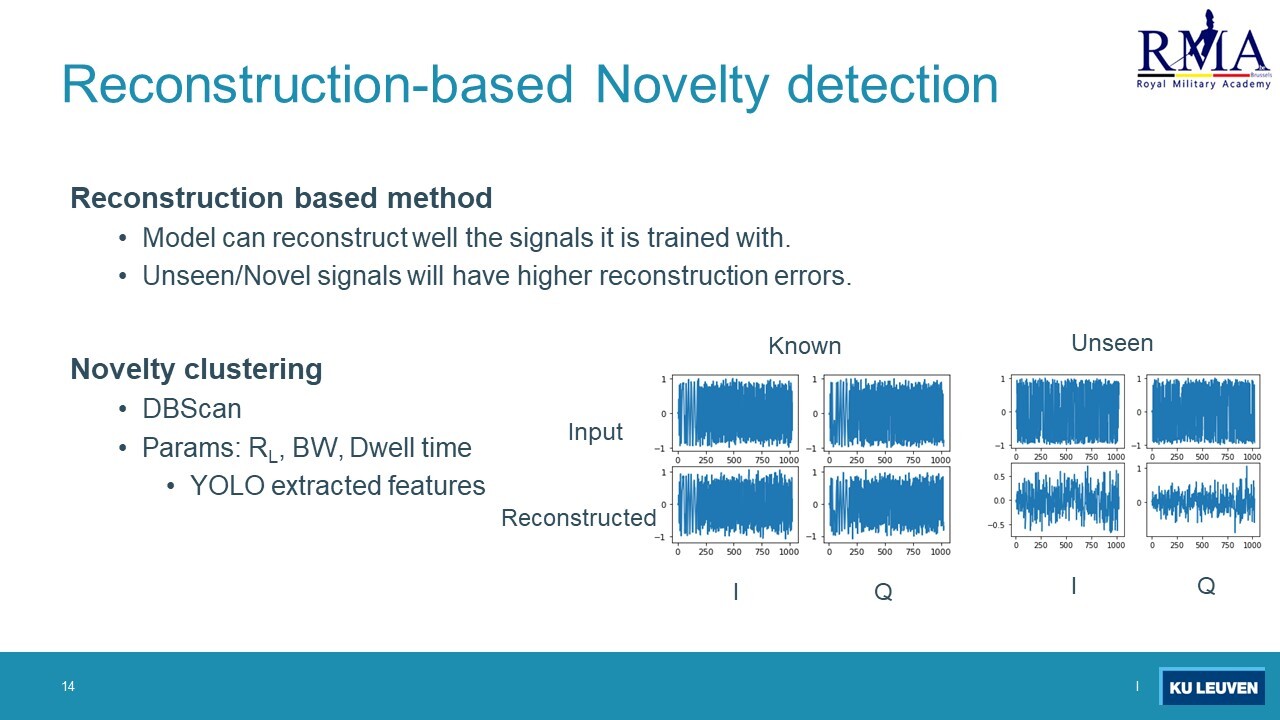 |
In this slide I showed how the reconstruction-based novelty detection method works:
The idea is quite simple. An autoencoder can reconstruct the signals well with what it is trained with and for unseen or novel signals we will obtain higher reconstruction error. We used this reconstruction error parameter to differentiate known and novel signals. Here I showed an example. As you can see, the known IQ signals are reconstructed very well whereas, for the unseen signals, we obtained high reconstruction errors. This concept is used for novelty detection.
For novelty clustering, we use the DBSCAN method. And as parameters, we used the reconstruction error and bandwidth and dwell time, where the bandwidth and dwell time parameters were extracted by YOLO.
|
ICACT20230149 Slide.12
[Big slide for presentation]
|
Chrome Text-to-Speach Click!! |
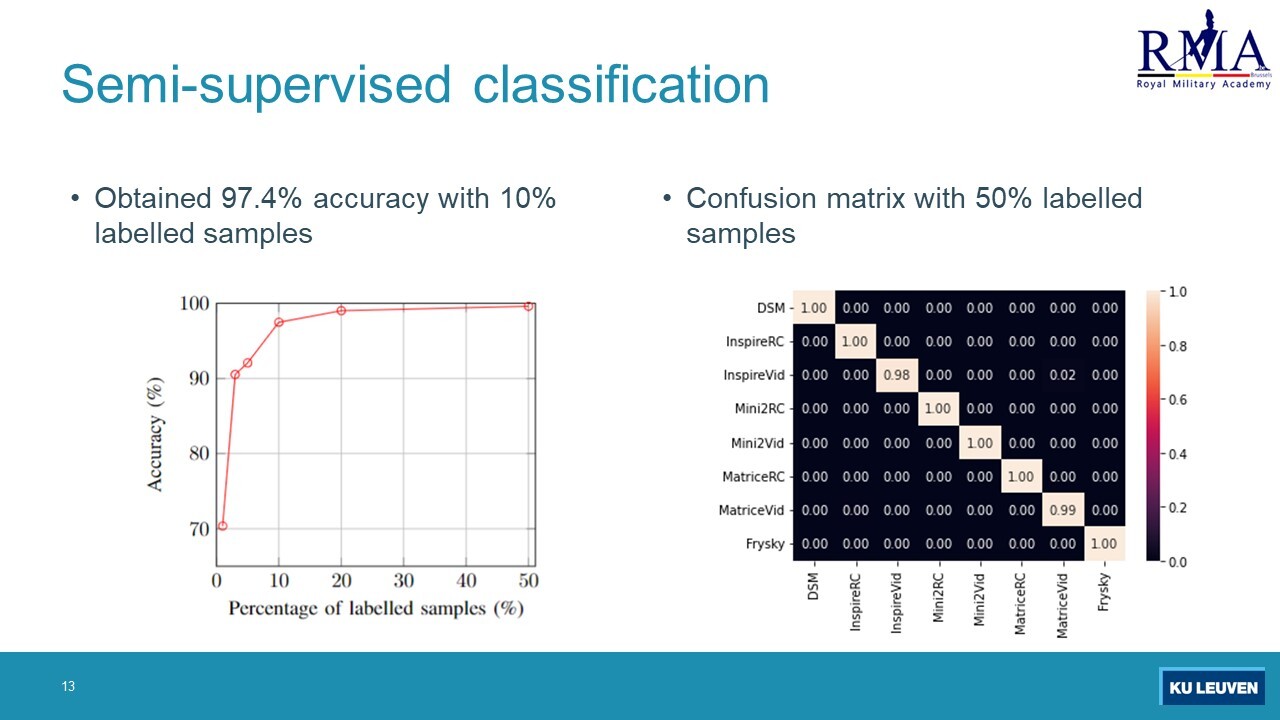 |
The classification was performed in a semi-supervised manner. The average classification accuracy for different numbers of labelled samples is presented in this figure. We obtained a 70.3% classification accuracy with only 1% labelled samples which corresponds to 34 labelled samples per class. With the increase in labelled samples, the average accuracy increases, and it reaches 97.4% with 10% labelled samples. The accuracy further increases and reaches 99.6% with 50% labelled samples.
