Now 50 visitors
Today:92 Yesterday:1040
Total: 27663
199S 93P 40R
2024-11-27, Week 48 |
Journal Homepage |
Call for Paper |
Author HomePage |
Paper Procedure |
Paper Submission |
Registration Invoice |
Welcome Message |
Statistics & History |
Committee |
Paper Archives |
Outstanding Papers |
Author HomePage |
- Paper Procedure |
- Journal Procedure |
- Presentation Tips |
|
Conference Proceedings |
Program with Papers |
Plenary Session |
Tutorial Session |
|
Presentation Assistant |
Hotel & Travel Info |
About Korea |
Accommodation |
Transportation |
VISA |
Other Infomation |
|
Photo Gallery |
FAQ |
Member Login |
Scheduler Login |
Seminar |
Archives Login |
|
Sponsors |
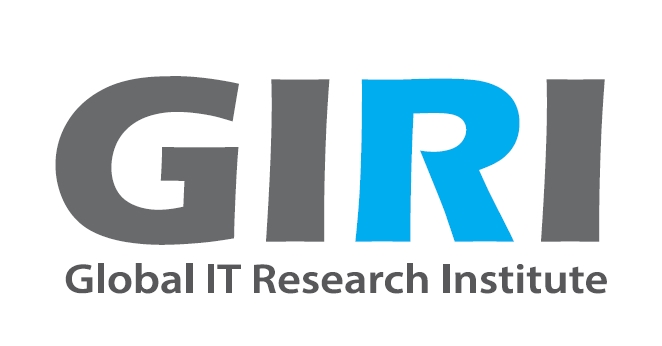
|
|
|
|
|
|
|
|
|
|
|
|
|
Save the slide and description |
|
* You can edit any slide by selecting the Slide # below, edit anything, and then 'Write' button (Save) |
ICACT20220314 Slide.22
[Big Slide]
[YouTube] |
Chrome Click!! |
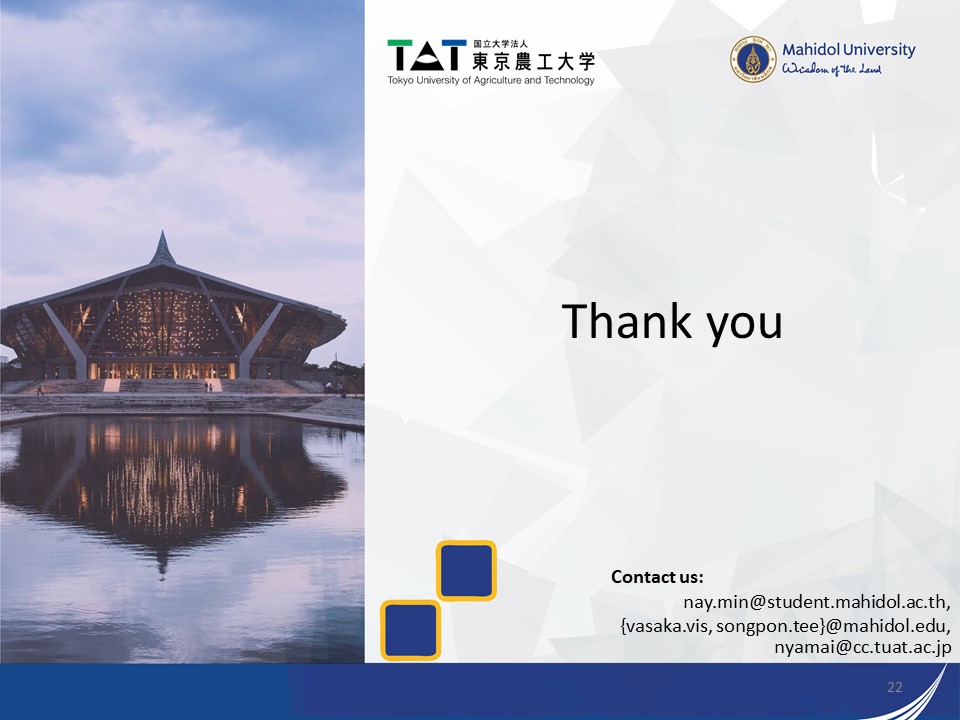 |
Thank you for listening. It was a pleasure presenting my paper.
|
ICACT20220314 Slide.21
[Big Slide]
[YouTube] |
Chrome Click!! |
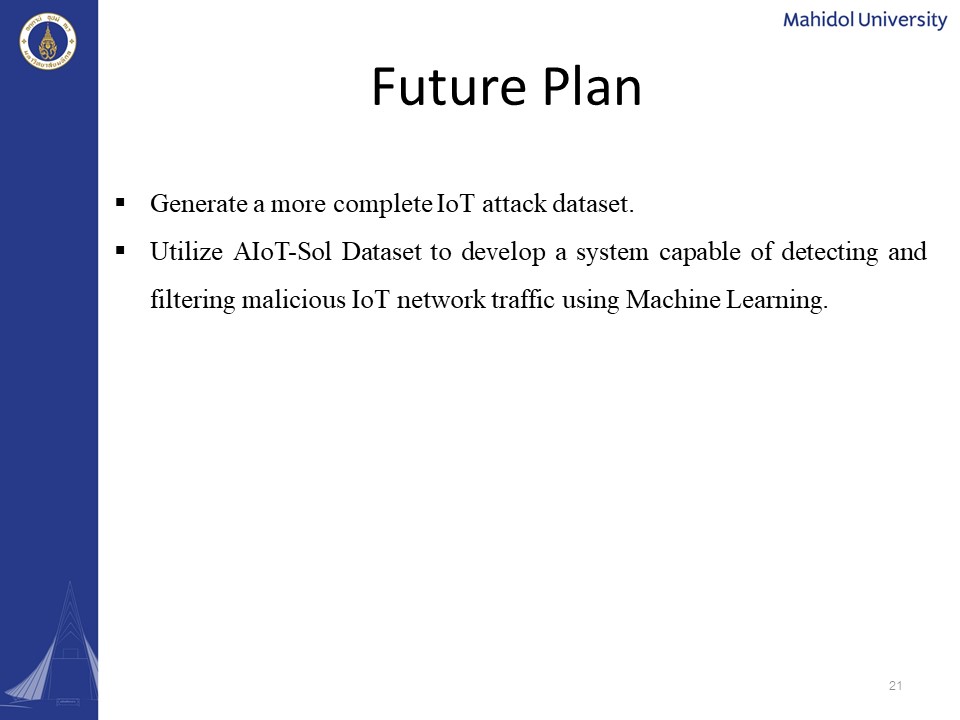 |
As for the future plan, we aim to generate a more complete IoT attack dataset. And also, we will be utilizing our AIoT-Sol attack dataset to develop a system capable of detecting and filtering malicious IoT network traffic using machine learning.
