ICACT20230246 Slide.19
[Big slide for presentation]
[YouTube] |
Chrome Text-to-Speach Click!! |
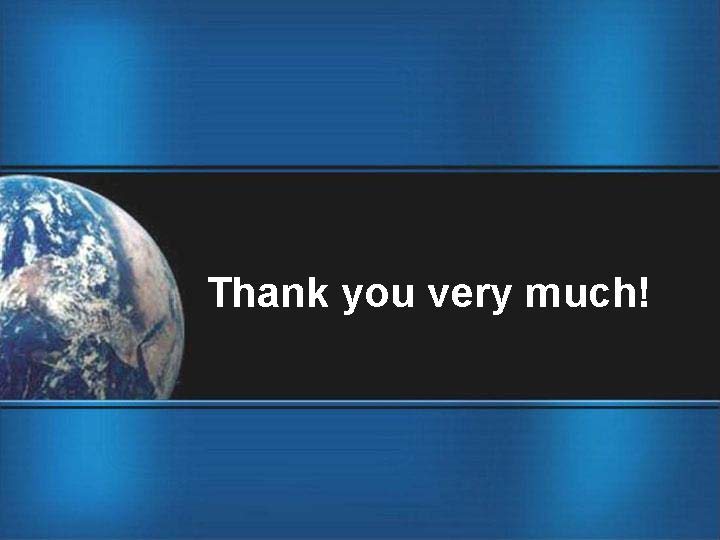 |
That's all.Thank you very much!
|
ICACT20230246 Slide.18
[Big slide for presentation]
[YouTube] |
Chrome Text-to-Speach Click!! |
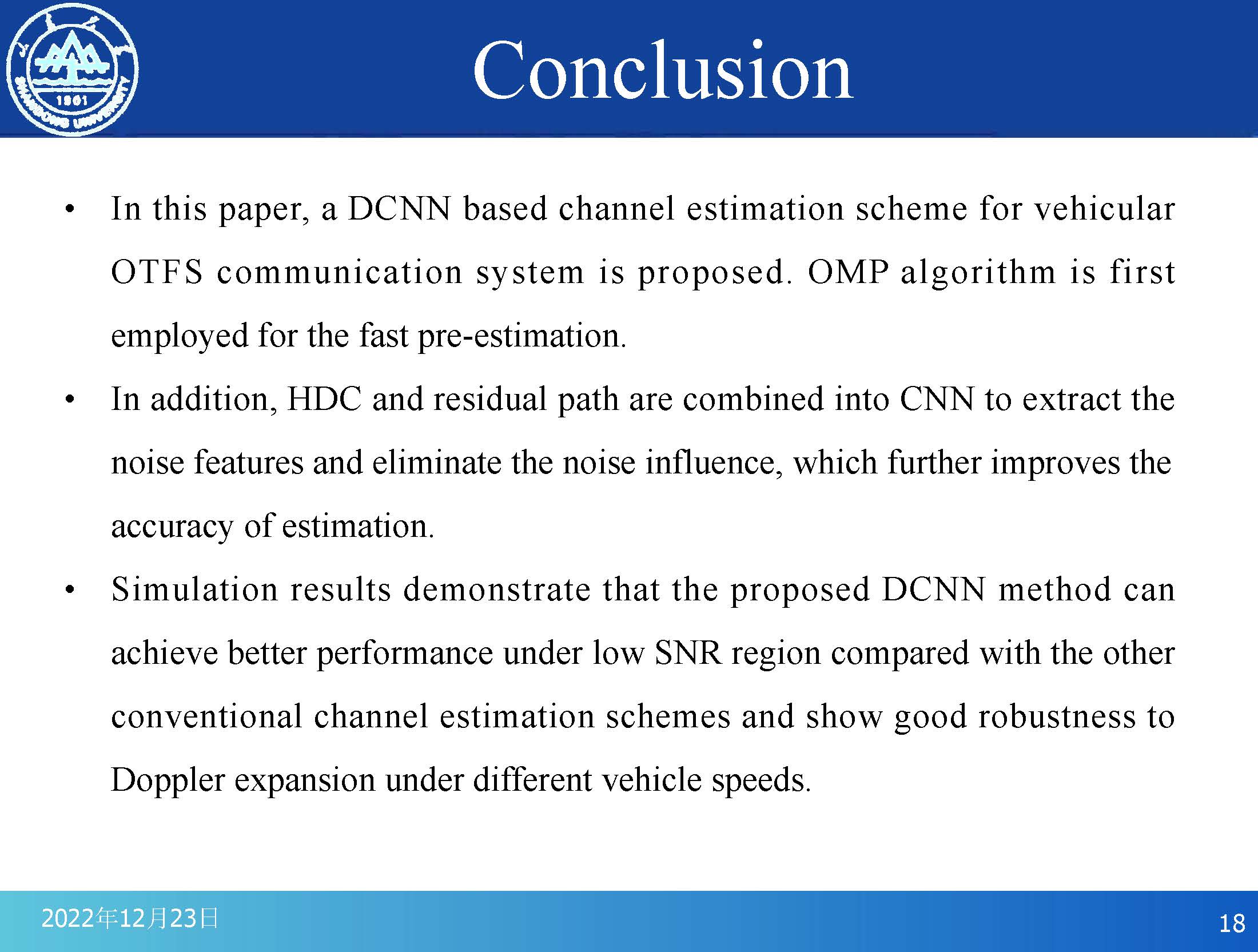 |
Finally,it is conclusion.
In this paper, a DCNN based channel estimation scheme for vehicular OTFS communication system is proposed. OMP algorithm is first employed for the fast pre-estimation. In addition, HDC and residual path are combined into CNN to extract the noise features and eliminate the noise influence, which further improves the accuracy of estimation. Simulation results demonstrate that the proposed DCNN method can achieve better performance under low SNR region compared with the other conventional channel estimation schemes and show good robustness to Doppler expansion under different vehicle speeds.
|
ICACT20230246 Slide.17
[Big slide for presentation]
[YouTube] |
Chrome Text-to-Speach Click!! |
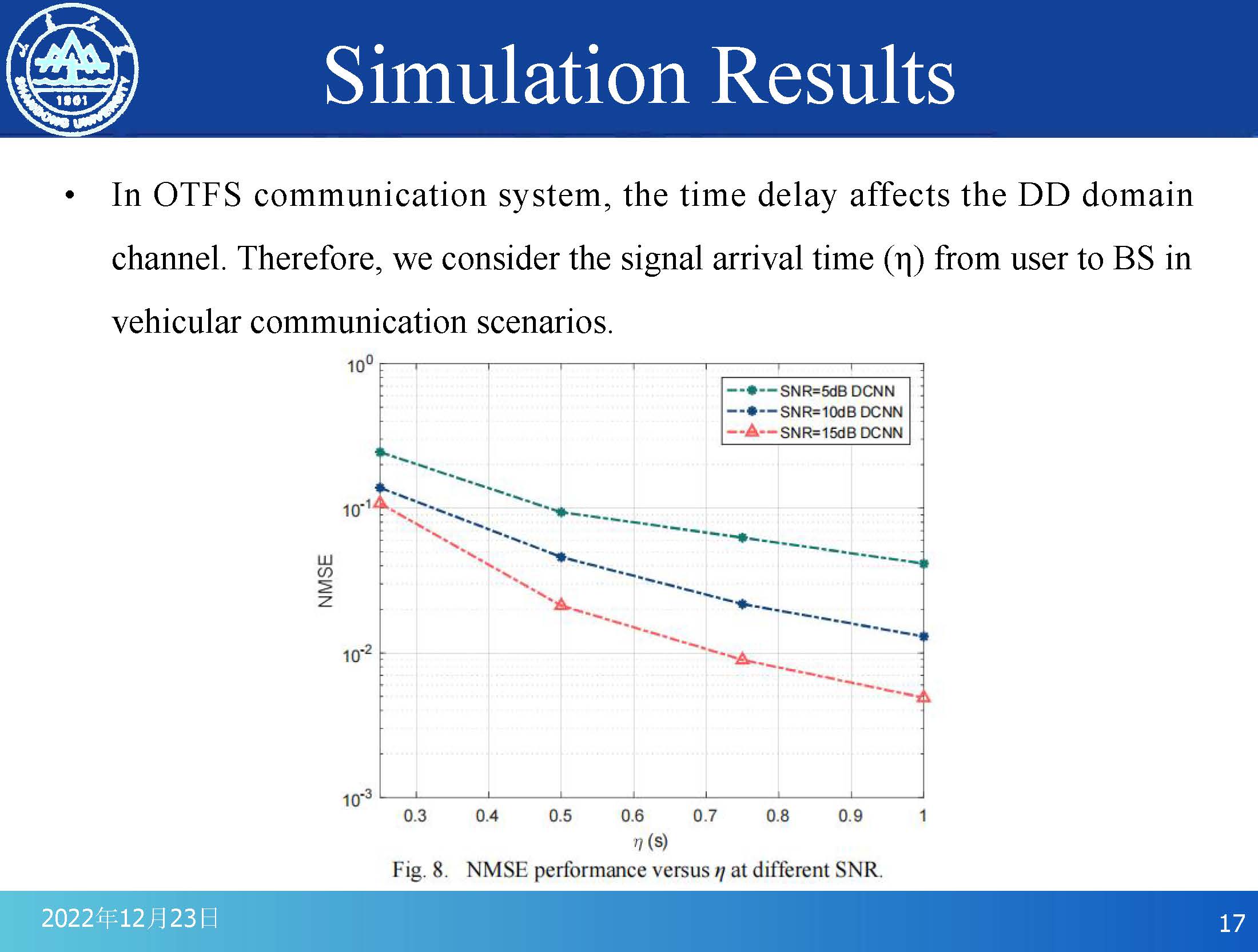 |
In OTFS communication system, the time delay affects the DD domain channel. Therefore, we consider the signal arrival time (亦) from user to BS in vehicular communication scenarios. In the simulation, We set the vehicle speed to 80 km/h uniformly. Fig. 8 depicts the effect of 亦 on the NMSE performance at different SNR. It is found that the NMSE performance gradually improves when 亦 increases at the same SNR. Since the higher delay can improve the delay resolution in the DD domain, thus making channel state characteristics better. Meanwhile, the NMSE performance of the DCNN method does not varies significantly when 亦 changes from 0.25s to 1s, which proves that the DCNN method has good robustness under different 亦 and has better channel estimation stability in different vehicular communication scenarios.