The confusion matrix with 50% labelled samples is presented in this figure. As you can see we obtained a good classification performance.
|
ICACT20230149 Slide.11
[Big slide for presentation]
|
Chrome Text-to-Speach Click!! |
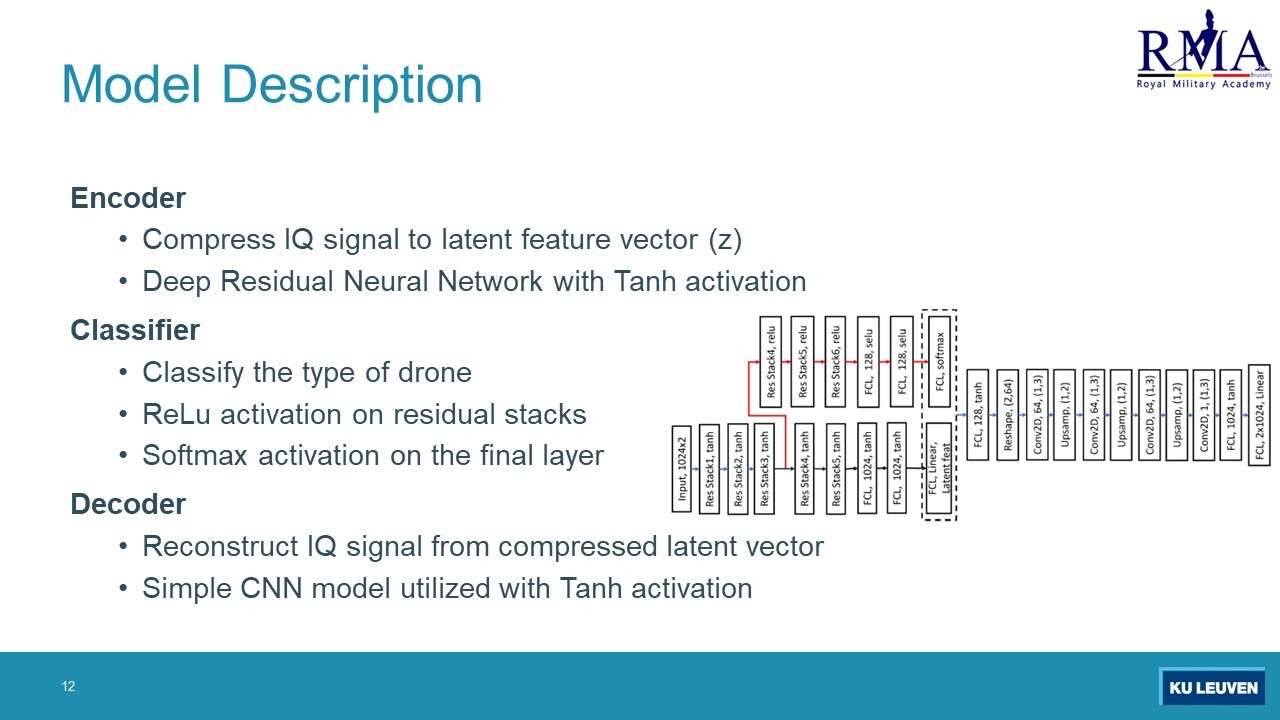 |
Our framework consists of an encoder, a classifier, and a decoder.
The encoder compresses the input IQ signal to a lower dimensional latent feature vector (z). The encoder consists of five residual stacks and three fully connected (FC) layers. We used Tanh activation in the encoder path.
The classifier classifies the type of drone. It shares some of the layers of the encoder. We added some residual stacks with RELU activation for the classification path which was followed by three fully connected layers. At the final layer, we used thesoftmax activation.
The decoder receives the concatenated vector from the encoder and classifier, and then it reconstructs the input signal. We have used a simple CNN architecture as the decoder. We utilized Tanh activation as we used for the encoder path.