|
ICACT20220314 Slide.20
[Big Slide]
[YouTube] |
Chrome Click!! |
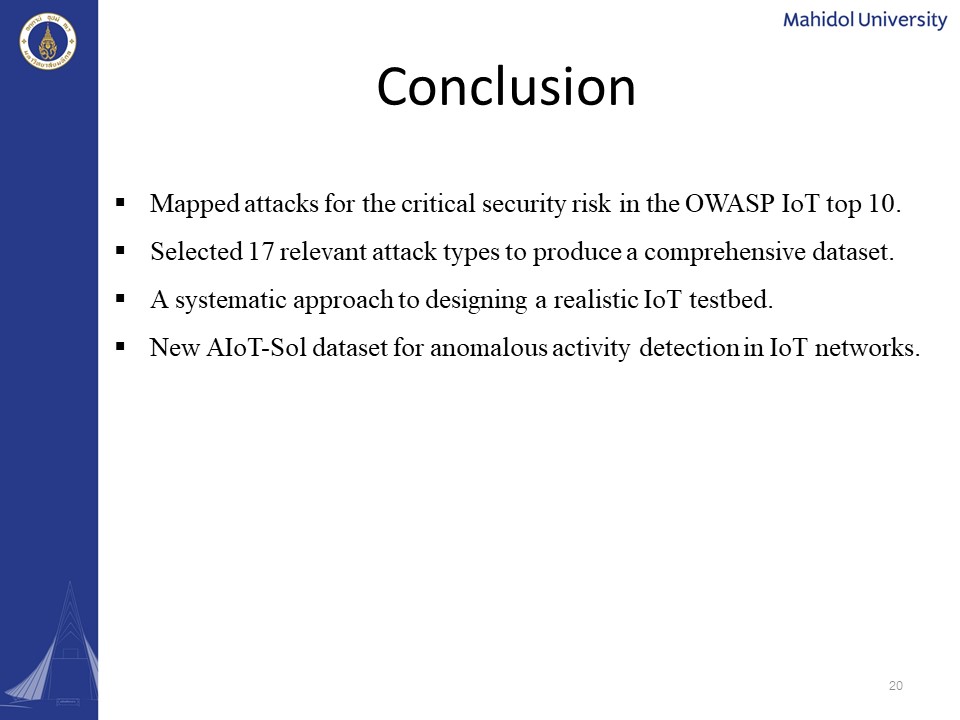 |
In conclusion, I would like to sum up the main points of our paper in our paper. We have mapped attacks for the critical security risk in the OWASP IoT top 10. We have also selected 17 relevant attack types to produce a comprehensive dataset. We have also shared a systematic approach to designing a realistic IoT testbed. Finally, we have created and shared a new AIoT-Sol dataset for anomalous activity detections in IoT networks.
|
ICACT20220314 Slide.19
[Big Slide]
[YouTube] |
Chrome Click!! |
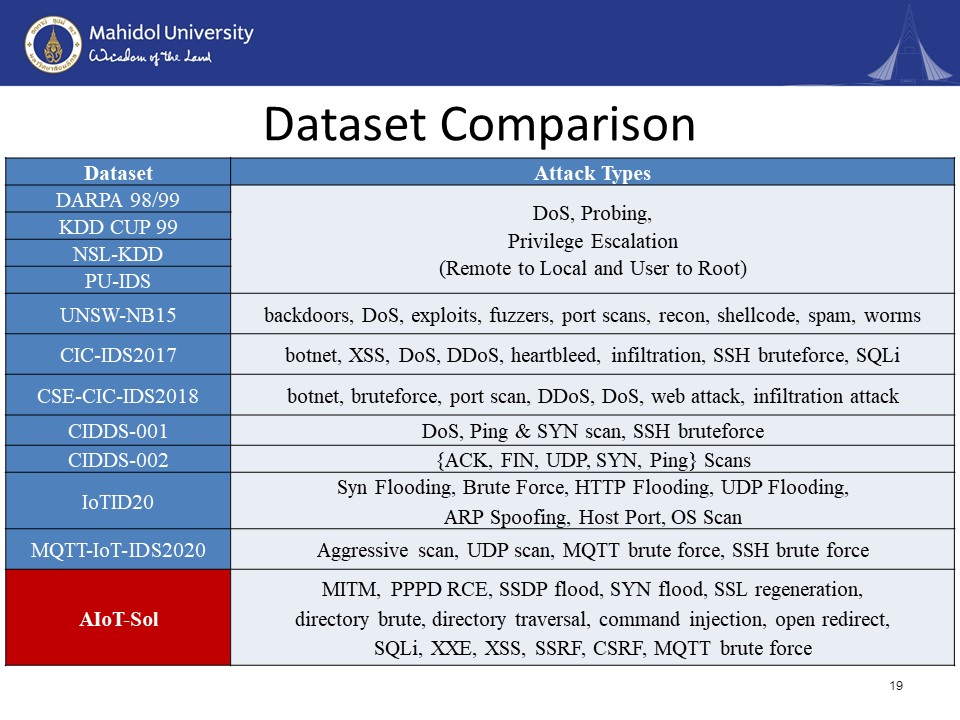 |
In this page, we will be presenting the comparison of our attack dataset named AIoT-Sol against the previous pre-existing attack sets. First of all, we have to compare with the datasets from the DARPA family. This family contains five attack datasets, which are the DARPA 98, DARPA 99, KDD cup 99, NSL KDD, and PUIDS. All of these DARPA family data sets contain the attack types such as DoS, Probing, Privilege Escalation from (Remote to Local and User to Root. Inside the UNSW-NB15, they contain backdoors, DoS, exploits, fuzzer, port scans, recon, shellcode, spam, worms. CIC-IDS2017 contain the botnet, XSS, DoS, DDoS, Heartbleed, infiltration, SSH bruteforce, SQL injection. The CSE-CIC-IDS2018 which is an improvement from the CIC-IDS2017, contained botnet, brute force, port scan, DDoS, DoS, web attack, infiltration attack. The CIDDS-001 and 002 contain two different types. The first one contains a Denial-of-service, Ping scan, SYN scan, and SSH brute-force. And 002 contains ACK Scan, FIN Scan, UDP Scan, SYN Scan, Ping Scan. IoTID20 includes the SYN Flooding, Brute Force, HTTP Flooding, UDP Flooding, ARP Spoofing, Host Port, OS Scan. Lastly, we have the MQTT-IoT-IDS2020. It contains Aggressive scan, UDP scan, MQTT brute force, SSH brute force. It can be seen from the comparison that our dataset contains more comprehensive attack types that actually reflect the real world. It has the MITM, PPPD RCE, SSDP flood, SYN flood, SSL regeneration, directory brute, directory traversal, command injection, open redirect, SQLi, XXE, XSS, SSRF, CSRF, MQTT brute force.
|
ICACT20220314 Slide.18
[Big Slide]
[YouTube] |
Chrome Click!! |
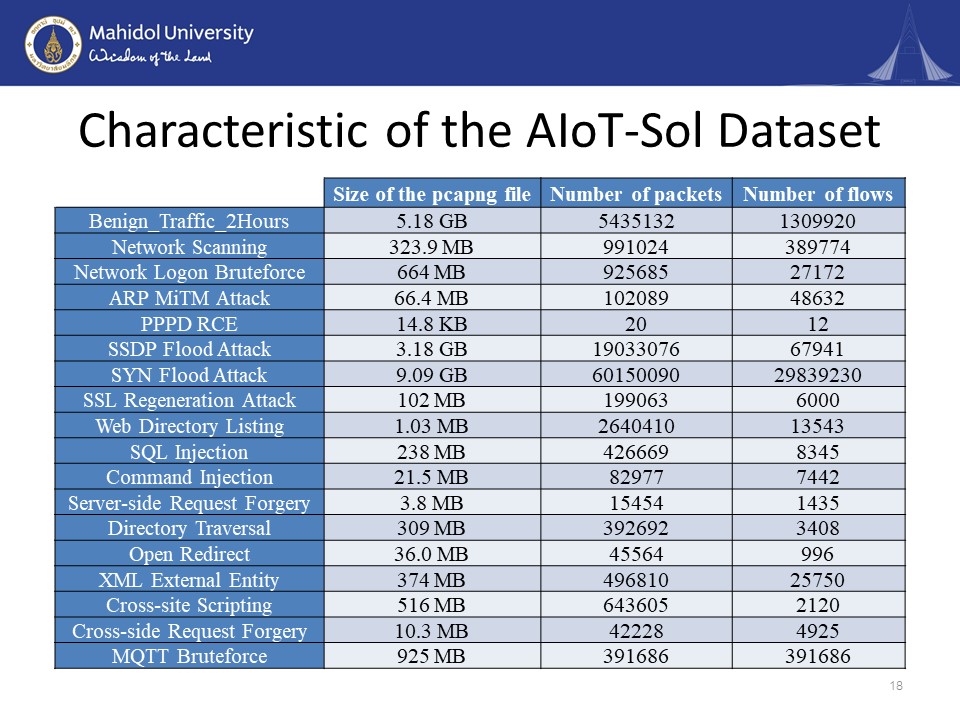 |
In this page, we have provided the characteristics of the AIoT-Sol attack dataset in terms of the size of the pcapng file, the number of packets, and the number of flows, for all of the attack types and the benign traffic. The benign traffic has been captured for two hours.