|
ICACT20230246 Slide.16
[Big slide for presentation]
[YouTube] |
Chrome Text-to-Speach Click!! |
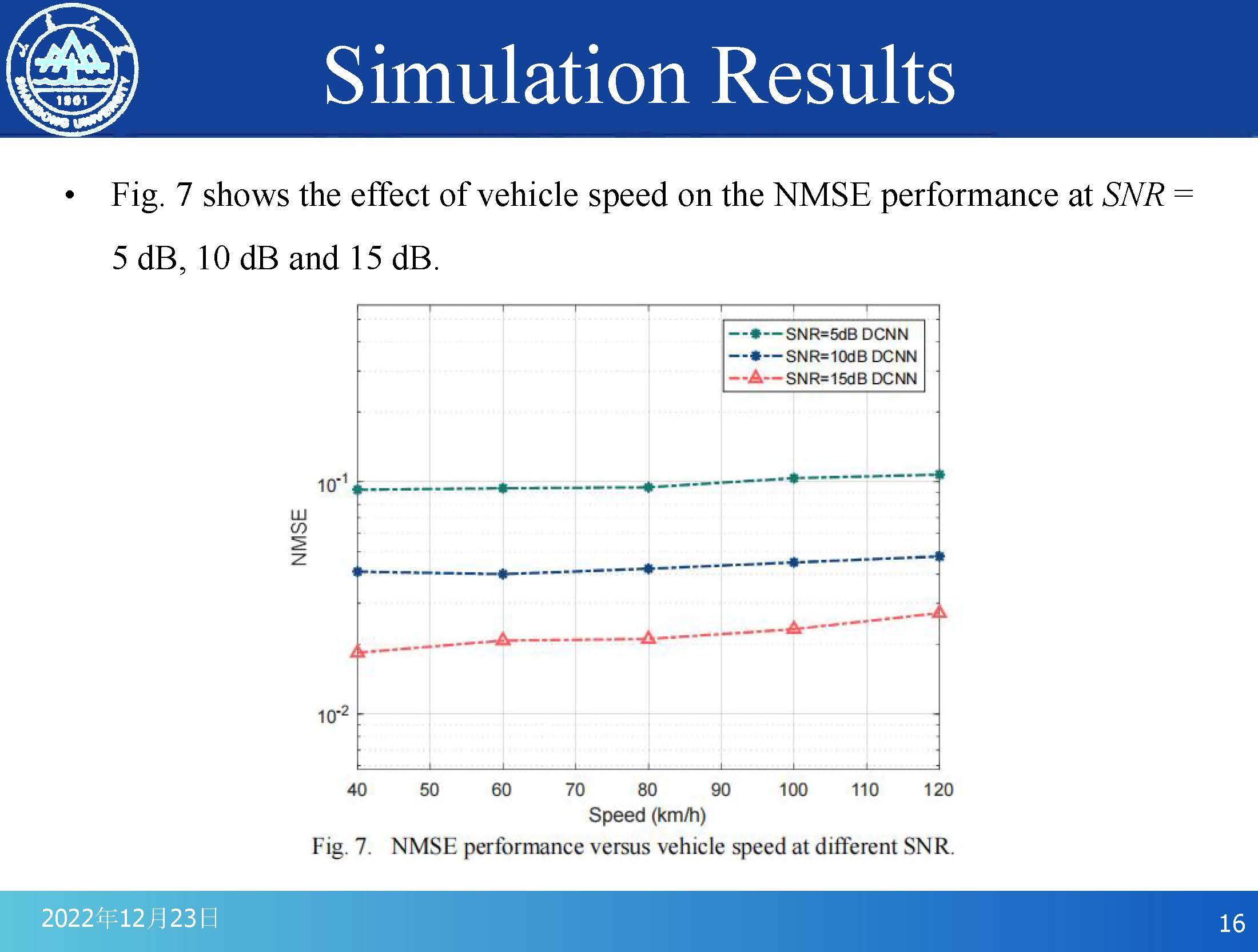 |
In the context of mobile vehicular communication, the channel parameters vary quickly with the increase of the vehicle speed. According to the characteristics of the DCNN method, it has good robustness to the variation of channel parameters. Fig. 7 shows the effect of vehicle speed on the NMSE performance at SNR = 5 dB, 10 dB and 15 dB. It is seen that the NMSE performance gradually decreases when SNR increases at the same speed. When the SNR is fixed, the vehicle speed increases from 40 km/h to 120 km/h, but the NMSE performance is almost unchanged. This is because the DCNN method has a strong adaptability to the variable channel parameters. Therefore, the DCNN method proposed in this paper has good robustness under different vehicle speeds, which can realize the channel estimation with high accuracy even when the vehicle speed is changed and enhance the reliability of communication in the vehicular communication scenarios.
|
ICACT20230246 Slide.15
[Big slide for presentation]
[YouTube] |
Chrome Text-to-Speach Click!! |
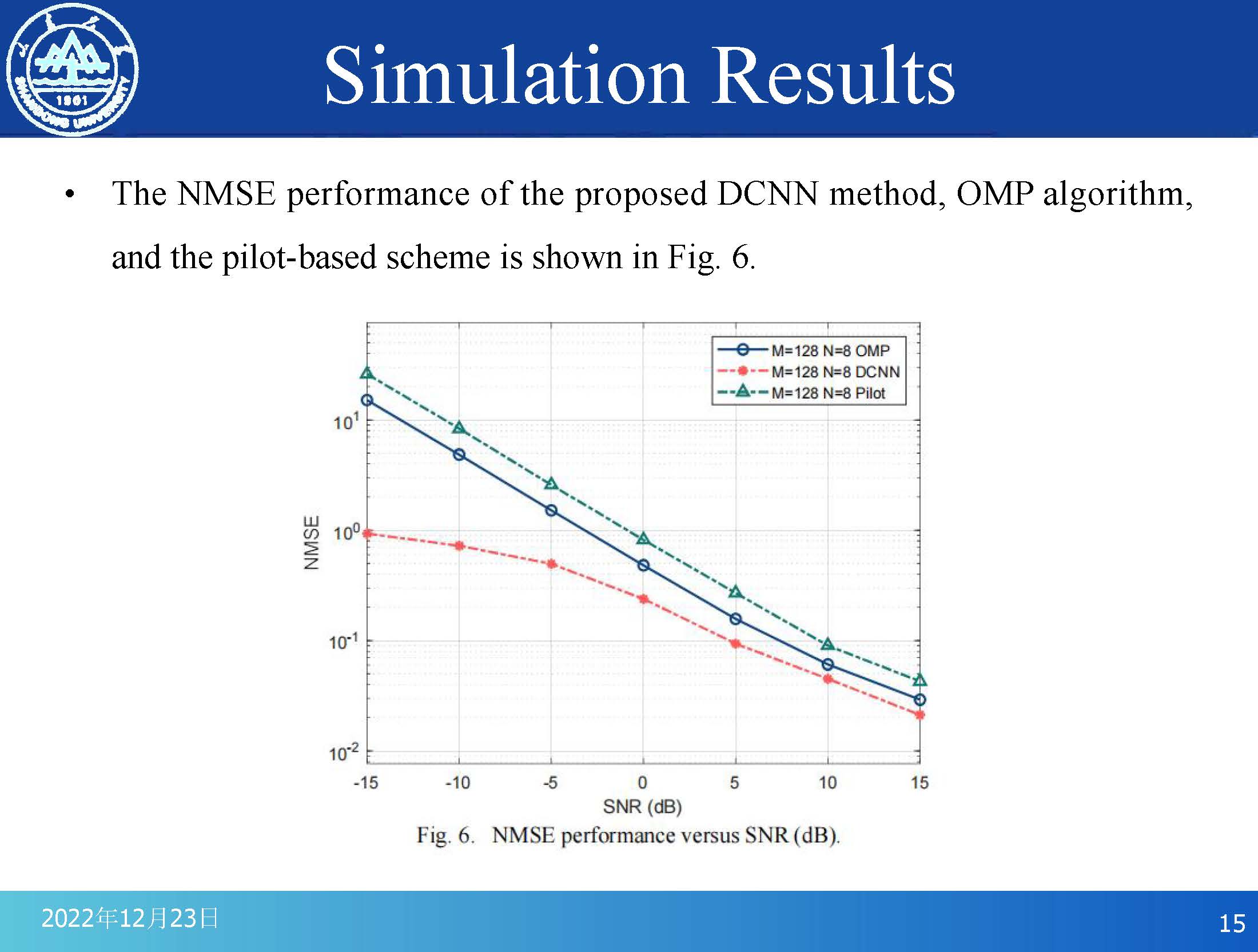 |
The NMSE performance of the proposed DCNN method, OMP algorithm, and the pilot-based scheme is shown in Fig. 6.