|
ICACT20230149 Slide.10
[Big slide for presentation]
|
Chrome Text-to-Speach Click!! |
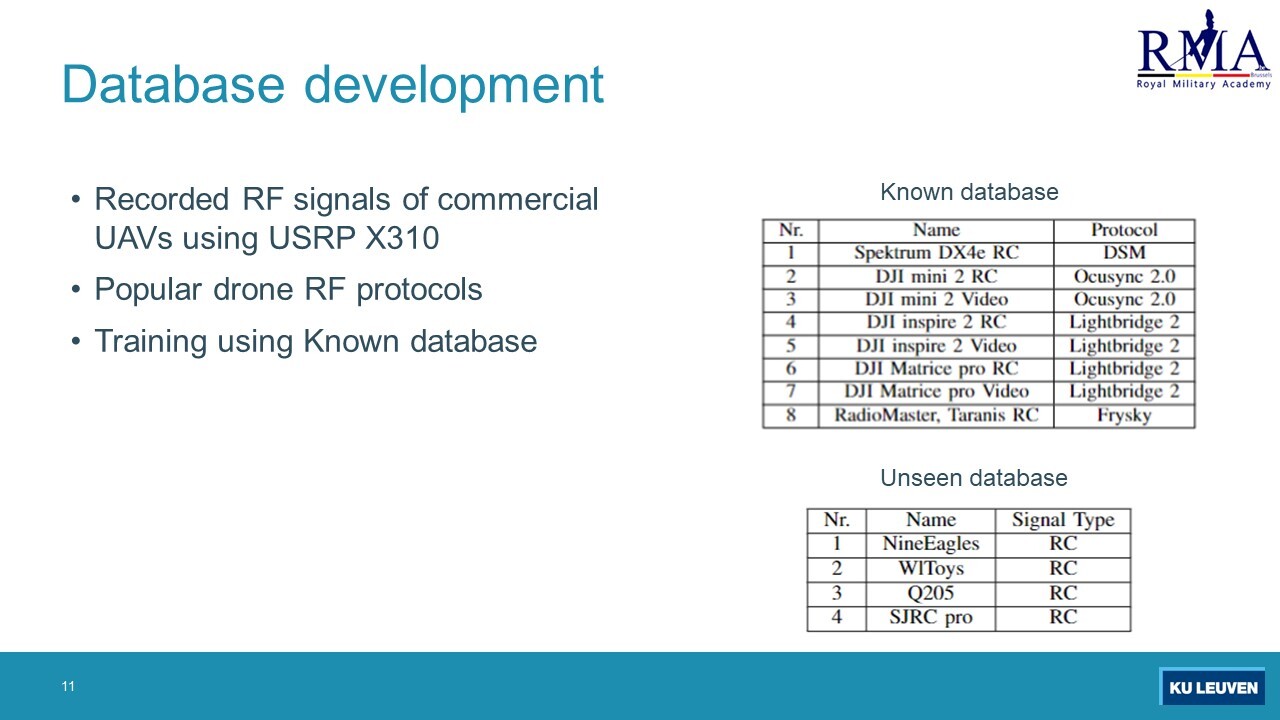 |
We developed our own RF database to evaluate the framework¡¯s performance. The RF signals were recorded in the semi-anechoic chamber of RMA. We used the USRP X310 to record the signals. We used all the drones available in our lab for this purpose. Among these drones, we used the signals with relatively popular RF protocols (e.g. DSM, Ocusync, Lightbridge) as the known signals. These we used to train our model. The rest of the drone signals were unseen to the model.
|
ICACT20230149 Slide.09
[Big slide for presentation]
|
Chrome Text-to-Speach Click!! |
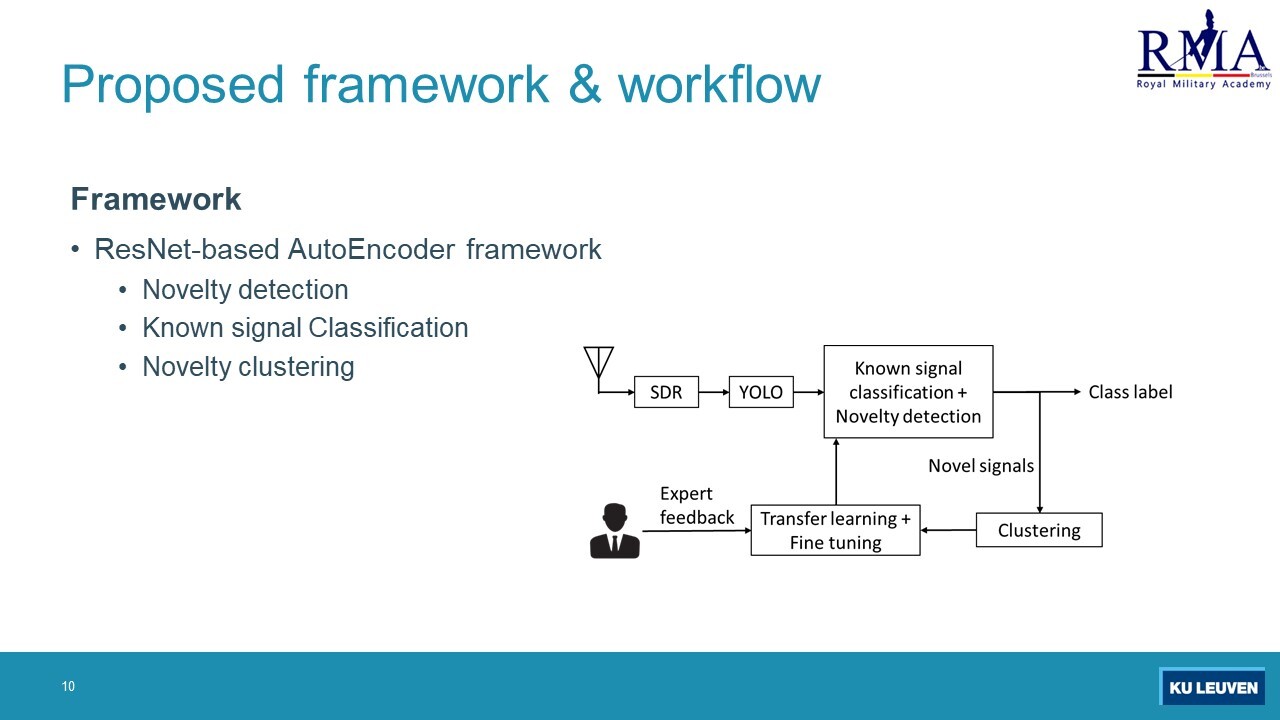 |
In this study, we proposed a ResNet-based autoencoder framework for Novelty detection, known signal classification, and Novelty clustering. Here we showed the workflow. After receiving a signal from an SDR, we utilize our YOLO framework for detecting the presence of RF signals. Afterward, we utilize our autoencoder framework. If the signal is known, the framework provides its class label. If unknown, we push it for clustering. After clustering, an expert can provide the class label and the model can relearn the novel signals.