|
ICACT20220314 Slide.17
[Big Slide]
[YouTube] |
Chrome Click!! |
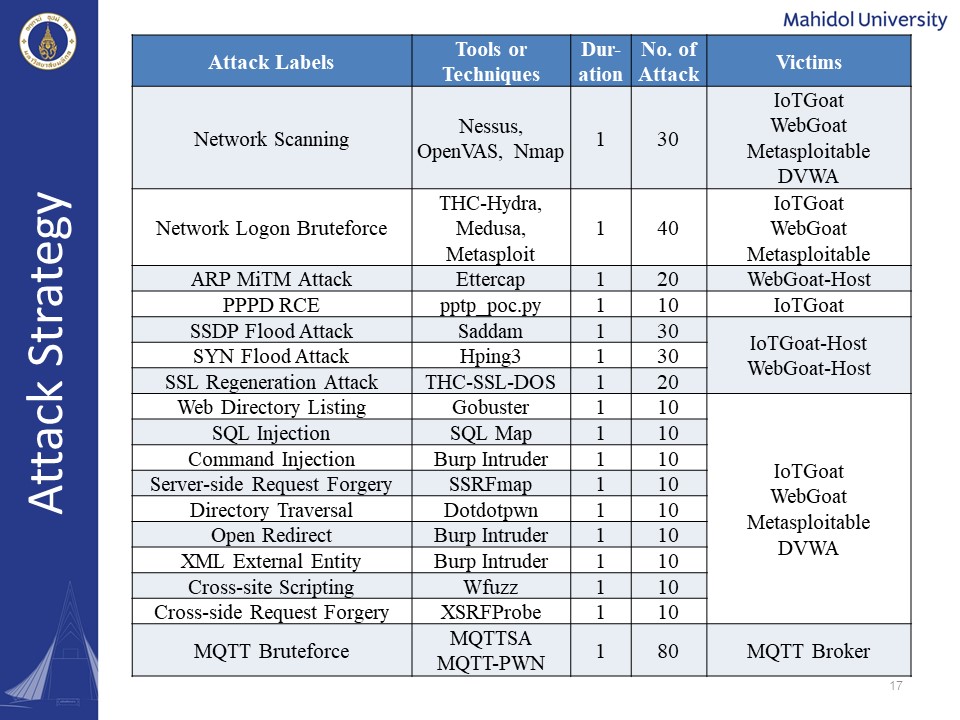 |
As for the attack strategy we simulated all of the 17 attack types for one-minute par packet capture each, using the attacking tools and techniques we have listed here. In order to have a realistic target, we have used the IoT devices and the deliberately vulnerable machines, and MQTT brokers as a victim machines.
|
ICACT20220314 Slide.16
[Big Slide]
[YouTube] |
Chrome Click!! |
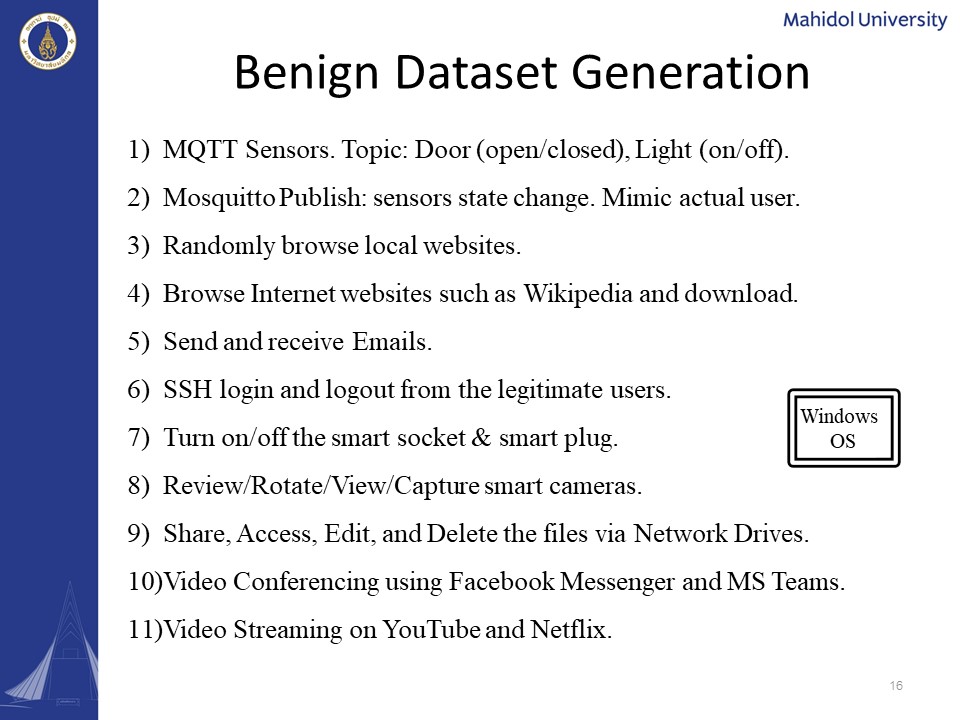 |
I would like to introduce you to how we generated the benign dataset. First of all, we included the MQTT sensors with two topics which are open and closed for the door and the on and off for the light. We use MQTT publish for the control of the sensor state change in order to actually mimic the actual users. And thirdly, we randomly browse local websites. Together with browsing the internet websites such as Wikipedia. And we also try simulating the downloading. We have sent and received emails using dummy accounts. And also, we tried ssh login and logout from the legitimate users. We also turned on/off the smart socket and the smart plug. We reviewed, rotate, view, capture the smart cameras. And as for the network drives share, we use it for sharing, accessing, editing, and deleting files. In number 10, we tried video conferencing using Facebook messenger and the Microsoft MS teams, and also, we streamed videos from YouTube and Netflix. All of these were recorded using the windows operating system.
|
ICACT20220314 Slide.15
[Big Slide]
[YouTube] |
Chrome Click!! |
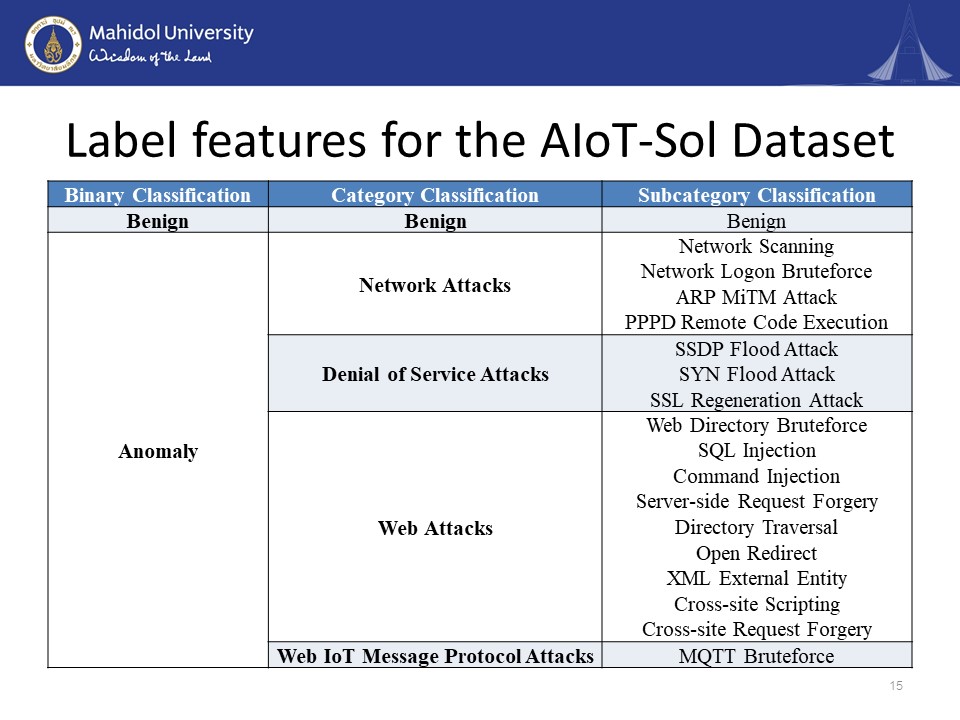 |
The label features of the dataset are three-fold. It can be binary, category, and subcategory. Binary contains benign and anomaly only. In the category, we have benign and other four major attack categories, which are network attacks, denial-of-service attacks, web attacks, and web IoT message protocol attacks. Inside the subcategory, we will have all of the 17 types of attacks we have selected. Of course, it will also have the binary.