It is found that the DCNN method performs much better than OMP algorithm and the pilot-based scheme because the DCNN method is a nonlinear estimator that can learn the noise features and eliminate the noise. In addition, the DCNN method outperforms the other two conventional schemes, especially in the case of low SNR since the DCNN method can realize better noise feature extraction and achieve the noise cancellation well when the noise interference is strong.
|
ICACT20230246 Slide.14
[Big slide for presentation]
[YouTube] |
Chrome Text-to-Speach Click!! |
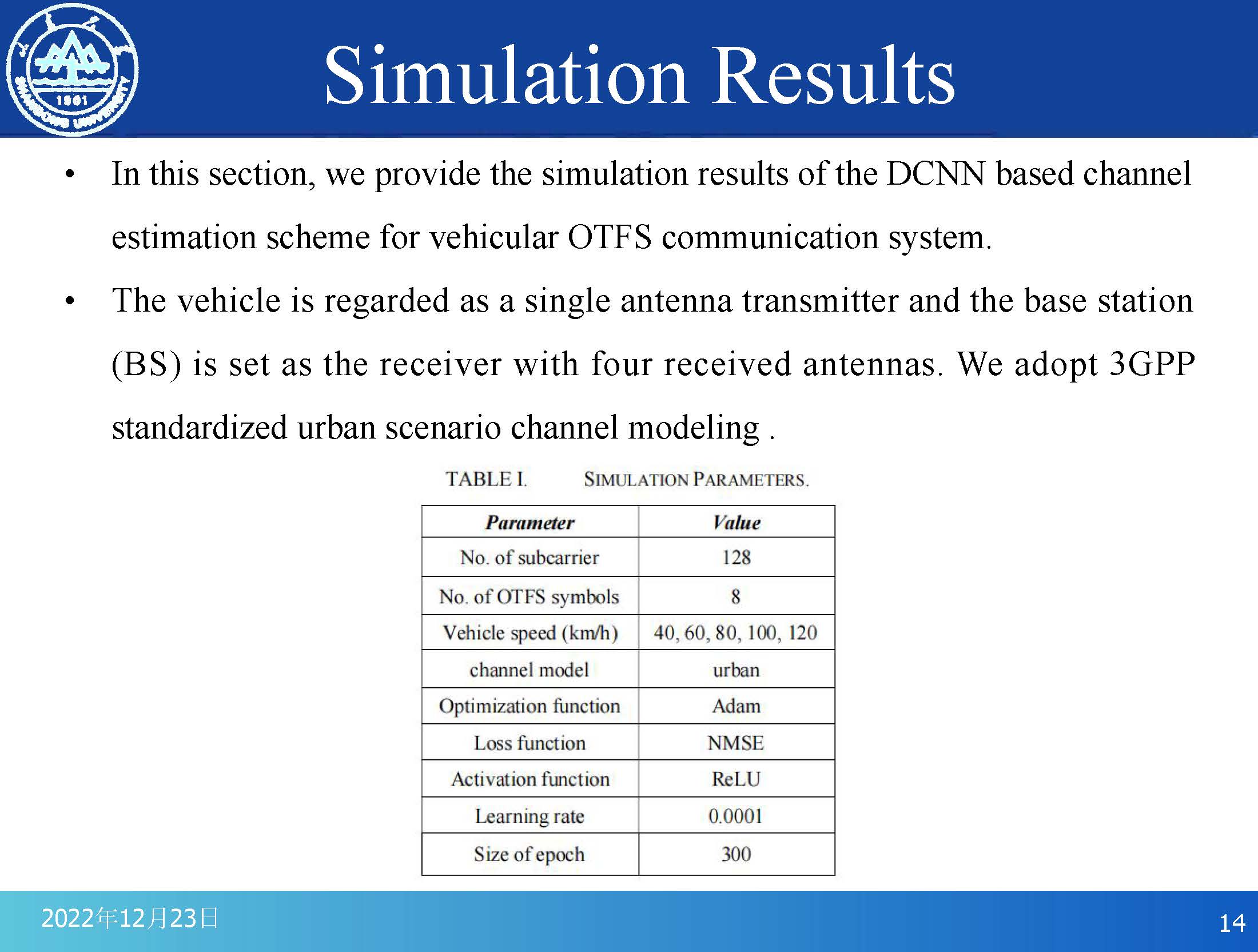 |
In this section, we provide the simulation results of the DCNN based channel estimation scheme for vehicular OTFS communication system. The vehicle is regarded as a single antenna transmitter and the base station(BS) is set as the receiver with four received antennas. We adopt 3GPP standardized urban scenario channel modeling .
|
ICACT20230246 Slide.13
[Big slide for presentation]
[YouTube] |
Chrome Text-to-Speach Click!! |
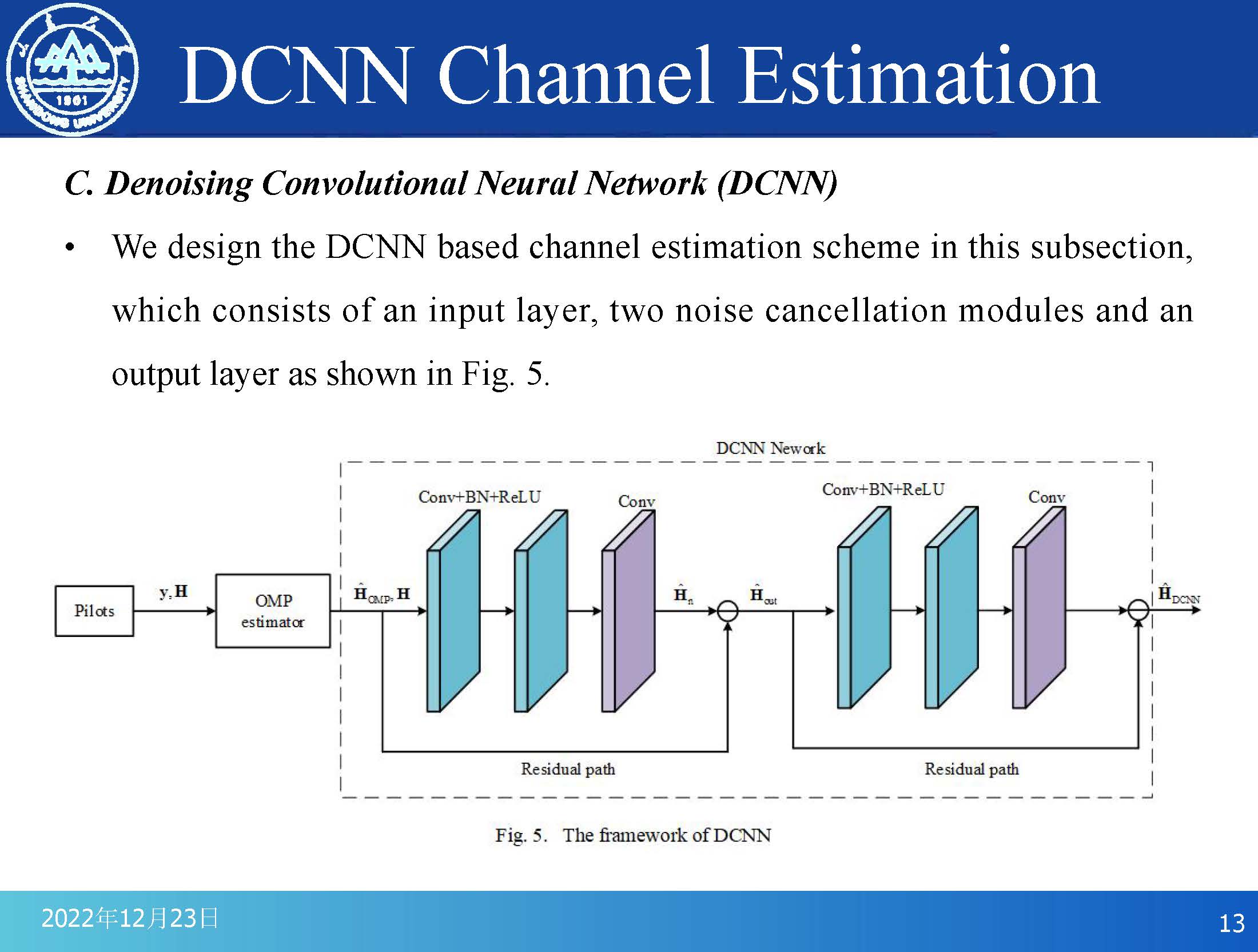 |
Now we introduce our networkㄩDenoising Convolutional Neural Network (DCNN).
We design the DCNN based channel estimation scheme in this subsection,
which consists of an input layer, two noise cancellation modules and an
output layer as shown in Fig. 5.
Each noise cancellation module has a residual path and three convolutional layers of 3▼3 size, and the expansion dilation rates of these three convolutional layers are set to be 1, 2, and 5, respectively. First, the pre-estimated channel matrix HOMP obtained by the OMP algorithm is taken as the input of DCNN, and the real channel matrix H is used as the label. Through the first noise cancellation module, the DCNN distinguishes the difference between HOMP and H to achieve the extraction of the noise matrix Hn. Subsequently, the channel matrix Hout from the output layer of the first noise cancellation module is obtained by subtracting Hn from HOMP through the residual path. Finally, Hout is used as the input of the second noise cancellation module, and the actual channel matrix H is also set as the label, and the above operation is repeated to obtain the final channel estimation matrix HDCNN of DCNN.