|
ICACT20230149 Slide.08
[Big slide for presentation]
|
Chrome Text-to-Speach Click!! |
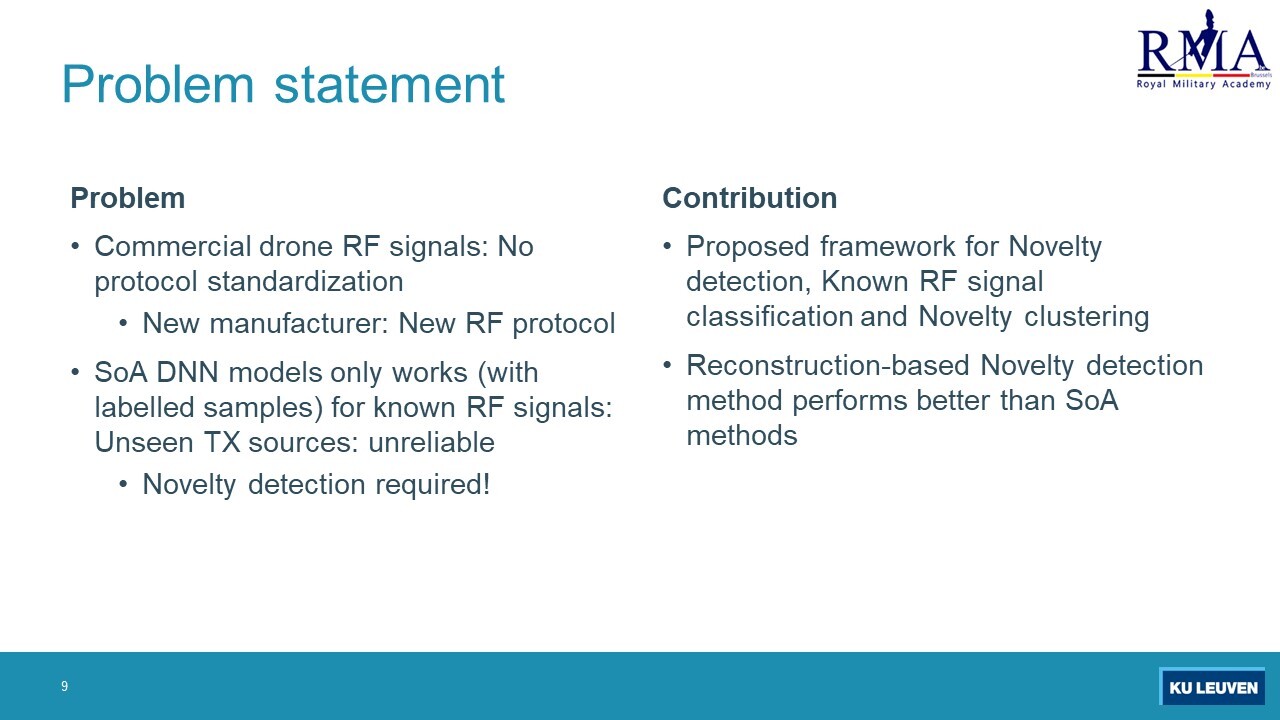 |
The commercial drone RF signals have no standardization for the RF protocol, which means that any manufacturer can create their own RF protocol and carry out the RF communication between the drone and the ground control station. State-of-the-art DNN-based classification can work with labelled samples, which means that it can only classify the signals it is trained with.
When you present a novel or an unknown signal, the classification performance becomes completely unreliable. Here, we need a novelty detection method before the classification.
Very limited literature addresses this RF novelty detection problem. In this study, we proposed a framework for novelty detection, known RF signal classification, and novelty clustering. We also show that the reconstruction-based novelty detection method outperforms the existing SoA methods.
|
ICACT20230149 Slide.07
[Big slide for presentation]
|
Chrome Text-to-Speach Click!! |
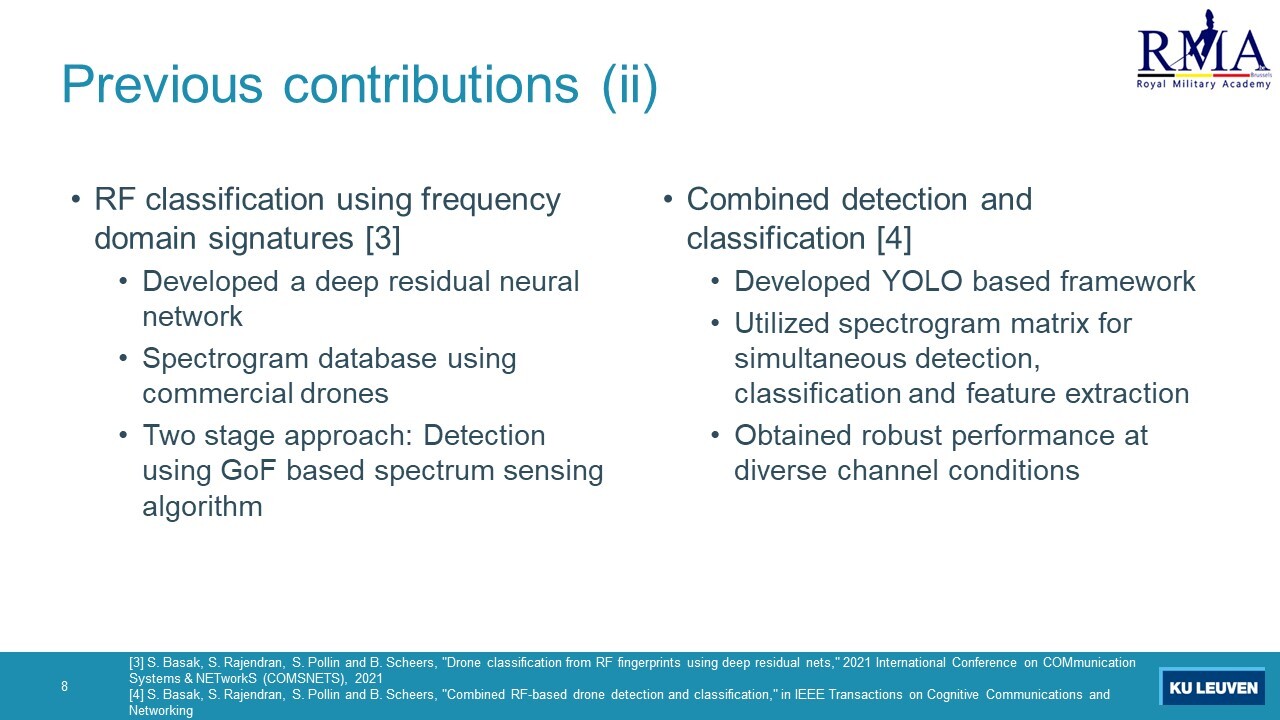 |
Then we developed an RF classification framework using a deep residual neural network. Here we utilized the frequency domain RF signatures. We built an RF spectrogram database. Here, we adopted a two-stage approach here: In the first stage, we performed RF detection using a goodness of fit-based spectrum sensing algorithm and then we performed classification using the deep residual neural network.