|
ICACT20220314 Slide.14
[Big Slide]
[YouTube] |
Chrome Click!! |
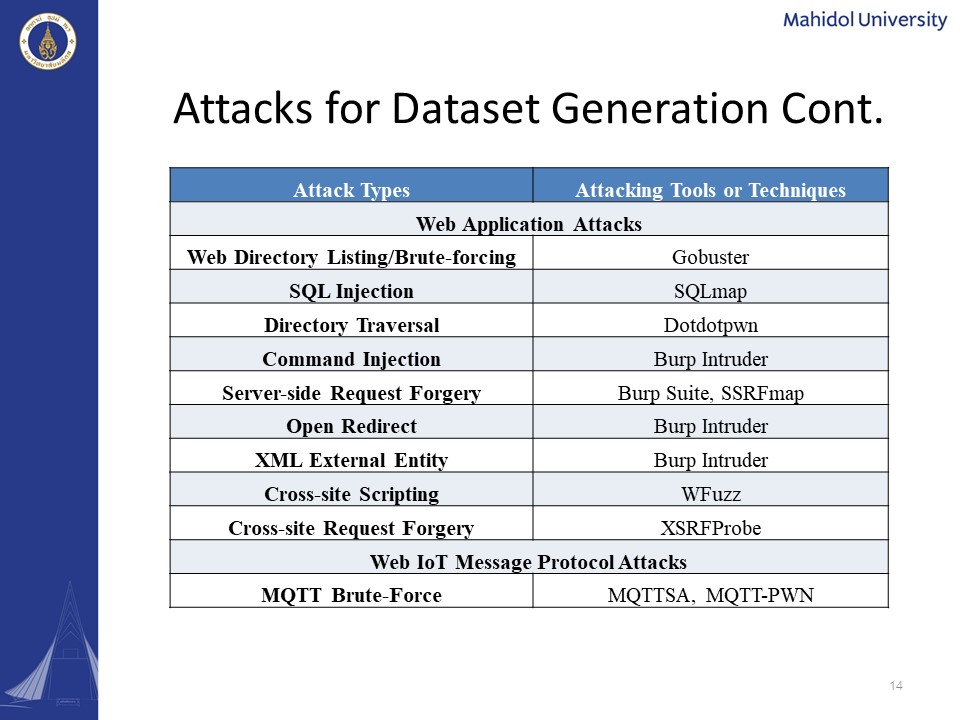 |
As for the web application attacks, we included the web directory brute-forcing, SQL injection, directory traversal, command injection, server-side request forgery, open redirect, XML external entity, cross-site scripting, and the cross-site request forgery. In order to generate the web application attacks, we had to use several different attacking tools such as Gobuster, sqlmap, dotdotpwn, burp intruder, Burp Suite, SSRFmap, burp intruder again, and the wfuzz and xsrf probing. And for the generation of the mqtt brute force, which is the inside the category of Web IoT message protocol attacks, we had to use mqttsa and mqttpwn attacking tools.
|
ICACT20220314 Slide.13
[Big Slide]
[YouTube] |
Chrome Click!! |
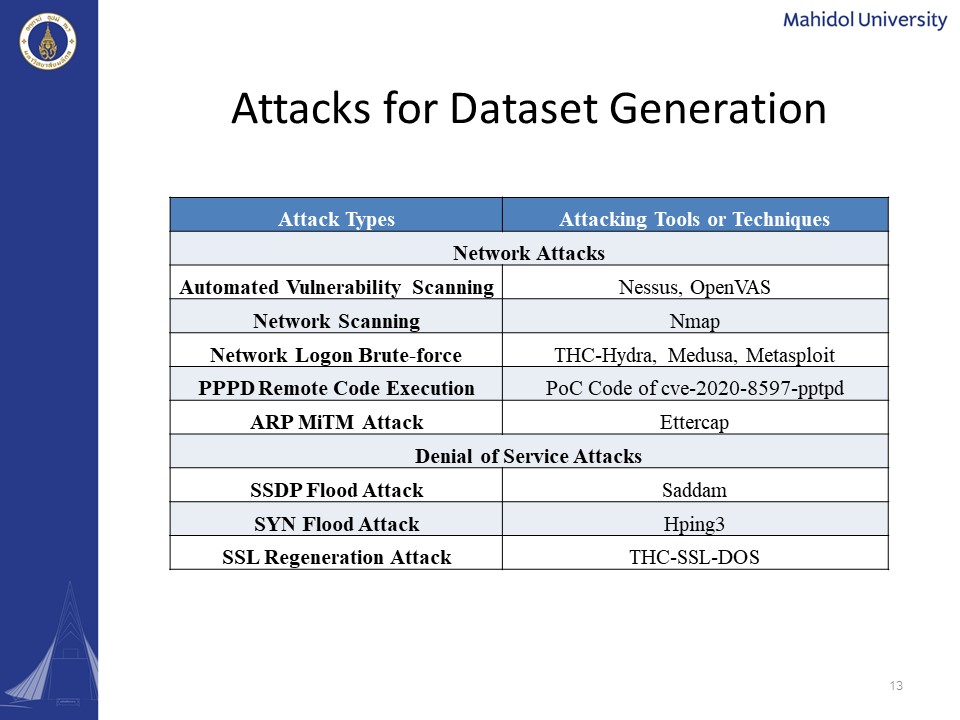 |
The automated vulnerability scanning, network scanning, network logon brute force, PPPD remote code execution, and man-in-the-middle attack for the network attacks category. And SSDP flood attack, SYN flood attack, SSL Regeneration attack, for the denial-of-service attack category. In order to generate the network attack category, we use the attacking tools such as Nessus, OpenVAS, Nmap, Hydra, Medusa, Metasploit, and Ettercap. As for the generation of the PPPD remote execution, we have downloaded a POC-code from the cve-2020-8597. And similarly, we have used the Saddam, Hping3, thc-ssl-dos for the generation of denial-of-service attacks.
