ICACT20200192 Slide.24
[Big slide for presentation]
[YouTube] |
Chrome Text-to-Speach Click!! |
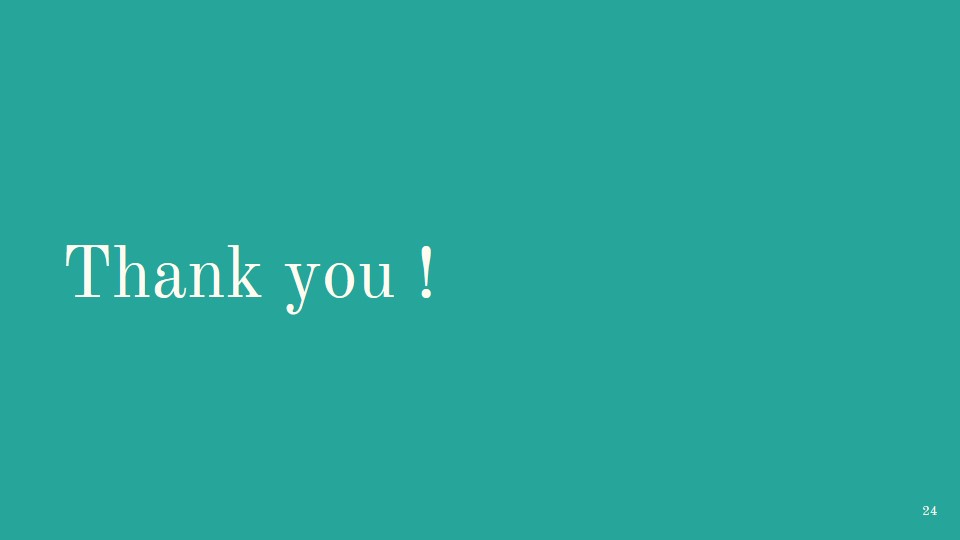 |
Thanks for your attention!
Now! Floor is open for QnA.
Welcome any comment or question.
Hi everybody, my name is Byeongnam Yoon.
For ICACT 2020, I have cooperated with two partners from ITI VNU.
|
ICACT20200192 Slide.23
[Big slide for presentation]
[YouTube] |
Chrome Text-to-Speach Click!! |
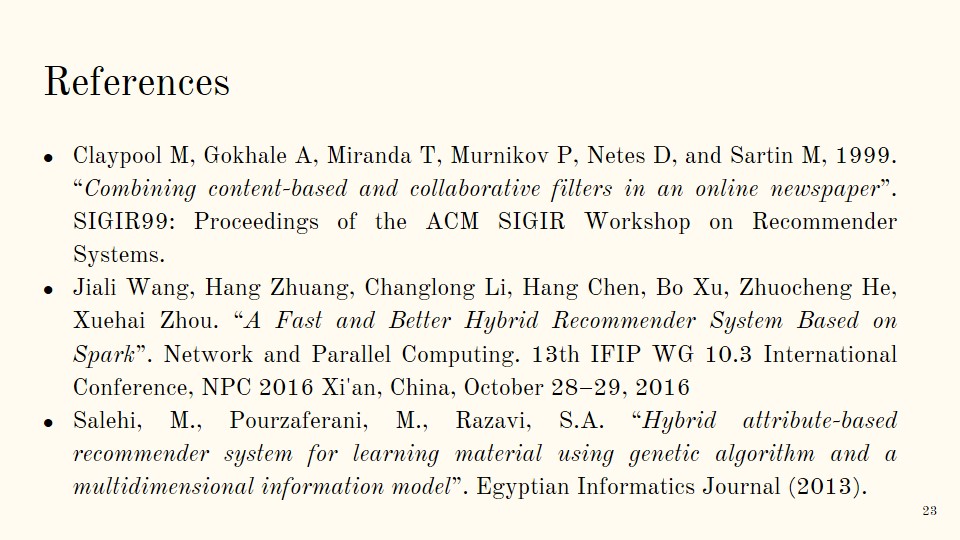 |
|
ICACT20200192 Slide.22
[Big slide for presentation]
[YouTube] |
Chrome Text-to-Speach Click!! |
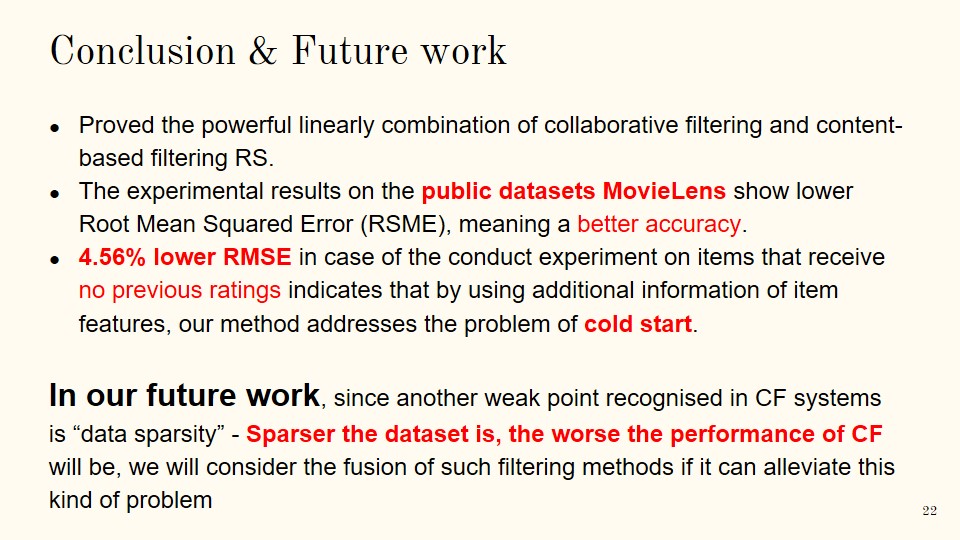 |
Proved the powerful linearly combination of collaborative filtering and content-based filtering RS.