Later on, we developed a combined detection and classification framework using a "you only look once" (YOLO)-based framework. Here, we utilized the spectrogram matrix for simultaneous detection and classification. For both cases mentioned here, we obtained robust detection and classification performances at diverse channel conditions.
|
ICACT20230149 Slide.06
[Big slide for presentation]
|
Chrome Text-to-Speach Click!! |
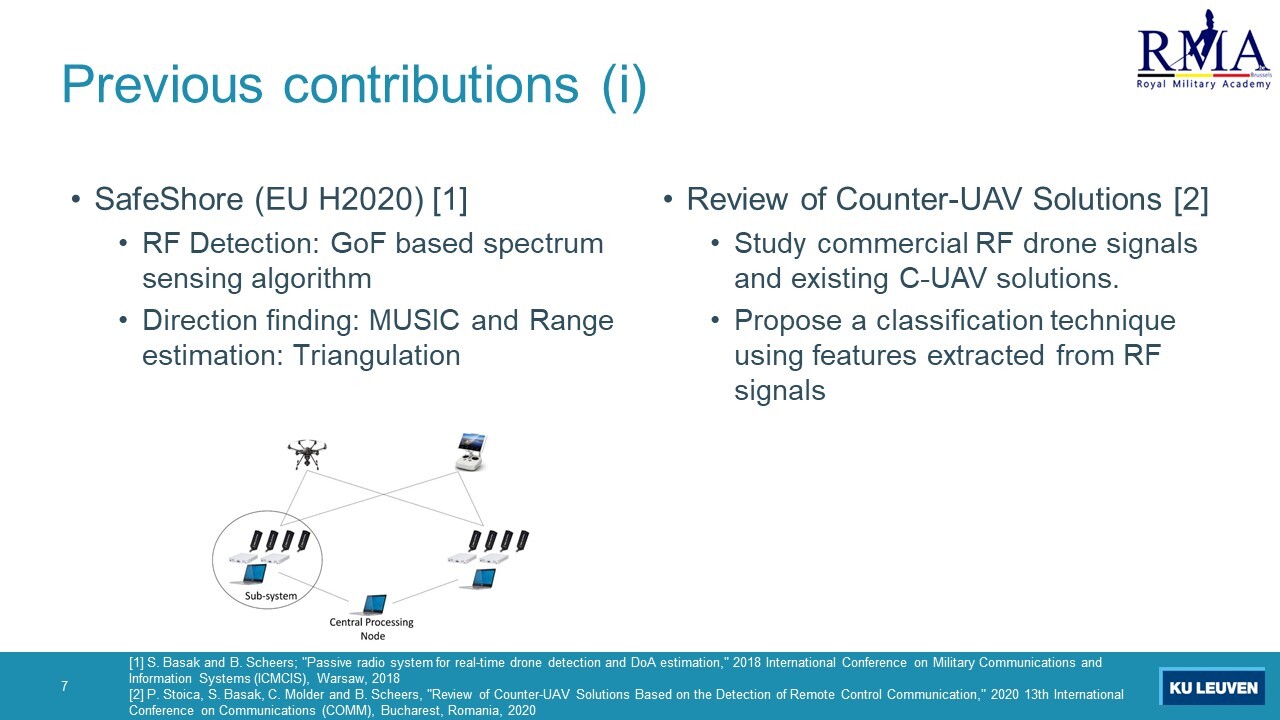 |
I will briefly talk about some of our previous works. Between 2016-18, we worked on an EU H2020 project called SafeShore, where we developed RF detection and localization system. The detection was performed using a goodness-of-fit based spectrum sensing algorithm. The direction of arrival estimation was performed using the MUSIC algorithm and the range estimation was performed using the triangulation method. Our system architecture is depicted in this figure.