|
ICACT20220314 Slide.12
[Big Slide]
[YouTube] |
Chrome Click!! |
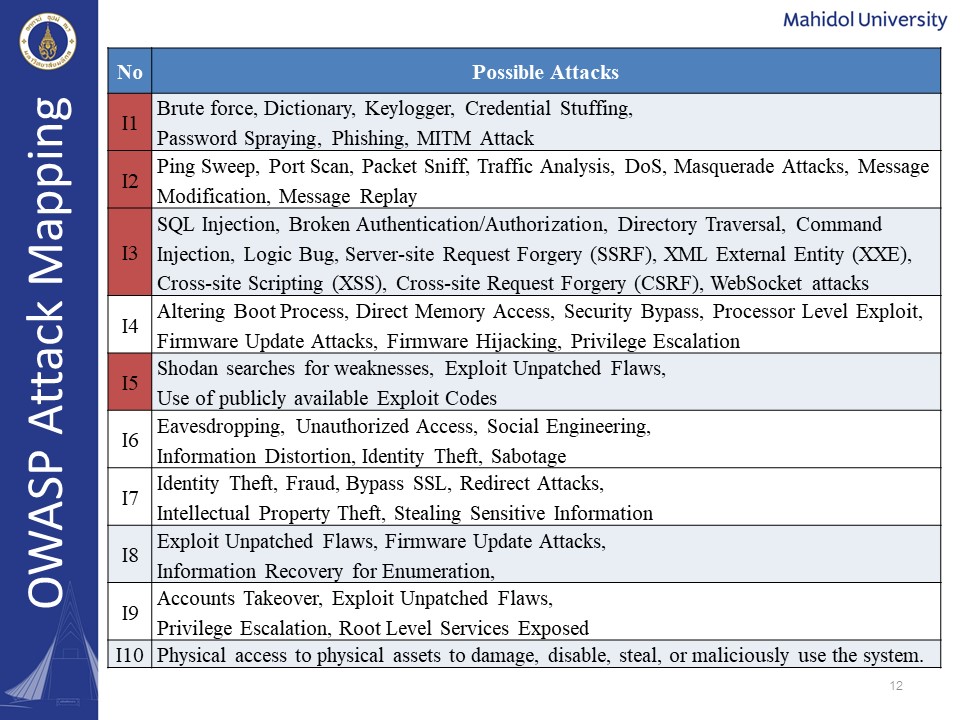 |
The OWASP IoT top 10 attack types mapping can be seen as follows: We had come up with several possible attack types for each critical security risk. In our dataset, we selected attacks from the I1, I2, I3, and I5. These attacks are:
|
ICACT20220314 Slide.11
[Big Slide]
[YouTube] |
Chrome Click!! |
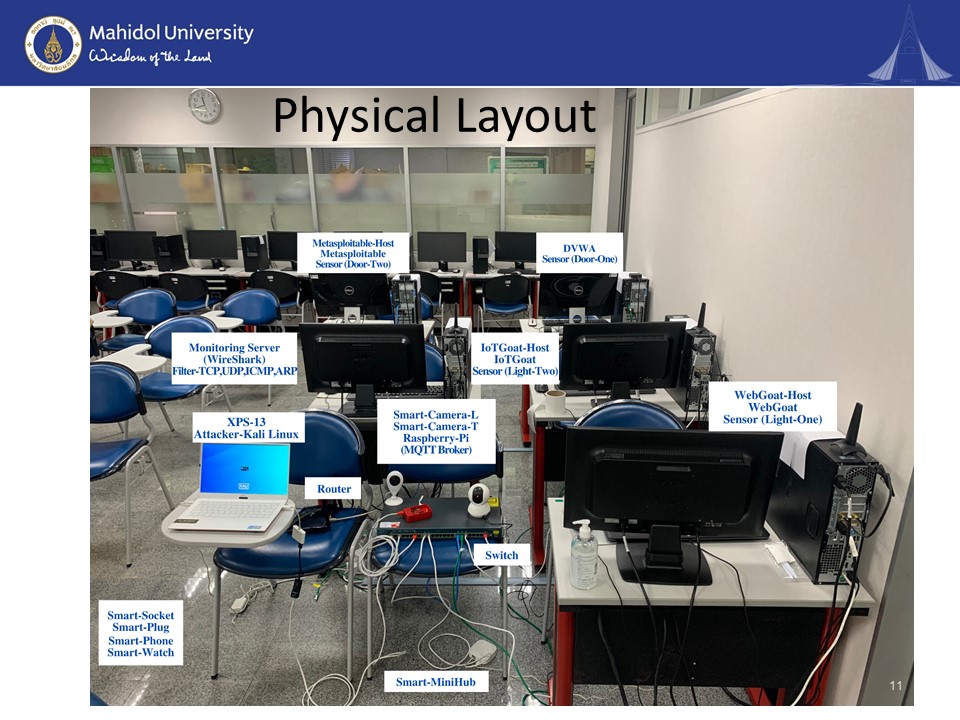 |
in the following picture. We have used the TCP, UDP, ICMP, and ARP filters for the Wireshark packet captures. We have a router for the wireless connection towards the IoT devices. The IoT devices are a smart socket, plug, phone, watch, and cameras, and the mini-hub. And we have used raspberry-pi for the simulation of the MQTT broker and we have the end-user devices and in these end-user devices, we have installed a deliberately vulnerable machine. And also, we have installed the sensors such as sensors for the door and the sensor for the light. You can see here that we have one attacking machine which is using the Kali Linux operating system in order to launch different attacks.
|
ICACT20220314 Slide.10
[Big Slide]
[YouTube] |
Chrome Click!! |
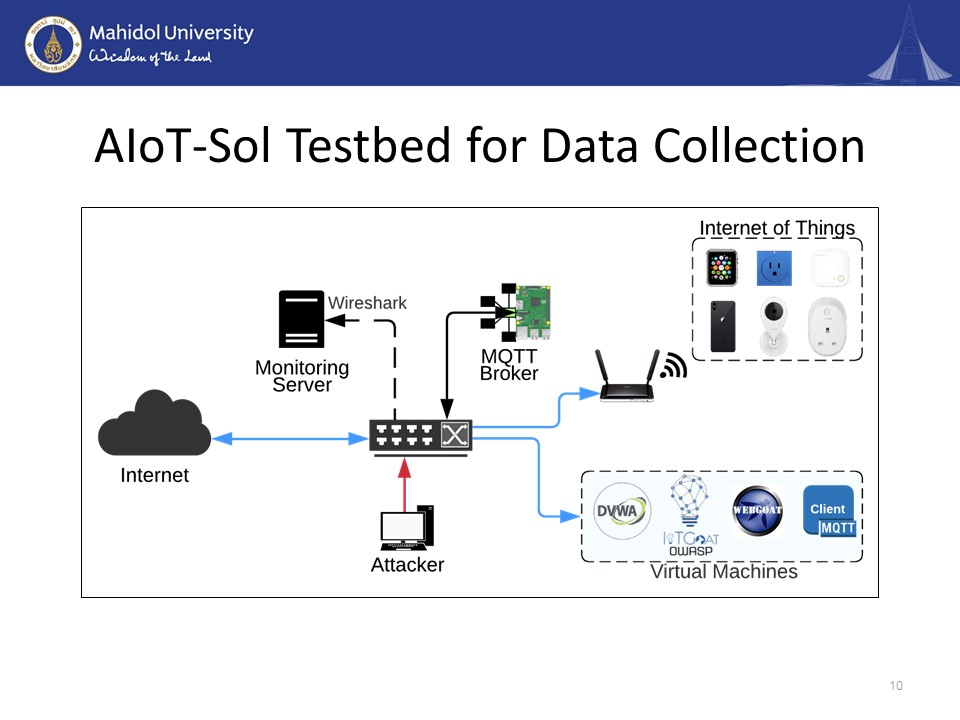 |
Since we have finished giving some of the background knowledge, I would now like to introduce you to our AIoT-Sol testbed architecture. We emulated the testbed in an internet-enabled environment. We have a central switch where all of the devices in the network are connected. The included IoT devices are the smartwatch, smartphone smart plug, smart cameras, and virtualized deliberately vulnerable machines. It also contains the MQTT broker and four MQTT sensors. We have one attacker machine inside the testbed from where we generate all of the attacks. We install Wireshark on one of the computers and transform it into the monitoring server. The physical layout can be seen
|
ICACT20220314 Slide.09
[Big Slide]
[YouTube] |
Chrome Click!! |
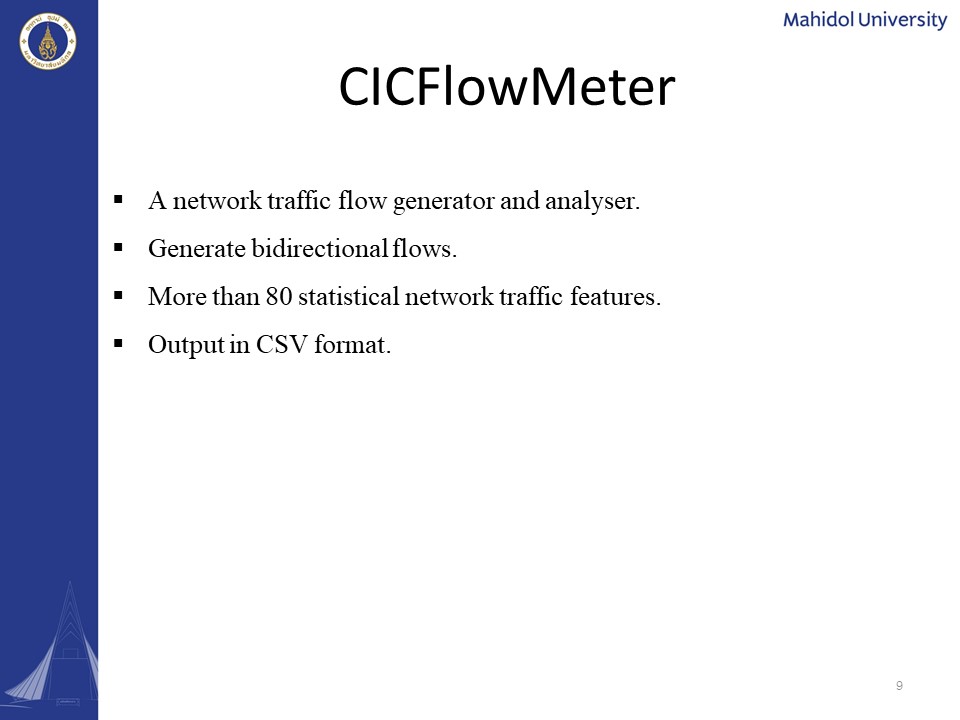 |
Let me also briefly explain about a network traffic flow generator analyzer we have utilized for our experiment. It is called a CIC Flowmeter and it is used to generate bi-directional flows. More than 80 statistical features were extracted using this tool. Since its outputs in the csv format, we can readily use the extracted dataset in the machine learning training.