The experimental results on the public datasets MovieLens show lower Root Mean Squared Error (RSME), meaning a better accuracy.
4.56% lower RMSE in case of the conduct experiment on items that receive no previous ratings indicates that by using additional information of item features, our method addresses the problem of cold start.
In our future work, since another weak point recognised in CF systems is “data sparsity” - Sparser the dataset is, the worse the performance of CF will be, we will consider the fusion of such filtering methods if it can alleviate this kind of problem
|
ICACT20200192 Slide.21
[Big slide for presentation]
[YouTube] |
Chrome Text-to-Speach Click!! |
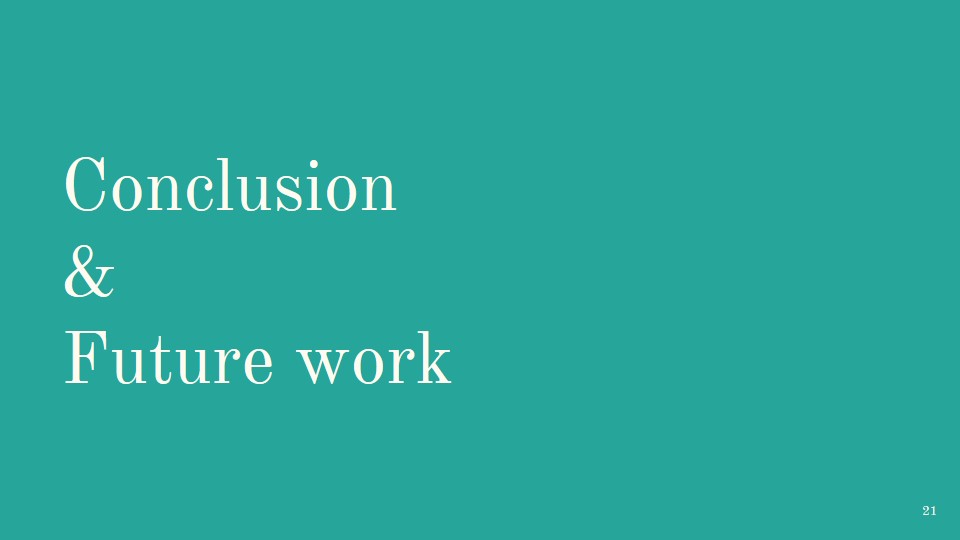 |
|
ICACT20200192 Slide.20
[Big slide for presentation]
[YouTube] |
Chrome Text-to-Speach Click!! |
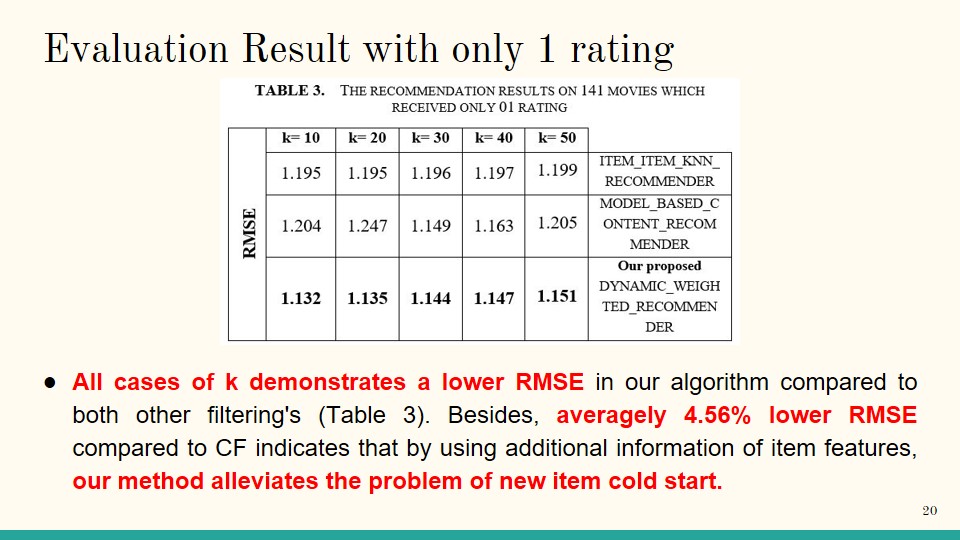 |
All cases of k demonstrates a lower RMSE in our algorithm compared to both other filtering's (Table 3). Besides, averagely 4.56% lower RMSE compared to CF indicates that by using additional information of item features, our method alleviates the problem of new item cold start.