Afterwards, we studied commercial drone RF signals and existing counter UAV solutions. There, we proposed a classification technique using the features extracted from drone RF signals.
|
ICACT20230149 Slide.05
[Big slide for presentation]
|
Chrome Text-to-Speach Click!! |
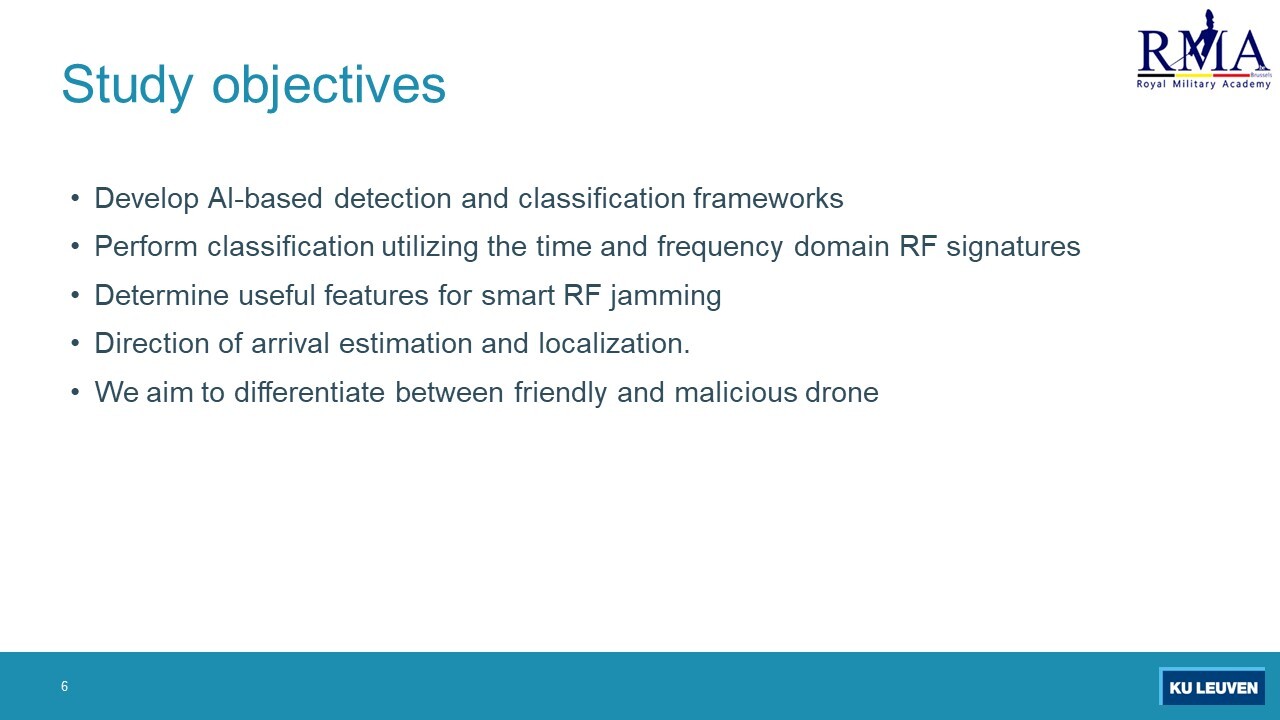 |
Now I will talk briefly about the objectives of my PhD. My study objectives include developing an artificial intelligence-based detection and classification framework. Mainly we utilize the time-frequency domain RF signatures for this purpose. We try to determine the useful RF features for RF jamming. We also develop our database for detection and classification. We also perform the direction of arrival estimation and localization of a drone. Furthermore, we also aim to differentiate between friendly and malicious drones from the RF signatures.
|
ICACT20230149 Slide.04
[Big slide for presentation]
|
Chrome Text-to-Speach Click!! |
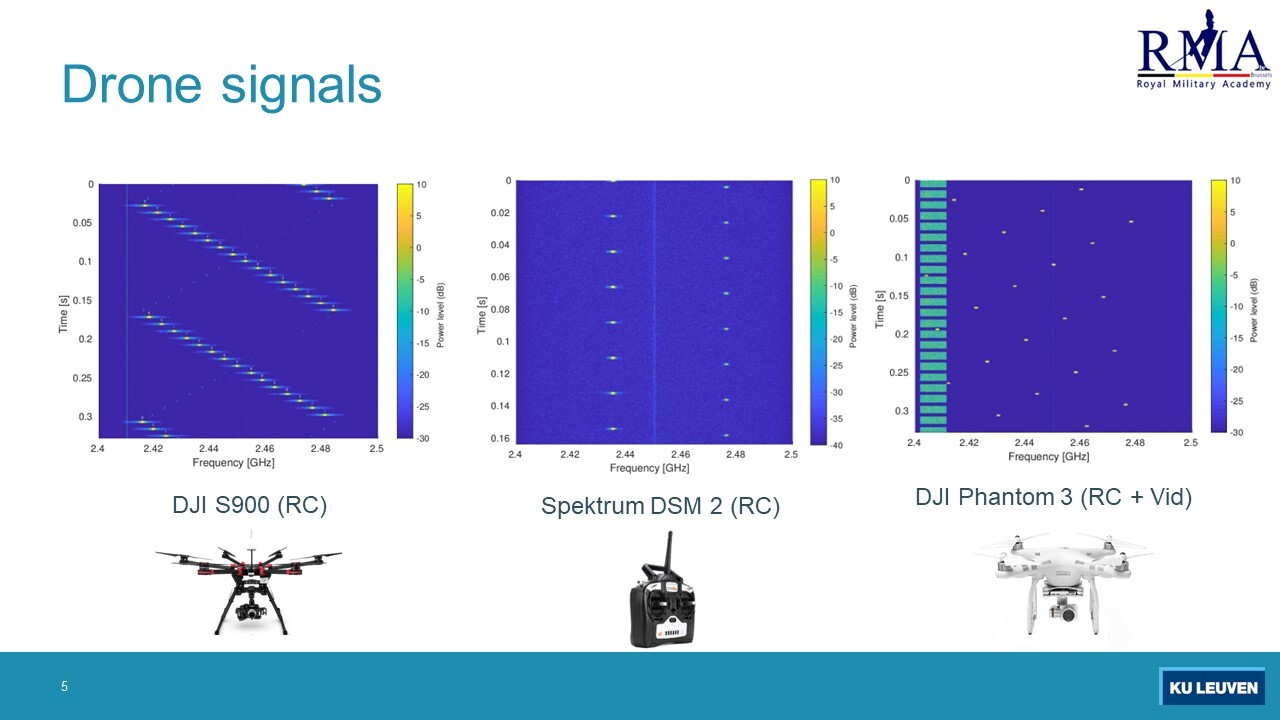 |
These are some examples of drone RF signals. Generally, the remote control signals or the command and control signals are frequency hopping spread spectrum. They hop between a range of frequencies for drones like DJI S900. For simple cases, it may also hop between two or three frequencies like you see for Spektrum DSM 2. This is a combined remote control and video RF signals for DJI phantom 3. Generally, the video RF signals are direct sequence spread spectrum or OFDM signal and as usual the hopping signal is the remote control signal.