|
ICACT20220314 Slide.08
[Big Slide]
[YouTube] |
Chrome Click!! |
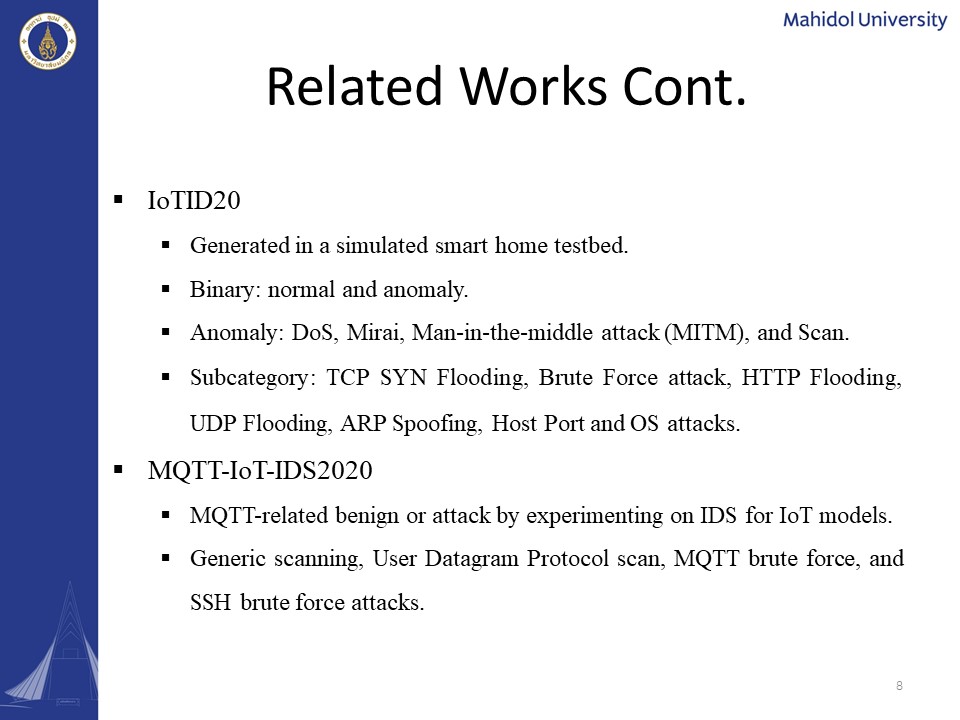 |
Lastly, in the IoTID20, which has been generated in a simulated smart home testbed, it includes the binary, category, and subcategory. Binary includes normal, and anomaly. In the anomaly it has a DoS, Mirai, Man-in-the-middle attack, and the scanning. In the subcategory, it has TCP SYN Flooding, Brute Force attack, HTTP Flooding, UDP Flooding, ARP Spoofing, Host Port and OS attacks. In the MQTT-IoT-IDS2020, it includes the MQTT related benign or attack by experimenting on the IDS for IoT models. It includes Generic scanning, User Datagram Protocol scan, MQTT brute force, and SSH brute force attacks.
|
ICACT20220314 Slide.07
[Big Slide]
[YouTube] |
Chrome Click!! |
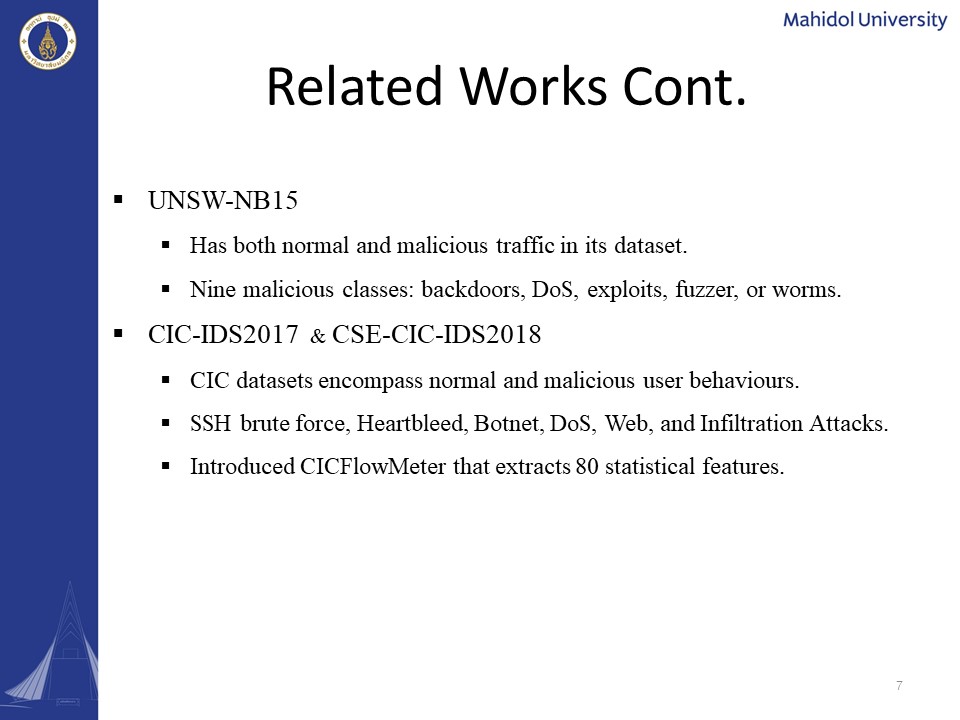 |
In UNSW-NB15, it has both normal and malicious traffic in its dataset. The nine malicious classes are included backdoors, DDoS, fuzzer, and worms. In the CIC-IDS-2017 and the 2018, they have normal and malicious user behavior. SSH brute force, Heartbleed, Botnet, DoS, Web, and Infiltration Attacks. They also introduced the CIC Flowmeter which can extract 80 statistical features.