|
ICACT20200192 Slide.19
[Big slide for presentation]
[YouTube] |
Chrome Text-to-Speach Click!! |
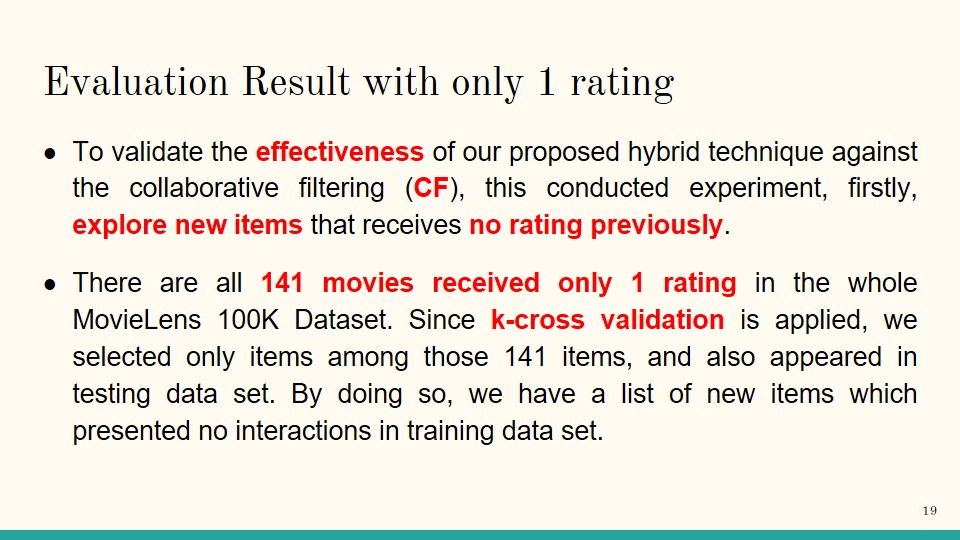 |
The quality of collaborative filtering recommendations is significantly affected by the number of ratings due to the fact that they rely on the item's interactions to make recommendations. When new items arrive with no or very little interactions, it leads to the problem of item cold start, in which a pure collaborative algorithm cannot recommend the item or the quality of those recommendations will be poor.
(Contd.)<above>
|
ICACT20200192 Slide.18
[Big slide for presentation]
[YouTube] |
Chrome Text-to-Speach Click!! |
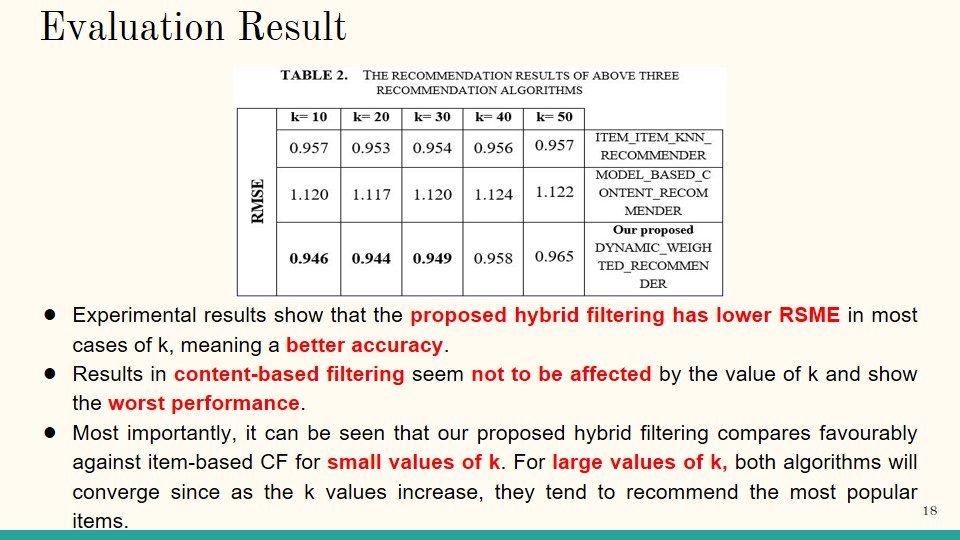 |
The experimental results show that the proposed hybrid filtering has lower RSME in most cases of k, meaning a better accuracy.
The results in content-based filtering seem not to be affected by the value of k and show the worst performance.
Most importantly, it can be seen that our proposed hybrid filtering compares favourably against item-based CF for small values of k. For large values of k, both algorithms will converge since as the k values increase, they tend to recommend the most popular items.