The above channel estimation can eliminate the noise interference and achieve better accuracy.
|
ICACT20230246 Slide.12
[Big slide for presentation]
[YouTube] |
Chrome Text-to-Speach Click!! |
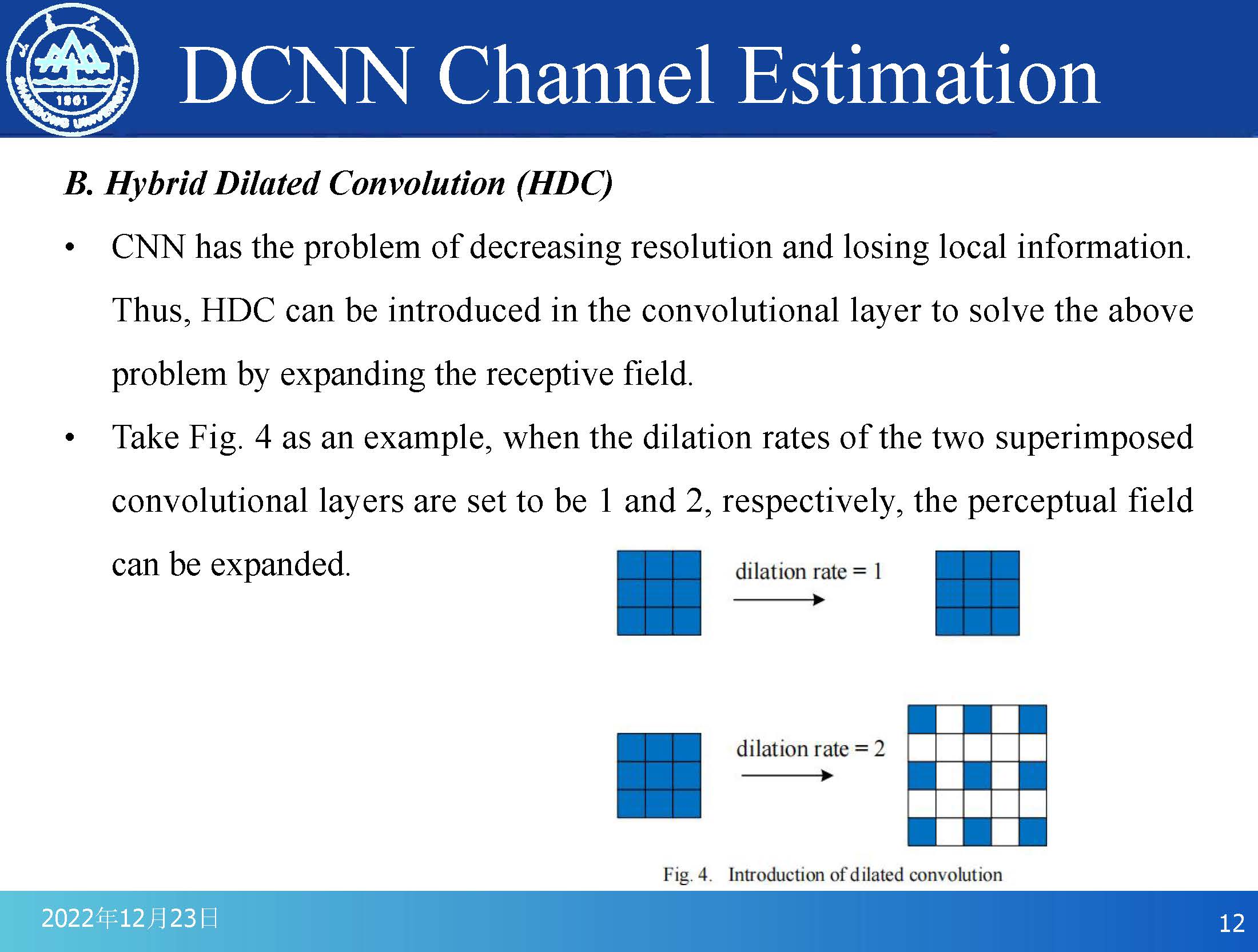 |
Now let*s talk about Hybrid Dilated Convolution (HDC).
CNN has the problem of decreasing resolution and losing local information. Thus, HDC can be introduced in the convolutional layer to solve the above problem by expanding the receptive field. Take Fig. 4 as an example, when the dilation rates of the two superimposed convolutional layers are set to be 1 and 2, respectively, the perceptual field can be expanded.
|
ICACT20230246 Slide.11
[Big slide for presentation]
[YouTube] |
Chrome Text-to-Speach Click!! |
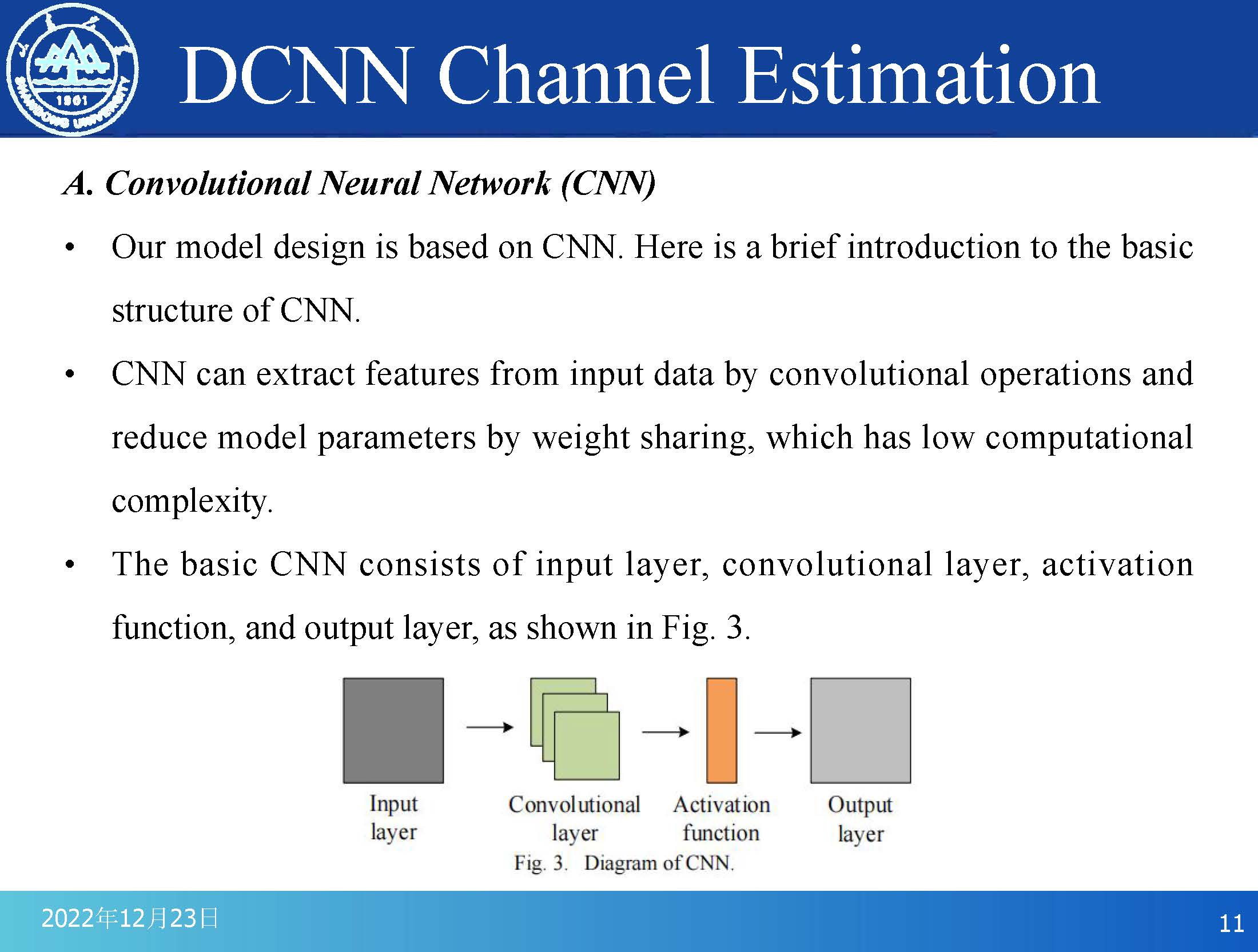 |
Our model design is based on CNN. Here is a brief introduction to the basic structure of CNN. CNN can extract features from input data by convolutional operations and reduce model parameters by weight sharing, which has low computational complexity. The basic CNN consists of input layer, convolutional layer, activation function, and output layer, as shown in Fig. 3.