|
ICACT20220314 Slide.06
[Big Slide]
[YouTube] |
Chrome Click!! |
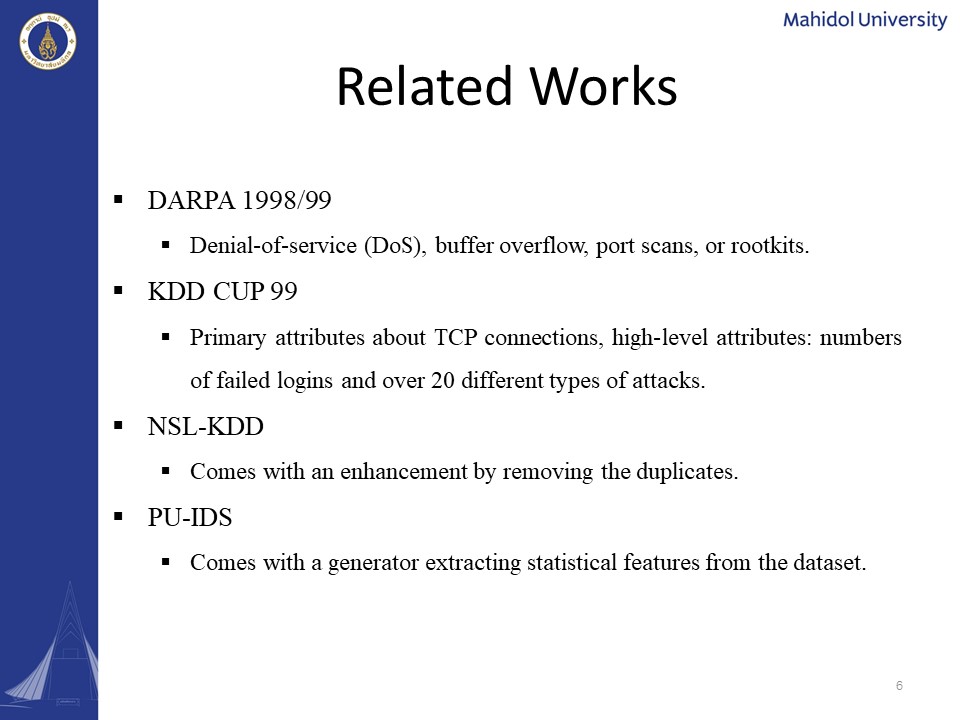 |
Allow me to introduce you to some of the several related works. DARPA 98 and 99 included the denial-of-service dos buffer overflow port scans or rootkits. In KDD cup 99, they included the primary attributes about TCP connections and several high-level attributes such as numbers of field logins and over 20 different types of attacks. In the NSL KDD, they try to come up with the enhancement by removing the duplicates from the KDD cup 99. In PU-IDS, it comes with a generator extracting statistical features from the dataset.
|
ICACT20220314 Slide.05
[Big Slide]
[YouTube] |
Chrome Click!! |
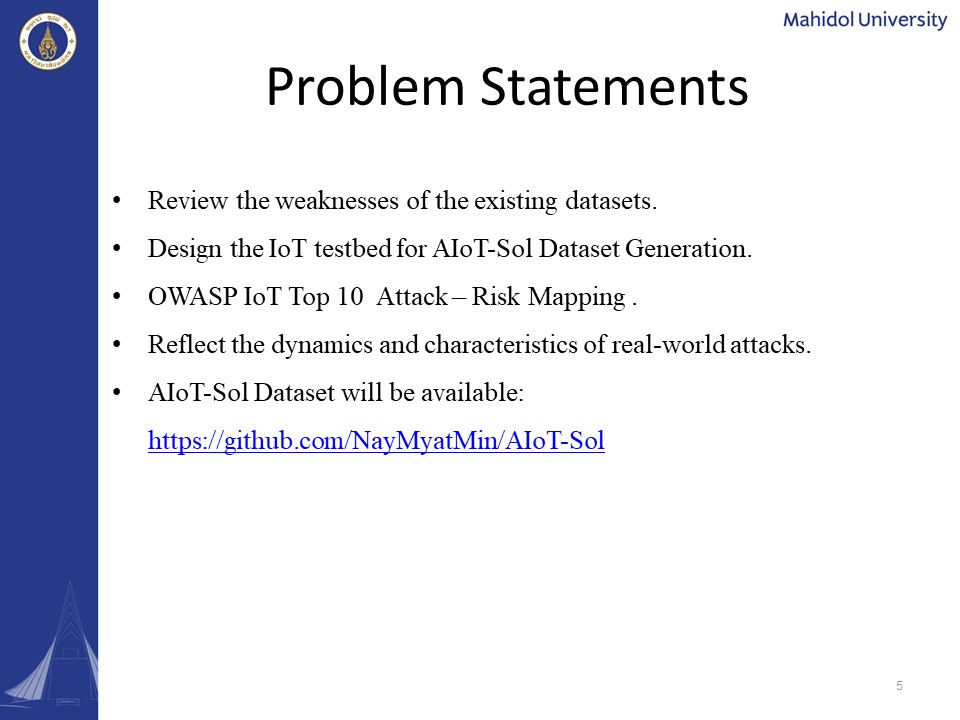 |
Next, we will talk about the problem statement. Firstly, we reviewed the weaknesses in various intrusion detection datasets. Secondly, we designed the IoT testbed for AIoT-Sol Dataset Generation. Thirdly, we provided a ¡°attack and risk¡± mapping based on the OWASP IoT Top 10 Attacks. And also, we tried to encompass the dynamics and characteristics of real-world attacks. The dataset will be available at the given link below.
|
ICACT20220314 Slide.04
[Big Slide]
[YouTube] |
Chrome Click!! |
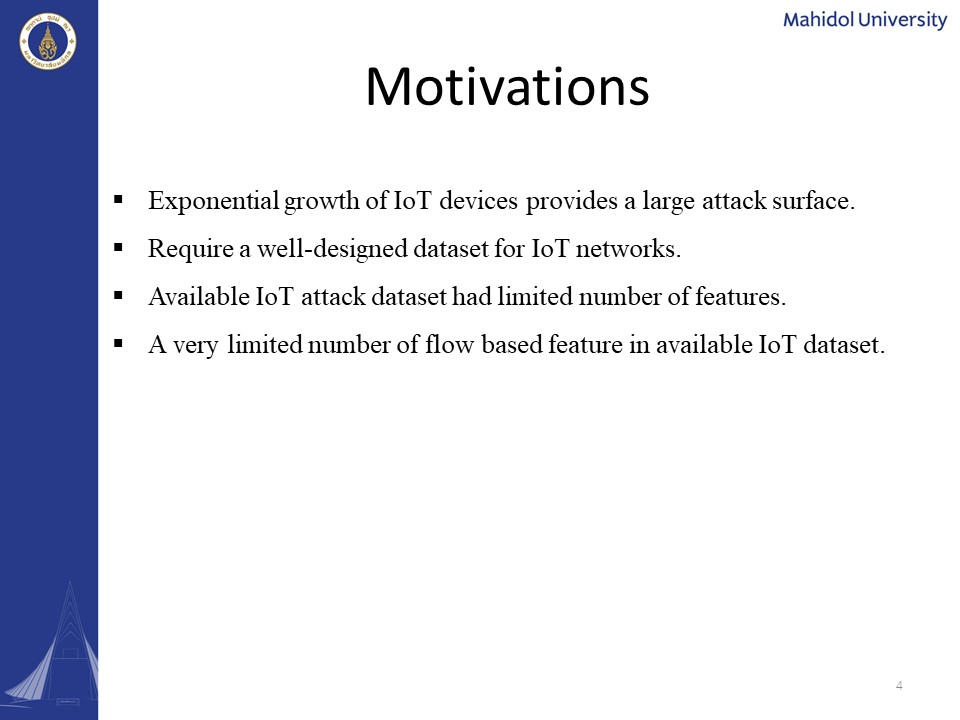 |
Let me continue wit the motivation. The exponential growth of IoT provides a large attack surface for intruders to launch more destructive cyber-attacks. New techniques and detection algorithms require a well-designed dataset for IoT networks. However, the available IoT intrusion datasets had a limited number of features. And also, a very limited number of flow-based features is available in the existing dataset.