|
ICACT20200192 Slide.17
[Big slide for presentation]
[YouTube] |
Chrome Text-to-Speach Click!! |
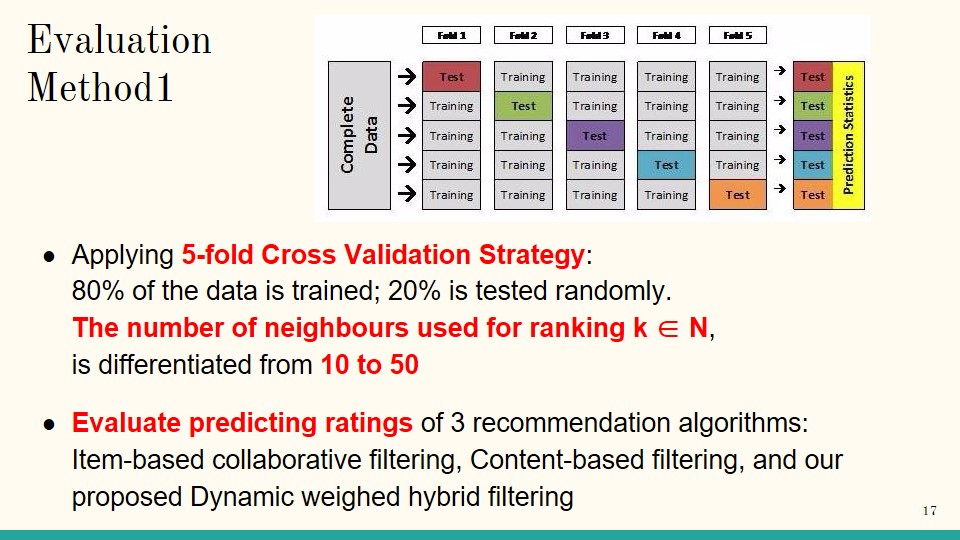 |
Evaluation 1
Applying 5-fold cross validation strategy: 80% of the data is trained; 20% is tested randomly. The number of neighbors used for ranking k ∈ N, is differentiated from 10 to 50
Compute predicting ratings of 3 recommendation algorithms: Item-based collaborative filtering, Content-based filtering, and our proposed Dynamic weighed hybrid filtering
|
ICACT20200192 Slide.16
[Big slide for presentation]
[YouTube] |
Chrome Text-to-Speach Click!! |
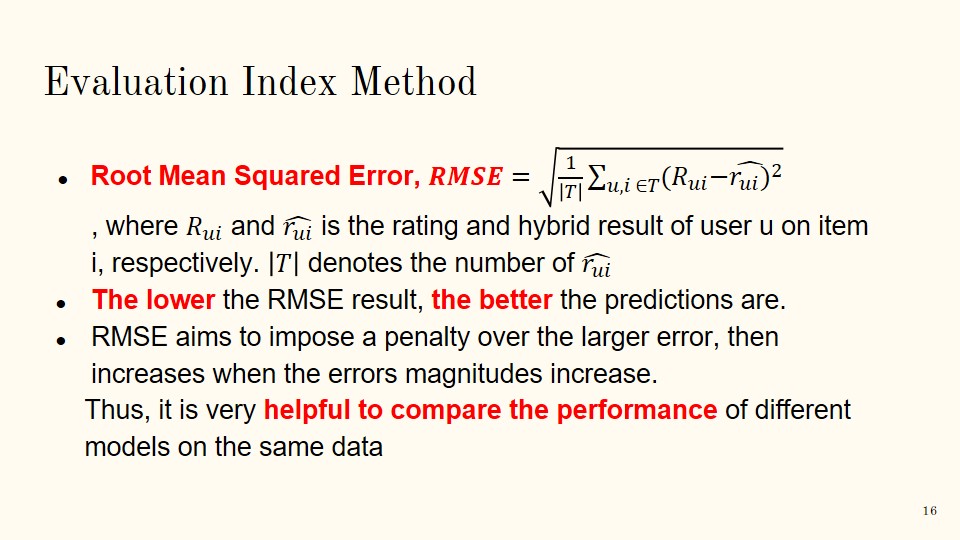 |
Root Mean Squared Error
The lower the RMSE result, the better the predictions are.
The RMSE aims to impose a penalty over the larger error, then increases when the errors magnitudes increase. Thus, it is very helpful to compare the performance of different models on the same data
|
ICACT20200192 Slide.15
[Big slide for presentation]
[YouTube] |
Chrome Text-to-Speach Click!! |
 |
The “observed” ratings are on a scale from 1 to 5. The value 0 can represent a missing value, then it is not displayed in the analysis (Figure 3). Most rating values are centered at 3-4. Average ratings is 3.52986.
|
ICACT20200192 Slide.14
[Big slide for presentation]
[YouTube] |
Chrome Text-to-Speach Click!! |
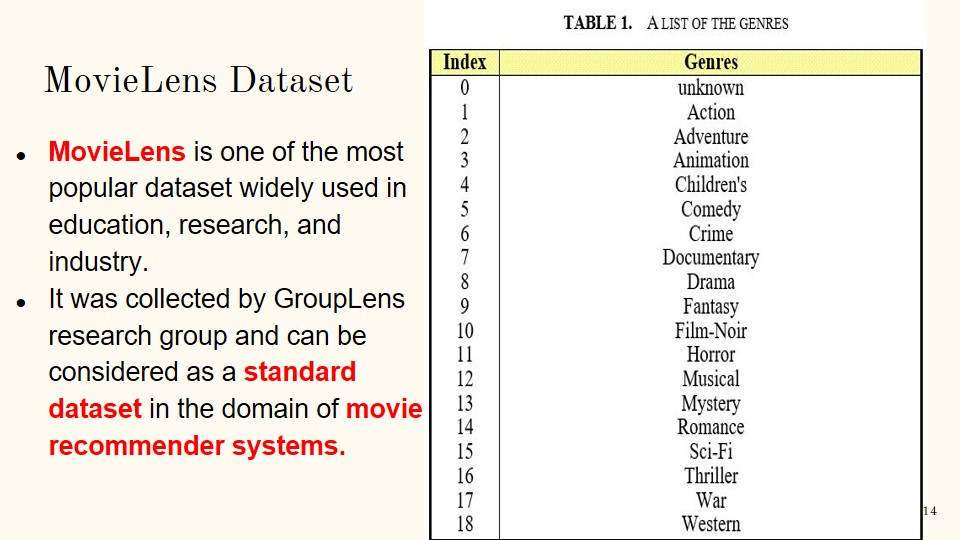 |
Datase
MovieLens is one of the most popular dataset widely used in education, research, and industry. It was collected by GroupLens research group and can be considered as a standard dataset in the domain of movie recommender systems.