|
ICACT20230246 Slide.10
[Big slide for presentation]
[YouTube] |
Chrome Text-to-Speach Click!! |
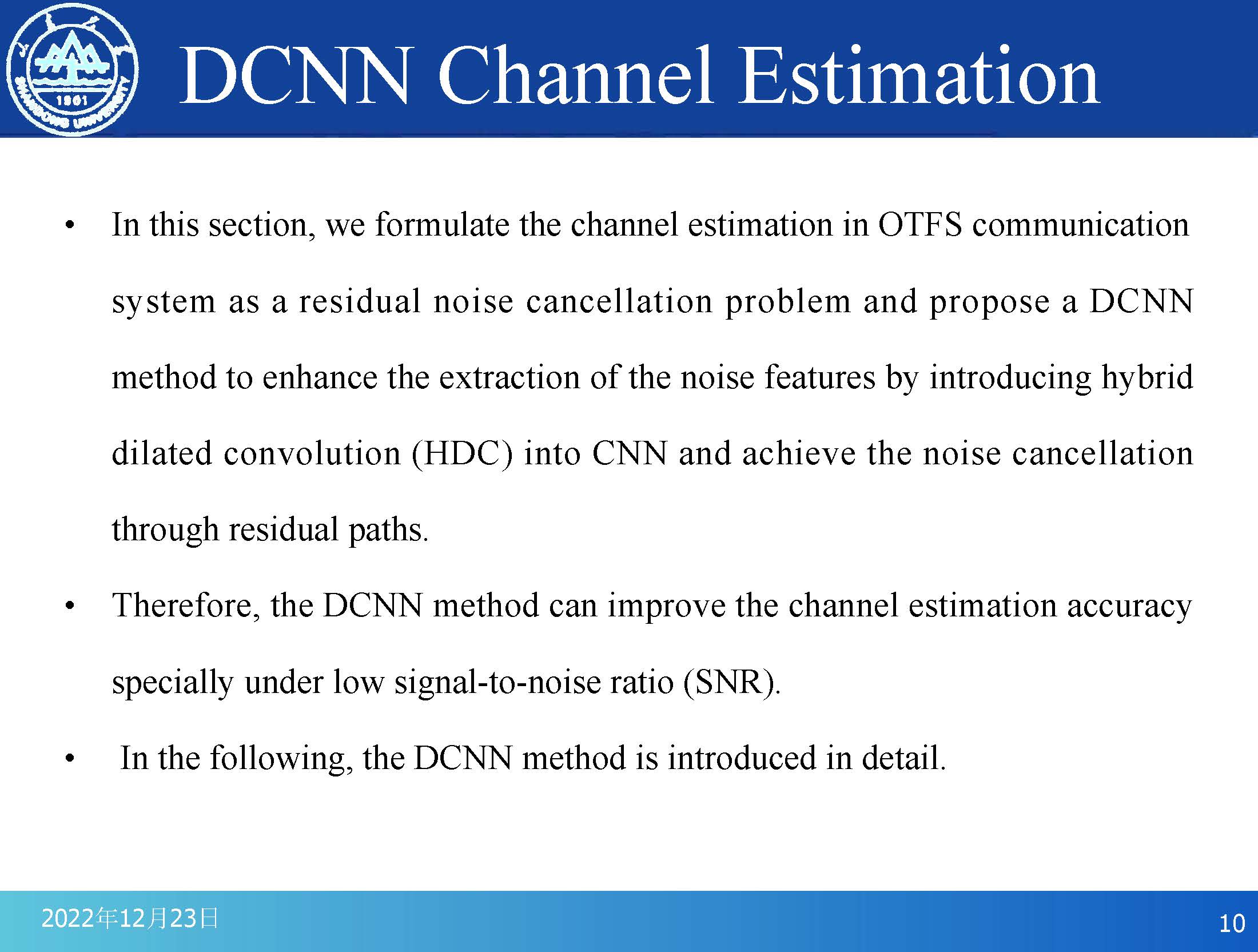 |
Next, let's introduce our DCNN channel estimation.
In this section, we formulate the channel estimation in OTFS communication system as a residual noise cancellation problem and propose a DCNN method to enhance the extraction of the noise features by introducing hybrid dilated convolution (HDC) into CNN and achieve the noise cancellation through residual paths. Therefore, the DCNN method can improve the channel estimation accuracy specially under low signal-to-noise ratio (SNR). In the following, the DCNN method is introduced in detail.
|
ICACT20230246 Slide.09
[Big slide for presentation]
[YouTube] |
Chrome Text-to-Speach Click!! |
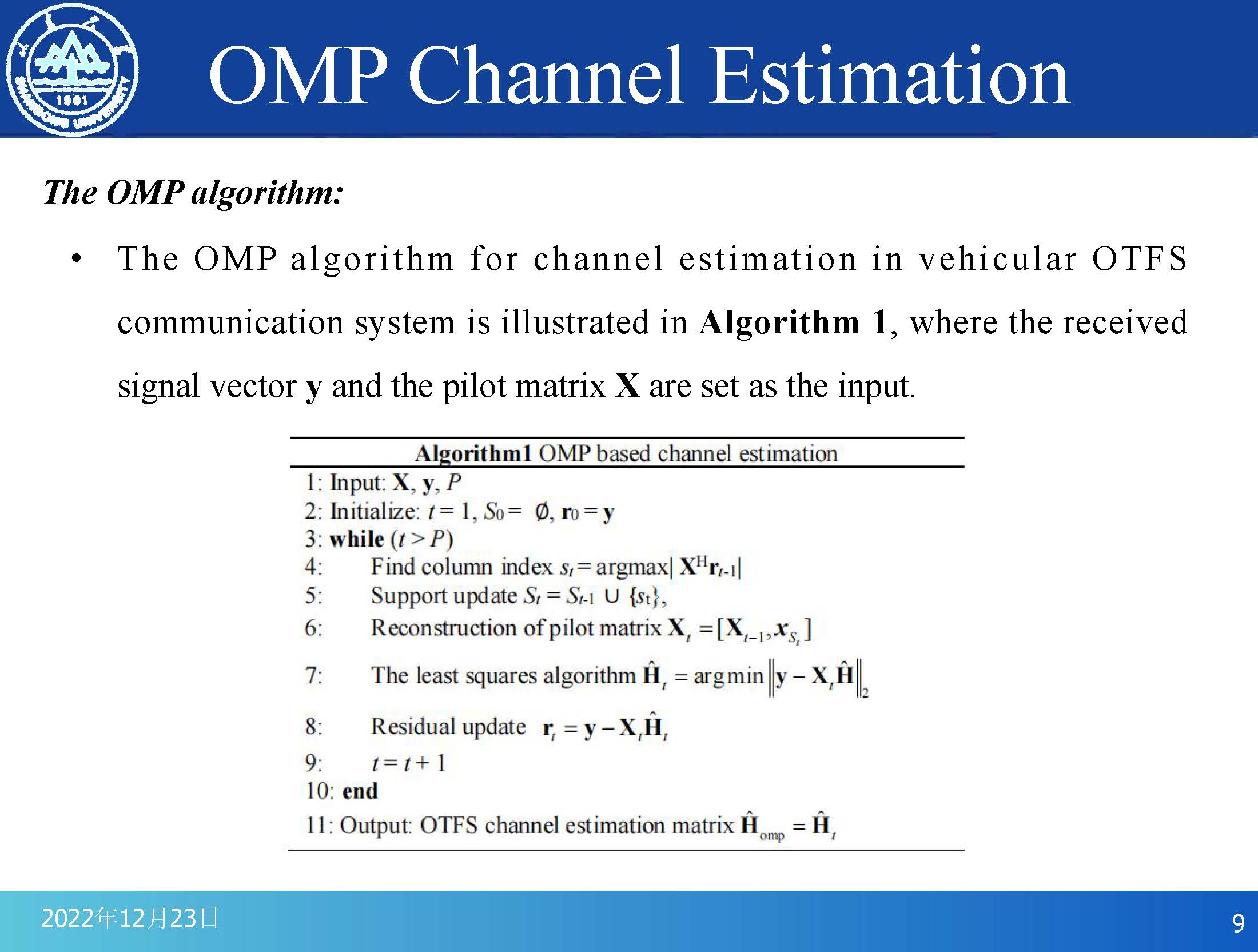 |
The OMP algorithm for channel estimation in vehicular OTFS communication system is illustrated in Algorithm 1, where the received signal vector y and the pilot matrix X are set as the input.
As we can see, In the t-th iteration, the residual rt-1 of the previous iteration is projected onto each column of X. The index st of the column with the largest absolute product value is selected and added to the current support St and the pilot matrix X is updated. Then the least square (LS) method is employed to obtain the t-th estimated vector Ht in the channel matrix, and the residual rt is updated. Finally, set the iteration number as t = t + 1. Once t > P is satisfied, the iteration is stopped and the newly obtained Ht is the final estimation result HOMP, otherwise the above OMP algorithm operates continuously.
|
ICACT20230246 Slide.08
[Big slide for presentation]
[YouTube] |
Chrome Text-to-Speach Click!! |
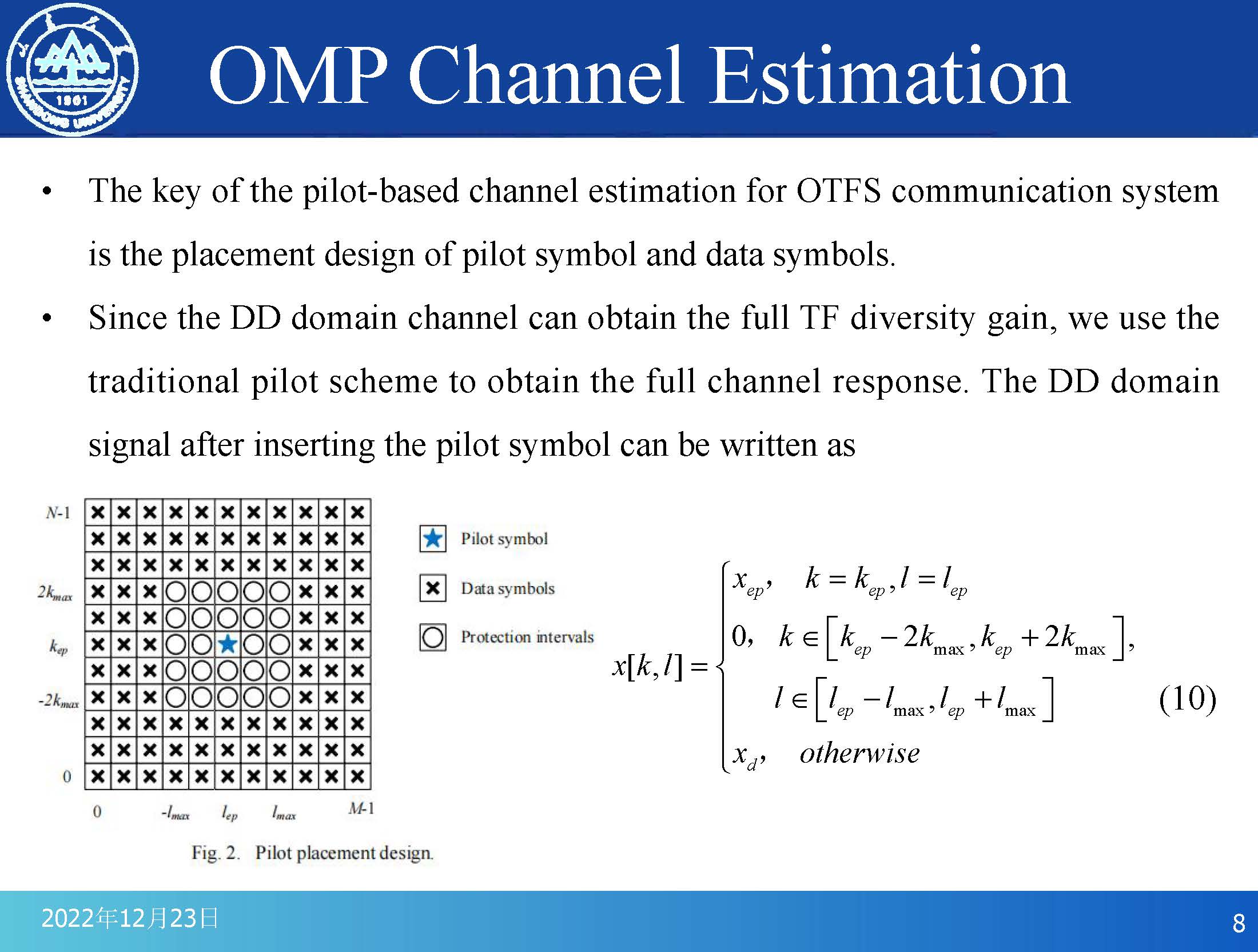 |
Next, we will introduce the OMP channel estimation algorithm.