|
ICACT20230149 Slide.03
[Big slide for presentation]
|
Chrome Text-to-Speach Click!! |
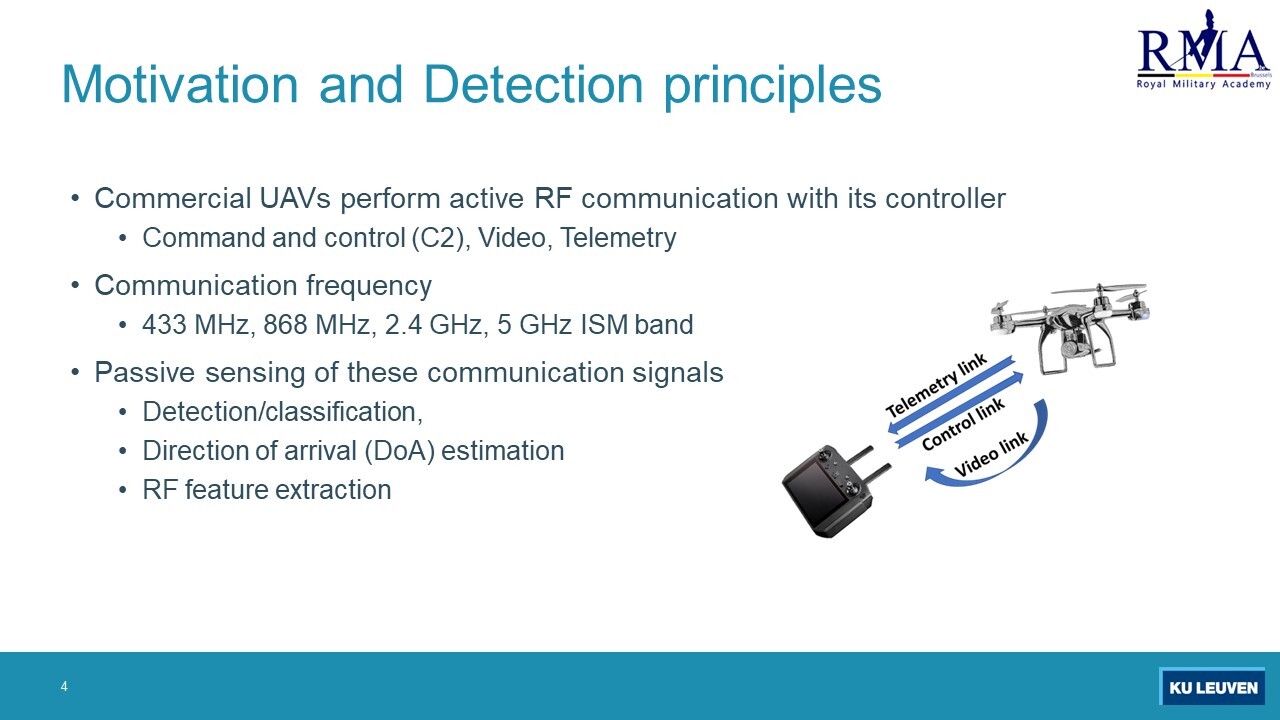 |
Commercial UAVs perform active RF communication with its remote controller or also known as ground control station. They use 3 communication links: command and control link, video link and telemetry link.
The communication is performed using these frequency bands: 433MHz, 868MHz, 2.4/5 GHz ISM band. Among these bands, 2.4 GHz and 5GHz ISM bands are very popular among the latest commercial drones.
The way RF detection works is: An RF detector listen to these communication links. With this it can perform detection and classification of a drone, direction of arrival estimation and useful RF feature extraction.
|
ICACT20230149 Slide.02
[Big slide for presentation]
|
Chrome Text-to-Speach Click!! |
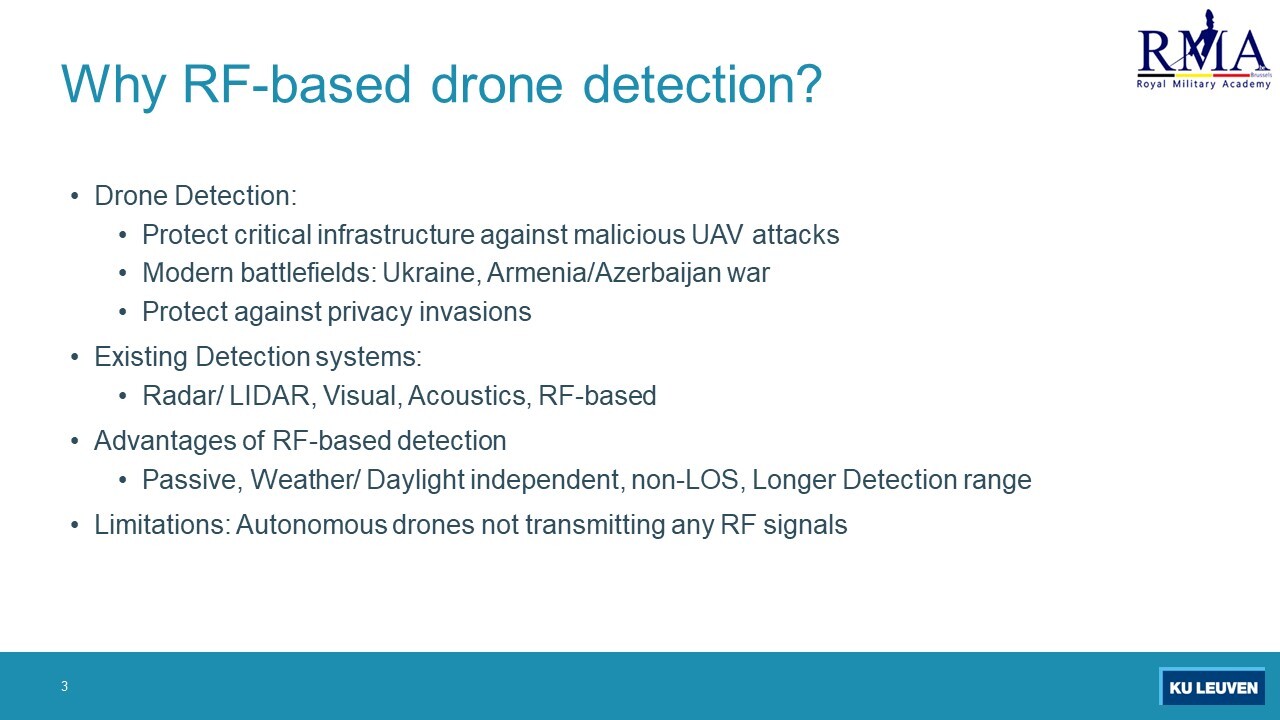 |
Why do we need a drone detection system? A drone detection is necessary to protect critical infrastructures against malicious UAV attacks. Now a days drones have become smaller and cheaper. Any terrorist organization can acquire a drone and can attack critical infrastructures like an airport, a military or a police base, an oil or a nuclear power station.