|
ICACT20200192 Slide.13
[Big slide for presentation]
[YouTube] |
Chrome Text-to-Speach Click!! |
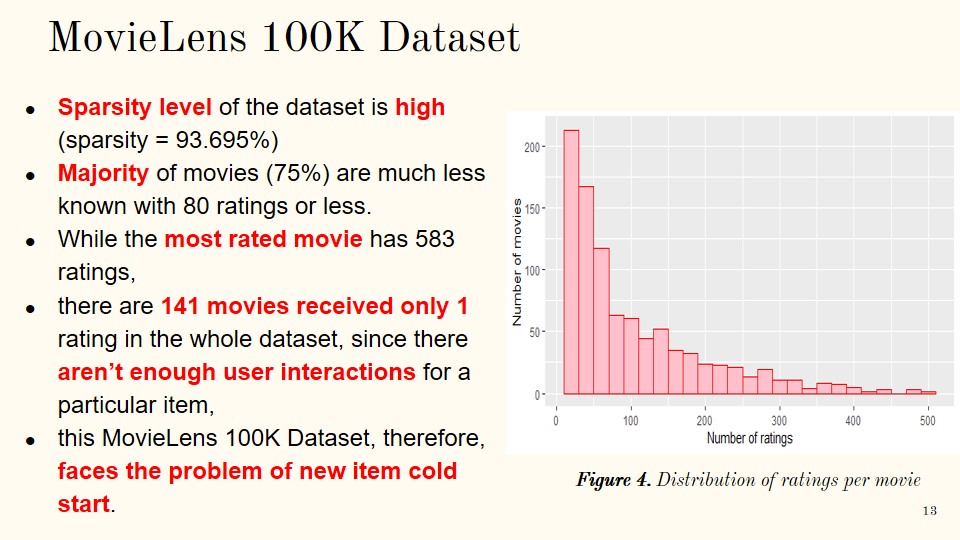 |
Dataset – MovieLens (Contd.)
The sparsity level of the dataset is high (sparsity = 93.695%)
The majority of movies (75%) are much less known with 80 ratings or less. While the most rated movie has 583 ratings, there are 141 movies received only 1 rating in the whole dataset (Figure 4). Since there aren’t enough user interactions for a particular item, this MovieLens 100K Dataset, therefore, faces the problem of new item cold start.
|
ICACT20200192 Slide.12
[Big slide for presentation]
[YouTube] |
Chrome Text-to-Speach Click!! |
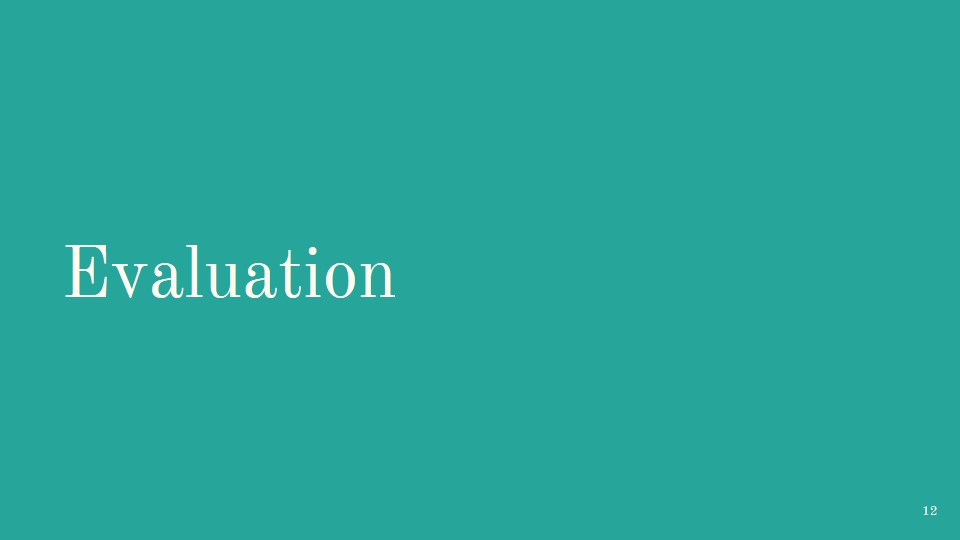 |
Evaluation
|
ICACT20200192 Slide.11
[Big slide for presentation]
[YouTube] |
Chrome Text-to-Speach Click!! |
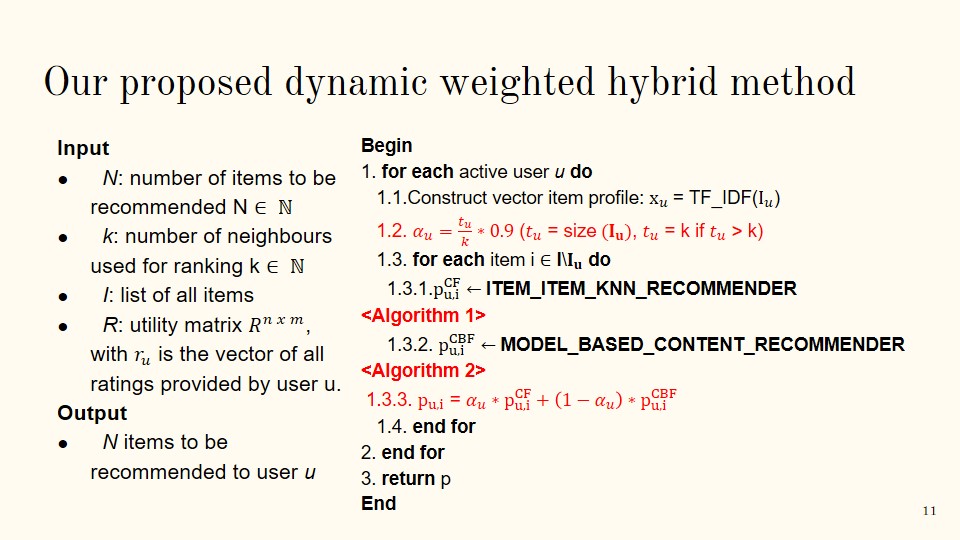 |
We are interested in combining 2 recommendation techniques, the computation of prediction score is established in step 1.3.3:
p_(u,i)= 𝛼_𝑢∗p_(u,i)^CF+(1−𝛼_𝑢 )∗p_(u,i)^CBF
Different from some related works, our contribution is that we form a simple calculation for 𝛼 with respect to a confidence factor. 𝛼 will be given in step 1.2:
𝛼_𝑢=𝑡_𝑢/𝑘∗0.9
, where k: number of neighbours used for ranking k ∈ℕ, and 𝑡_𝑢 is the number of items ∈ I\𝐈_𝐮. Note that, in case that 𝑡_𝑢 is greater than k, set〖 𝑡〗_𝑢= k. We called the ratio between 𝑡_𝑢 and b as a confidence factor for each user u.
|
ICACT20200192 Slide.10
[Big slide for presentation]
[YouTube] |
Chrome Text-to-Speach Click!! |
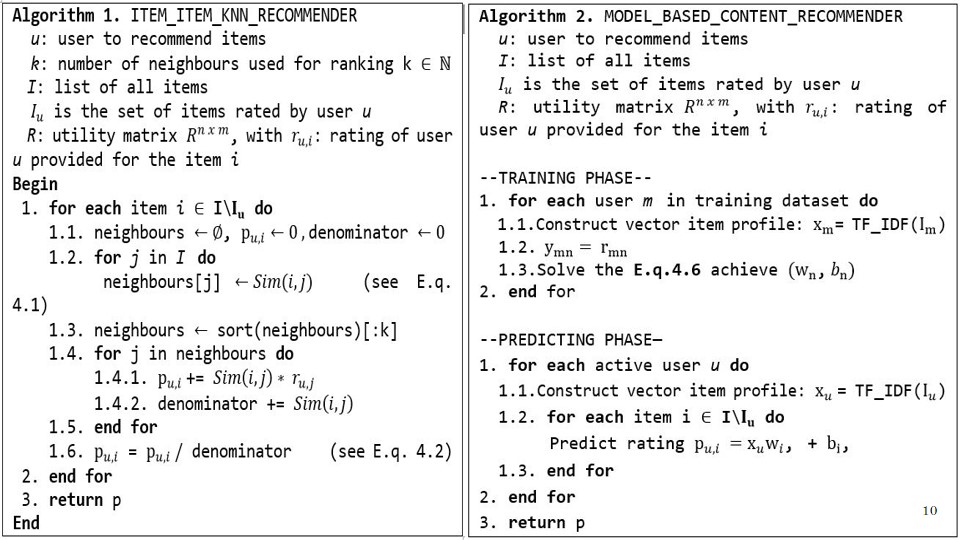 |
Research aims to improve the quality and accuracy of recommendation and address the new item problem by linearly combining Content-Based and Collaborative Filtering techniques.
Different from the related works, we form a simple calculation for α with respect to a confidence factor.