The key of the pilot-based channel estimation for OTFS communication system is the placement design of pilot symbol and data symbols.
Since the DD domain channel can obtain the full TF diversity gain, we use the traditional pilot scheme to obtain the full channel response. The DD domain signal after inserting the pilot symbol can be written as formula (10).
|
ICACT20230246 Slide.07
[Big slide for presentation]
[YouTube] |
Chrome Text-to-Speach Click!! |
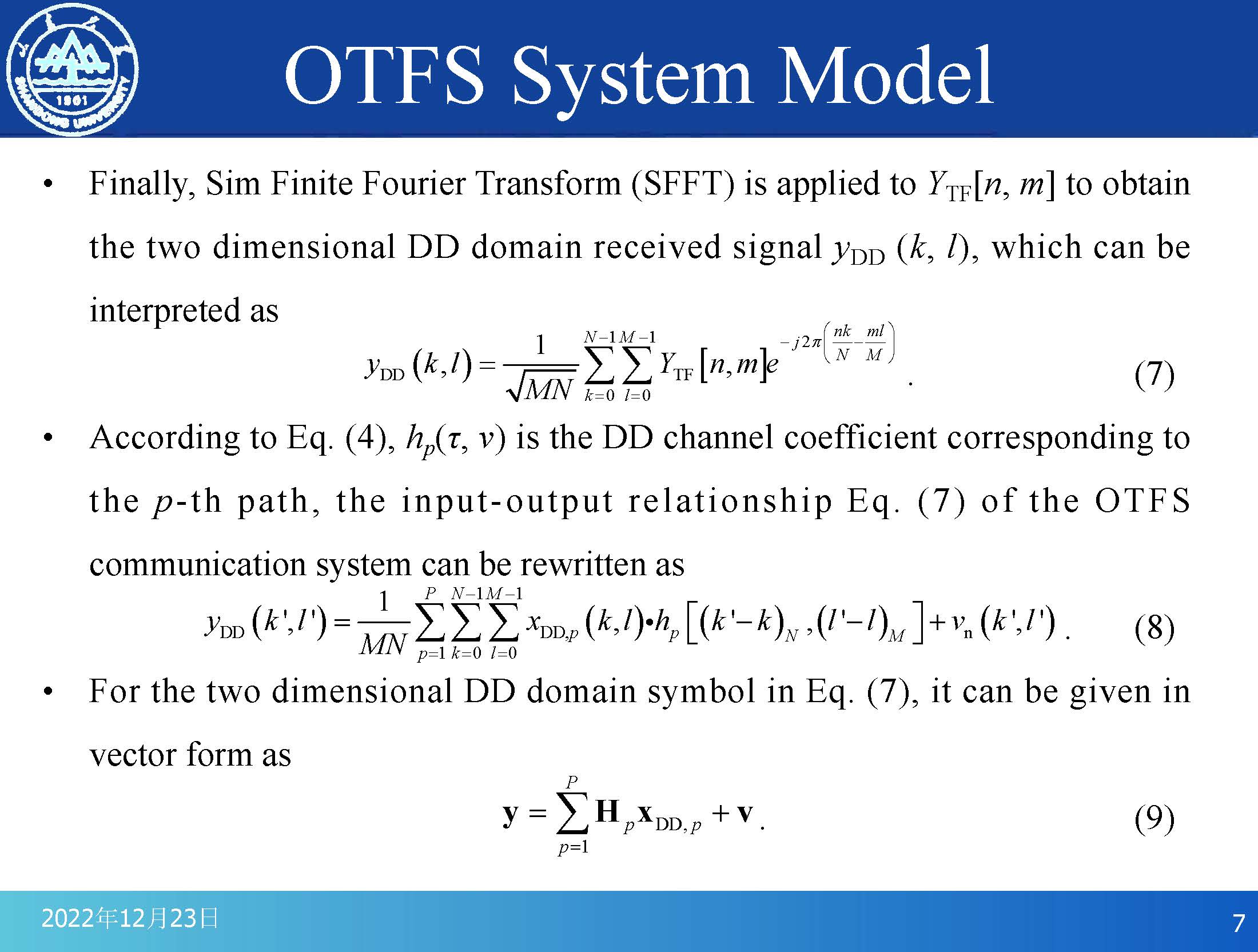 |
Finally, Sim Finite Fourier Transform (SFFT) is applied to YTF[n, m] to obtain the two dimensional DD domain received signal yDD (k, l), which can be interpreted as (7). According to Eq. (4), hp(件, v) is the DD channel coefficient corresponding to the p-th path, the input-output relationship Eq. (7) of the OTFS communication system can be rewritten as (8). For the two dimensional DD domain symbol in Eq. (7), it can be given in vector form as (9).
|
ICACT20230246 Slide.06
[Big slide for presentation]
[YouTube] |
Chrome Text-to-Speach Click!! |
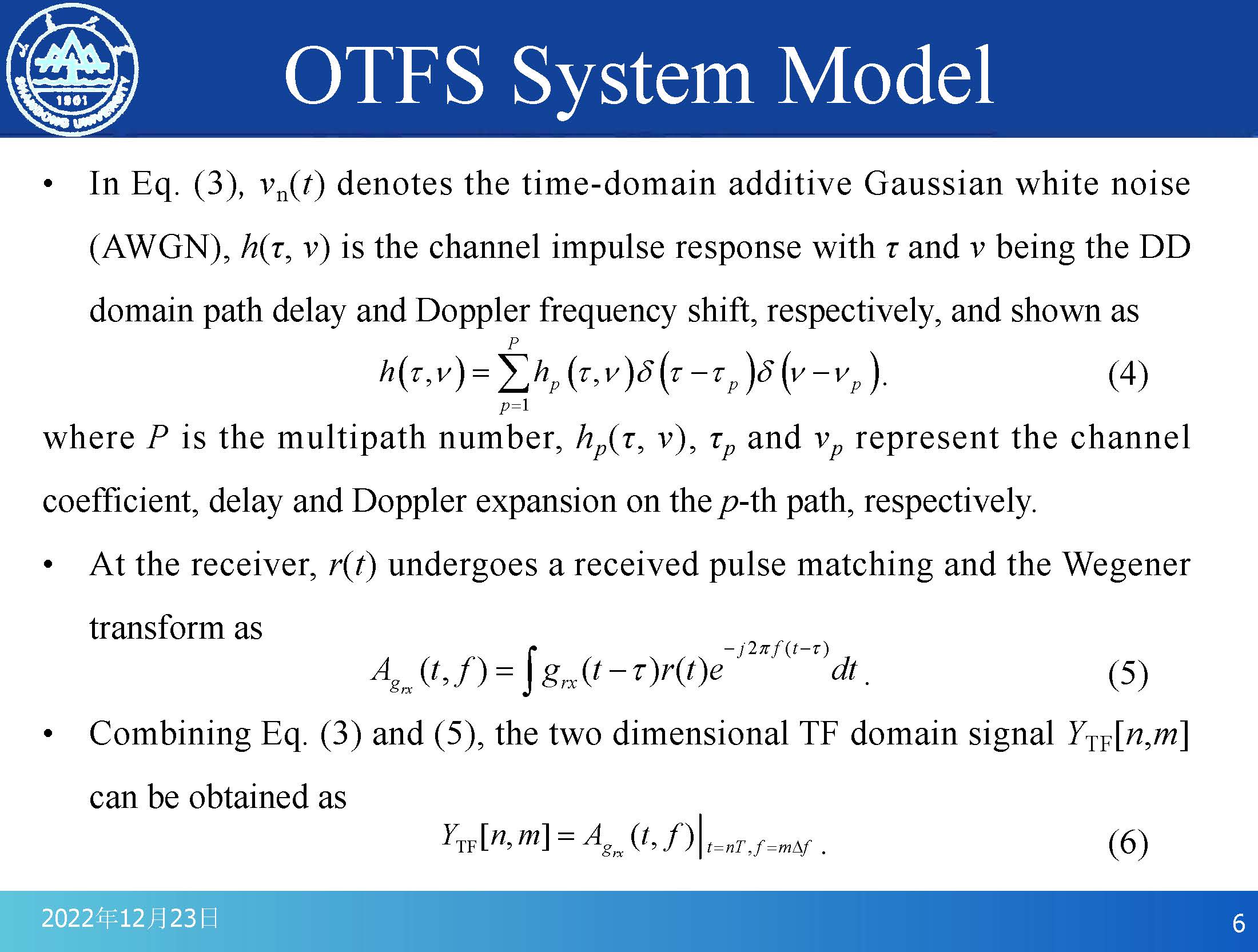 |
In Eq. (3), vn(t) denotes the time-domain additive Gaussian white noise(AWGN), h(件, v) is the channel impulse response with 件 and v being the DD domain path delay and Doppler frequency shift, respectively, and shown as (4), where P is the multipath number, hp(件, v), 件p and vp represent the channel coefficient, delay and Doppler expansion on the p-th path, respectively. At the receiver, r(t) undergoes a received pulse matching and the Wegener transform as (5). Combining Eq. (3) and (5), the two dimensional TF domain signal YTF[n,m] can be obtained as (6).