|
ICACT20220314 Slide.03
[Big Slide]
[YouTube] |
Chrome Click!! |
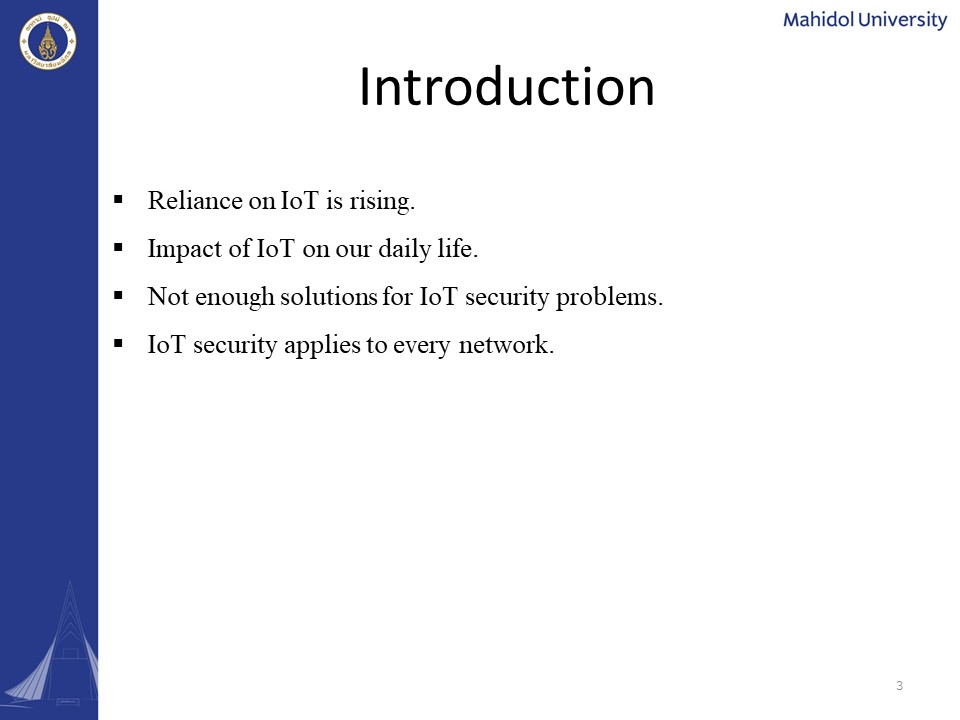 |
I would like to give you some background information about IoT ¡°the Internet of things¡±. With the latest technological advancements, Reliance on IoT has become very popular. Therefore, we cannot underestimate the impact of IoT on our daily life anymore. We also do not have enough solutions to match the growth in IoT security problems. IoT security actually applies to every network and most especially critical for the enterprises with many IoT system deployments.
|
ICACT20220314 Slide.02
[Big Slide]
[YouTube] |
Chrome Click!! |
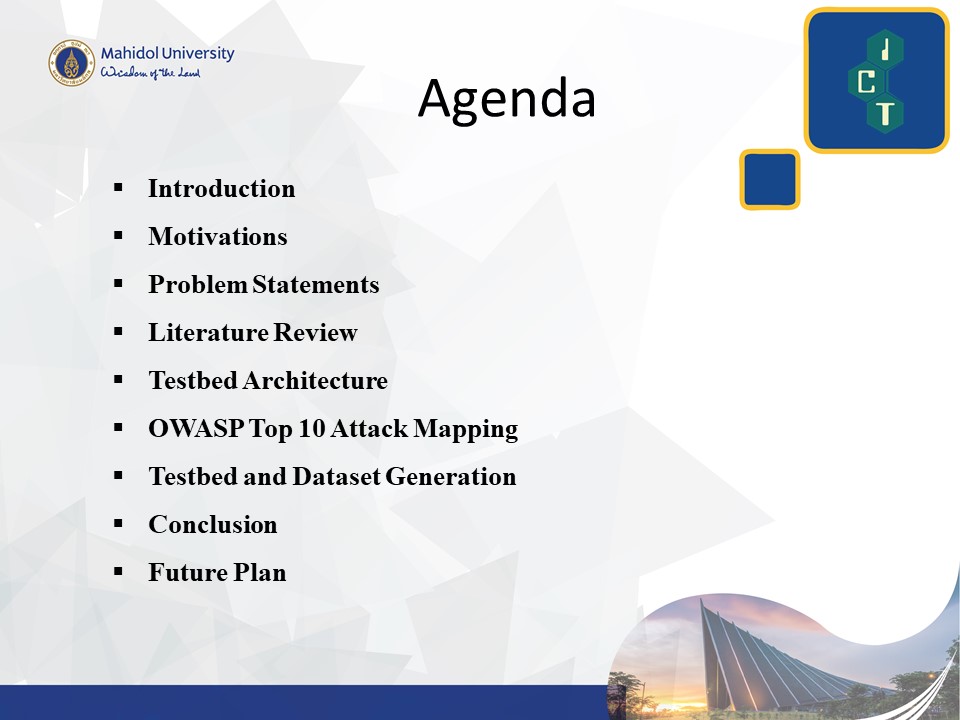 |
First of all, we will talk about the Introduction, Motivations, Problem Statements and Literature Review. And it will be followed by the Testbed Architecture, OWASP Top 10 Attack Mapping, and the Testbed and Dataset Generation. And finally, the Conclusion and the Future Plan will be presented.
|
ICACT20220314 Slide.01
[Big Slide]
[YouTube] |
Chrome Click!! |
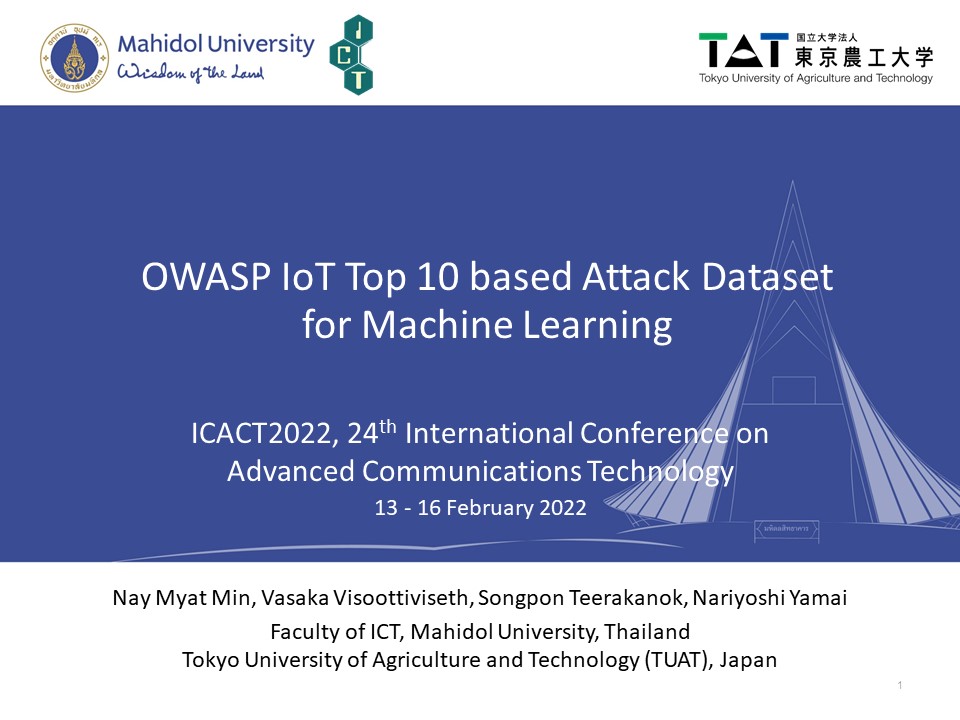 |
Hello everyone. Welcome to my presentation. My name is Nay Myat Min. I am a master¡¯s student in Mahidol University. Today, I will be presenting my paper, ¡°OWASP IoT Top 10 based Attack Dataset for Machine Learning¡±. Let me start with an agenda for today¡¯s presentation.
|
| |