α is calculated from a confidence factor for each item, so that, the calculation of prediction takes more parts from Collaborative Filtering or Content-based Filtering depending on such confidence level
Proposed method is also straightforward enough to be implemented in a real application
|
ICACT20200192 Slide.09
[Big slide for presentation]
[YouTube] |
Chrome Text-to-Speach Click!! |
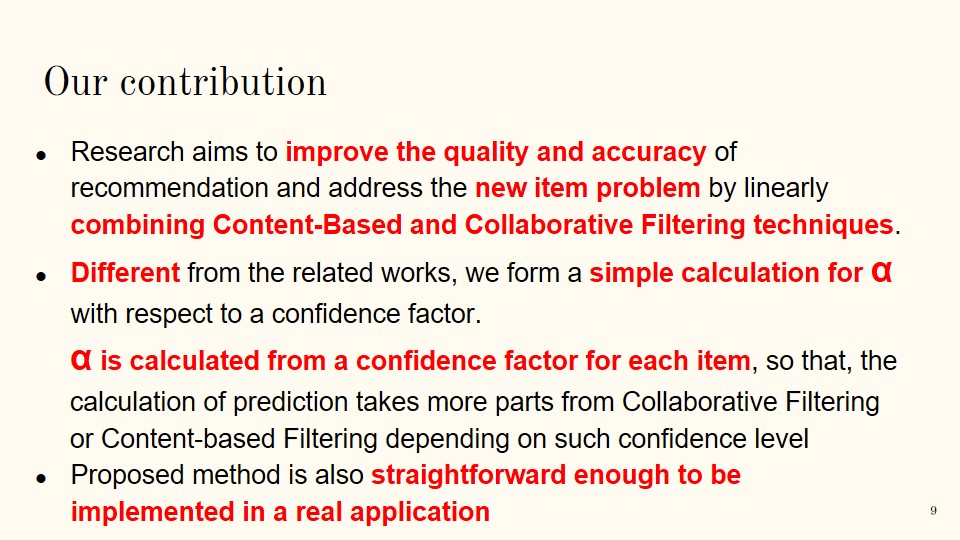 |
Research aims to improve the quality and accuracy of recommendation and address the new item problem by linearly combining Content-Based and Collaborative Filtering techniques.
Different from the related works, we form a simple calculation for α with respect to a confidence factor.
α is calculated from a confidence factor for each item, so that, the calculation of prediction takes more parts from Collaborative Filtering or Content-based Filtering depending on such confidence level
Proposed method is also straightforward enough to be implemented in a real application
|
ICACT20200192 Slide.08
[Big slide for presentation]
[YouTube] |
Chrome Text-to-Speach Click!! |
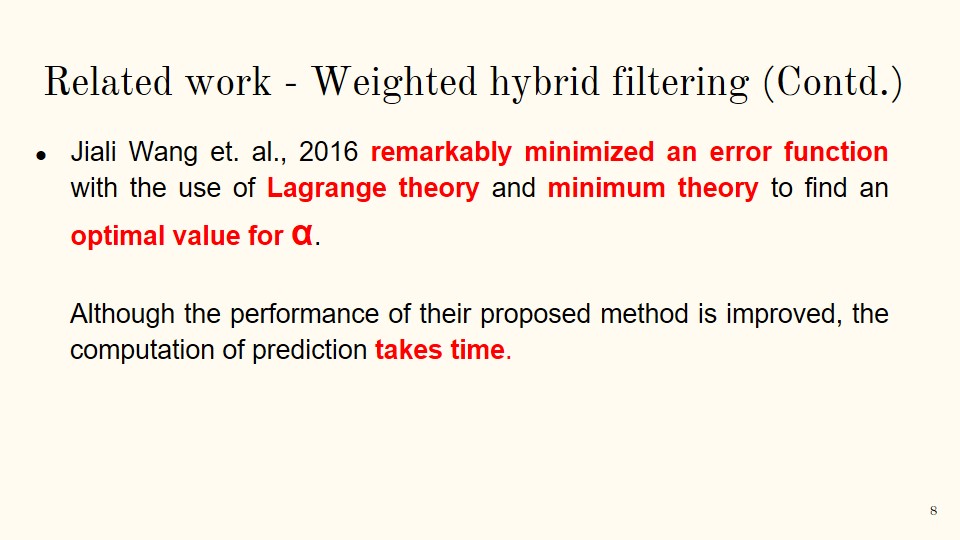 |
Jiali Wang et. al., 2016 remarkably minimized an error function with the use of Lagrange theory and minimum theory to find an optimal value for α.
Although the performance of their proposed method is improved, the computation of prediction takes time.
|
ICACT20200192 Slide.07
[Big slide for presentation]
[YouTube] |
Chrome Text-to-Speach Click!! |
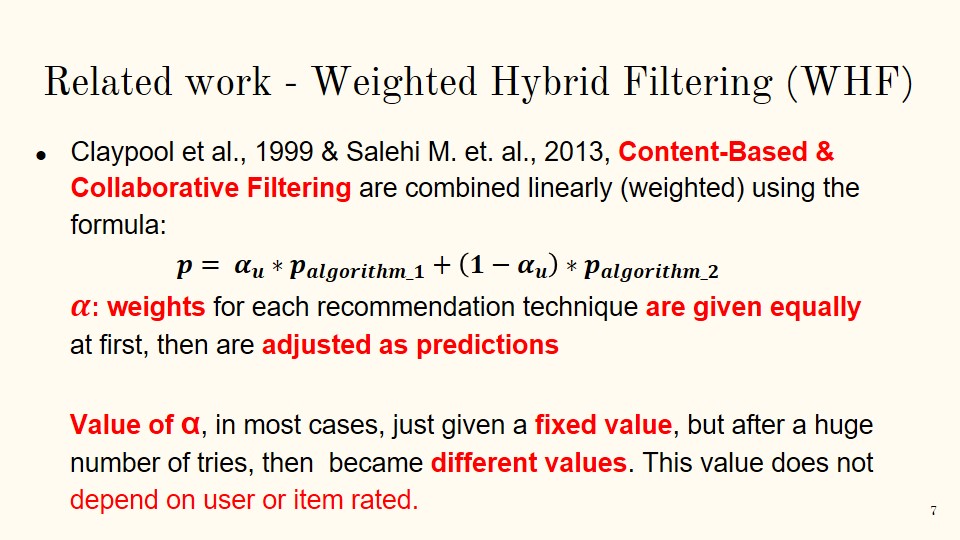 |
The two approaches: Collaborative filtering and Content-based filtering can also be combined as hybrid recommender systems.