|
ICACT20230246 Slide.05
[Big slide for presentation]
[YouTube] |
Chrome Text-to-Speach Click!! |
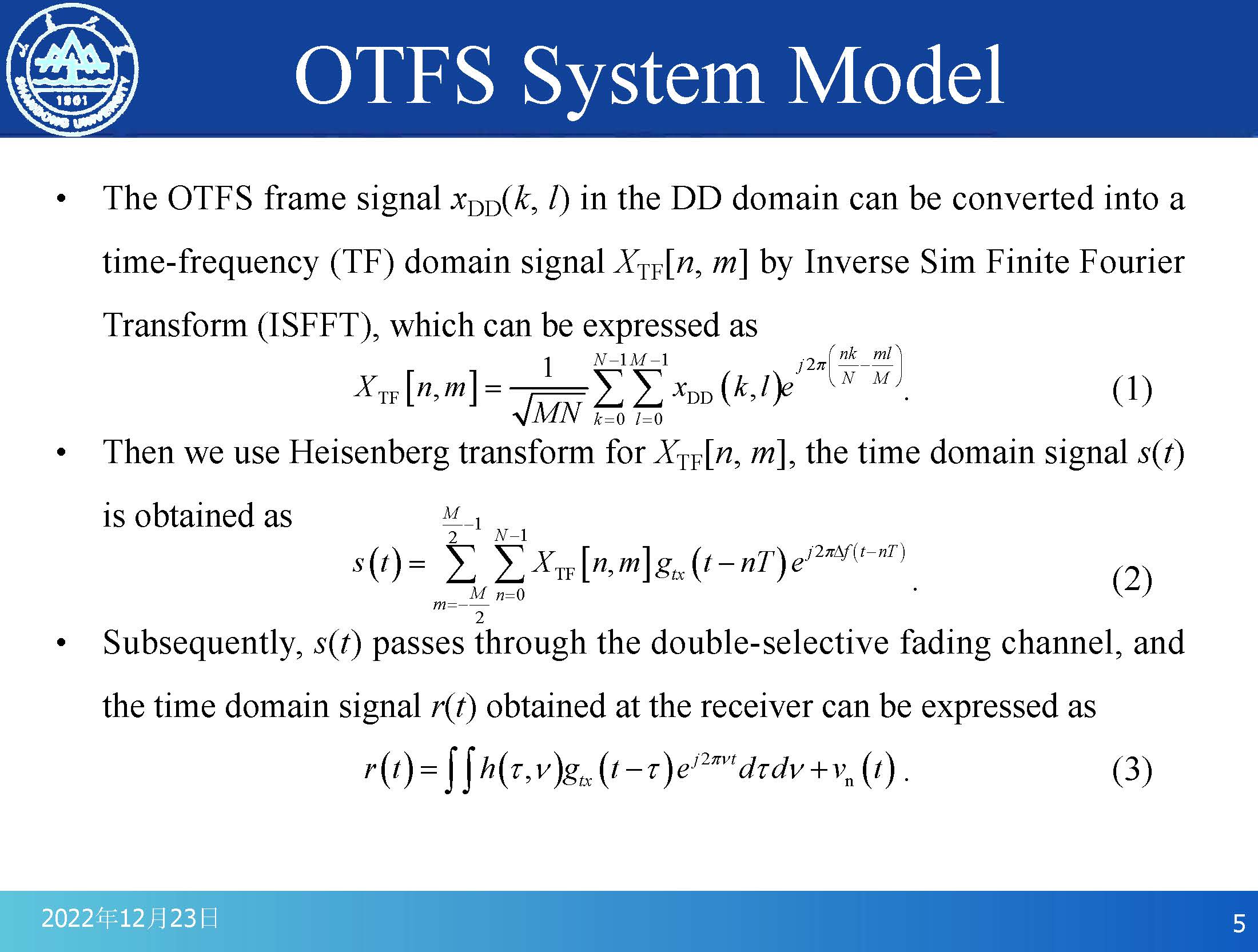 |
The OTFS frame signal xDD(k, l) in the DD domain can be converted into a time-frequency (TF) domain signal XTF[n, m] by Inverse Sim Finite Fourier Transform (ISFFT), which can be expressed as (1). Then we use Heisenberg transform for XTF[n, m], the time domain signal s(t) is obtained as (2). Subsequently, s(t) passes through the double-selective fading channel, and the time domain signal r(t) obtained at the receiver can be expressed as (3).
So, the above is the basic process operation of OTFS transmitter.
|
ICACT20230246 Slide.04
[Big slide for presentation]
[YouTube] |
Chrome Text-to-Speach Click!! |
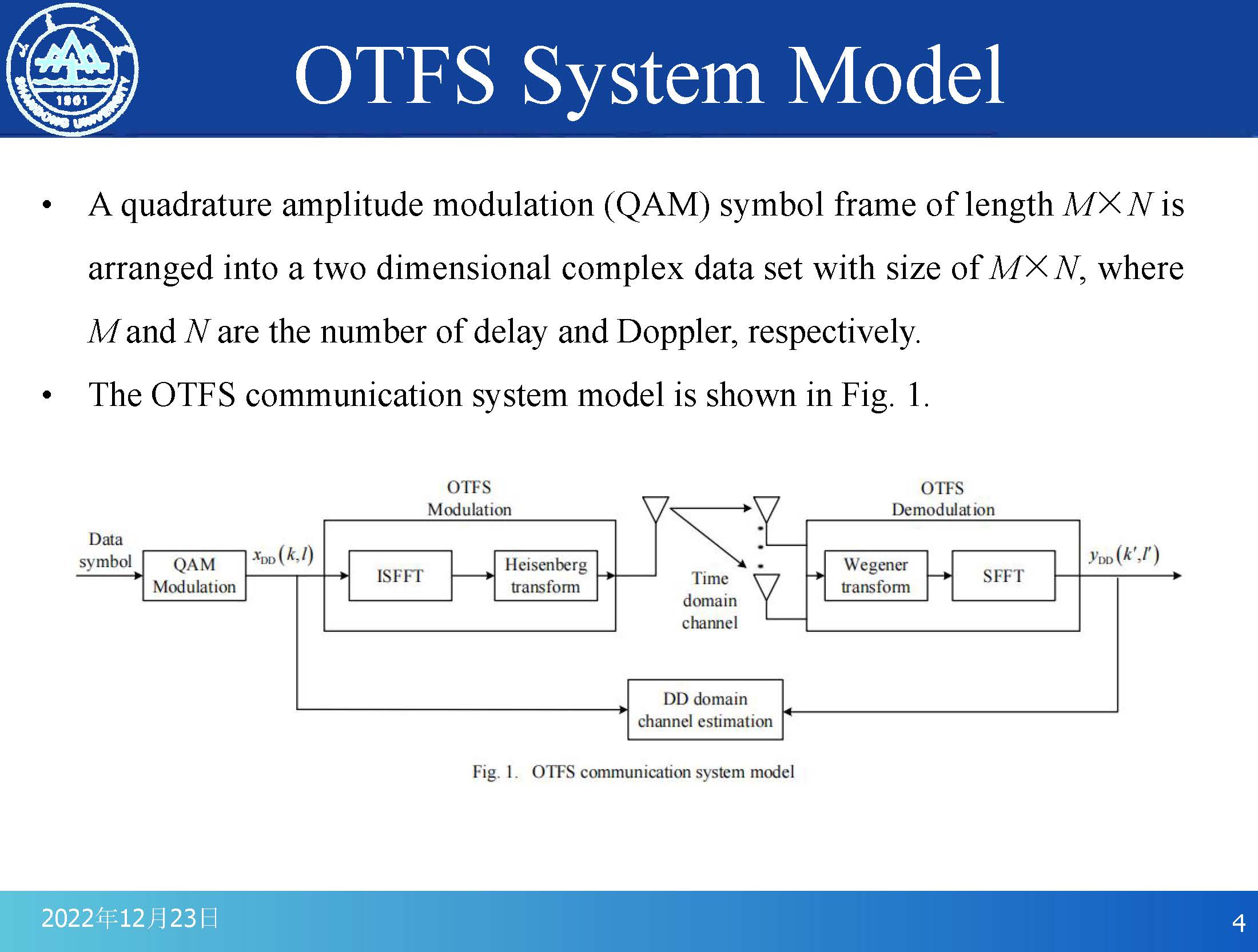 |
Then, let me introduce the OTFS system model.