Modern battlefields such as the war in Ukraine or the Armenia/Azerbaijan war showed the importance of having a drone detection system. Furthermore, it also helps to protect against privacy invasions.
The existing drone detection systems include radar or lidar system, visual, acoustic system and an RF based system.
There are many benefits of an RF based detection systems over other detection systems. For example, it can work passively, it does not depend on daylight or weather conditions, it can work on non-line of sight conditions, and it has a longer detection range.
The only limitation is that if an autonomous drone does not transmit any RF signal, the detection will not work.
|
ICACT20230149 Slide.01
[Big slide for presentation]
|
Chrome Text-to-Speach Click!! |
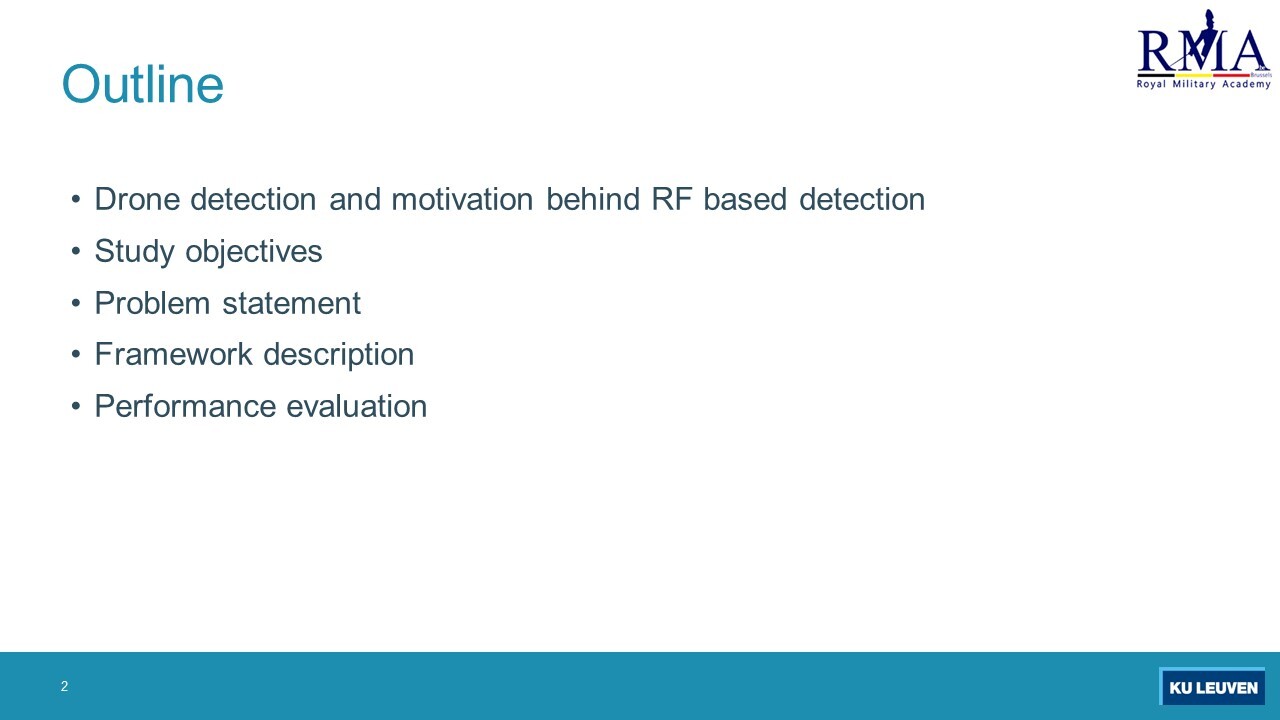 |
This is the outline of my today¡¯s talk. First, I am going to talk about the importance of drone detection and the motivation behind RF based drone detection. Then I will talk briefly about my study: what I work on and what my PhD is all about. Then I will talk about the problem statement: why do we need a RF novelty detection method. Afterwards I will describe our framework for RF signal classification, novelty detection and novelty clustering. Finally, I will show performance evaluation.
|
ICACT20230149 Slide.00
[Big slide for presentation]
[YouTube] |
Chrome Text-to-Speach Click!! |
 |
Hello everyone. My name is Sanjoy Basak. I am currently pursuing a joint Ph.D. at the Royal Military academy in association with KU Leuven. My mentor is Dr. Sreeraj Rajendran. My supervisors are Prof. Sofie Pollin and Prof. Bart Scheers. Today I am going to talk about an autoencoder-based framework that we developed for drone signal classification and novelty detection.
|