As we can see in Fig. 1, the data symbol must first be modulated by QAM. A QAM symbol frame of length M multiple N is arranged into a two dimensional complex data set with size of M multiple N, where M and N are the number of delay and Doppler, respectively.
|
ICACT20230246 Slide.03
[Big slide for presentation]
[YouTube] |
Chrome Text-to-Speach Click!! |
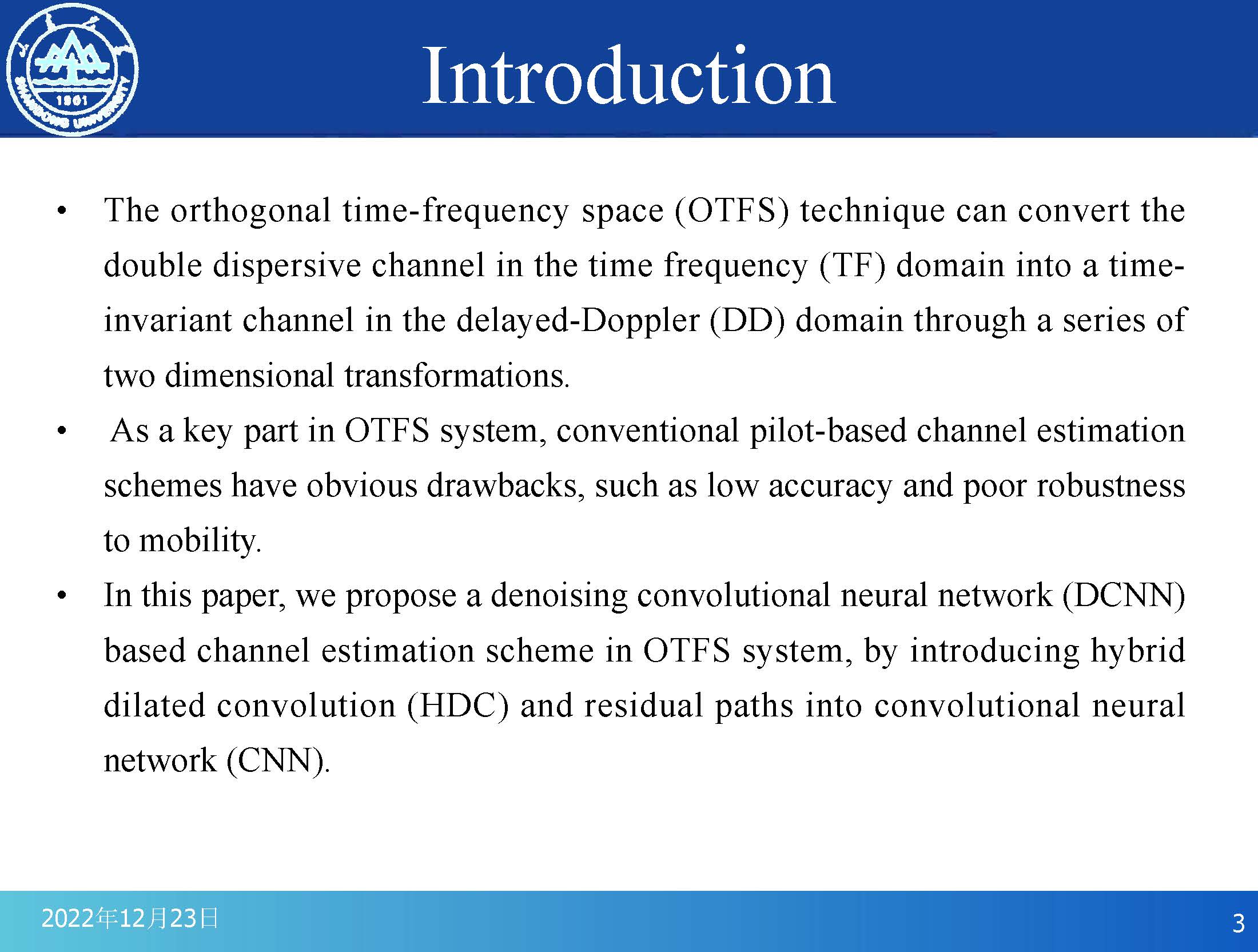 |
First of all, let me briefly introduce the work of this paper.
The orthogonal time-frequency space (OTFS) technique can convert the double dispersive channel in the time frequency (TF) domain into a time-invariant channel in the delayed-Doppler (DD) domain through a series of two dimensional transformations.
As a key part in OTFS system, conventional pilot-based channel estimation schemes have obvious drawbacks, such as low accuracy and poor robustness to mobility.
In this paper, we propose a denoising convolutional neural network (DCNN) based channel estimation scheme in OTFS system, by introducing hybrid dilated convolution (HDC) and residual paths into convolutional neural network (CNN).
|
ICACT20230246 Slide.02
[Big slide for presentation]
[YouTube] |
Chrome Text-to-Speach Click!! |
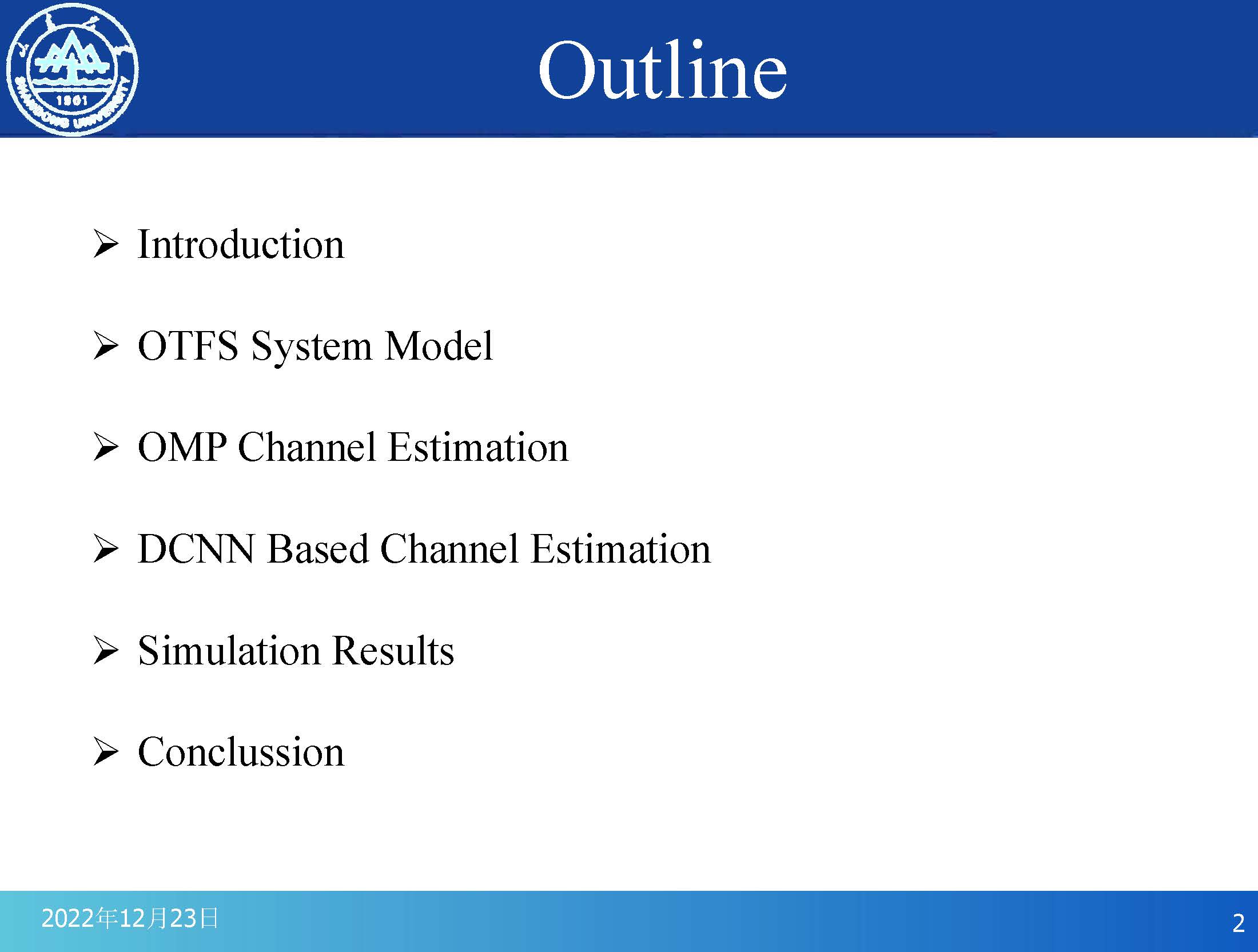 |
Next, I will describe the following six aspectsㄩIntroduction﹜OTFS System Model﹜OMP Channel Estimation﹜DCNN Based Channel Estimation﹜Simulation Results and Conclussion.
|
ICACT20230246 Slide.01
[Big slide for presentation]
[YouTube] |
Chrome Text-to-Speach Click!! |
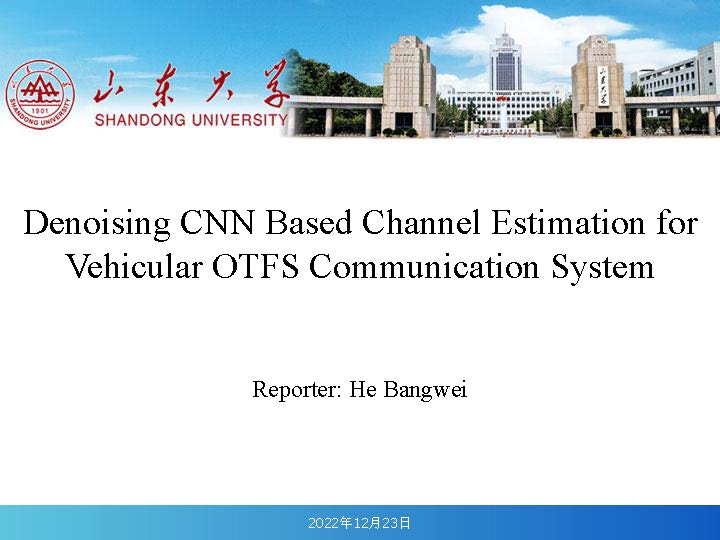 |
Hello, everyone. I am Bangwei He from Shandong University. Today I'm going to talk about my conference paperㄩDenoising CNN Based Channel Estimation for Vehicular OTFS Communication System
|