(Contd.)<above>
|
ICACT20200192 Slide.06
[Big slide for presentation]
[YouTube] |
Chrome Text-to-Speach Click!! |
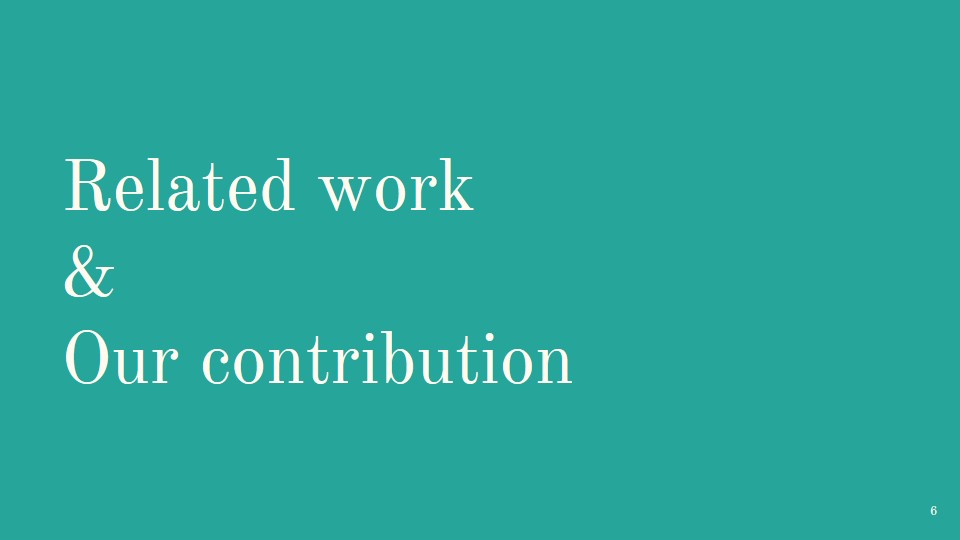 |
Related work & Our contribution
|
ICACT20200192 Slide.05
[Big slide for presentation]
[YouTube] |
Chrome Text-to-Speach Click!! |
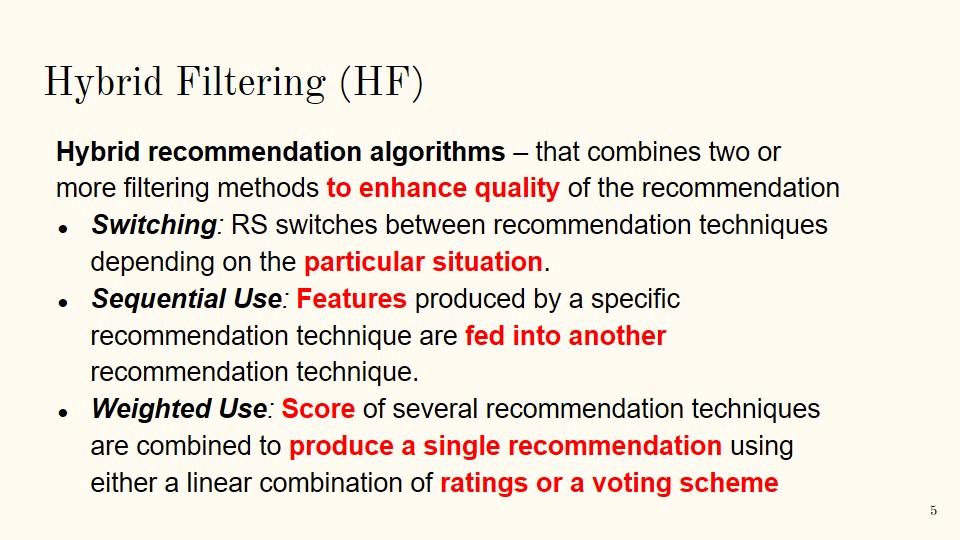 |
Due to these limitations in each single recommender technique, new approaches that combines two or more filtering receive tons of attentions. These approaches are named Hybrid filtering. By combining multiple filters into a hybrid filtering, it aims to enhance quality of the recommendation.
(Contd.)<above>
|
ICACT20200192 Slide.04
[Big slide for presentation]
[YouTube] |
Chrome Text-to-Speach Click!! |
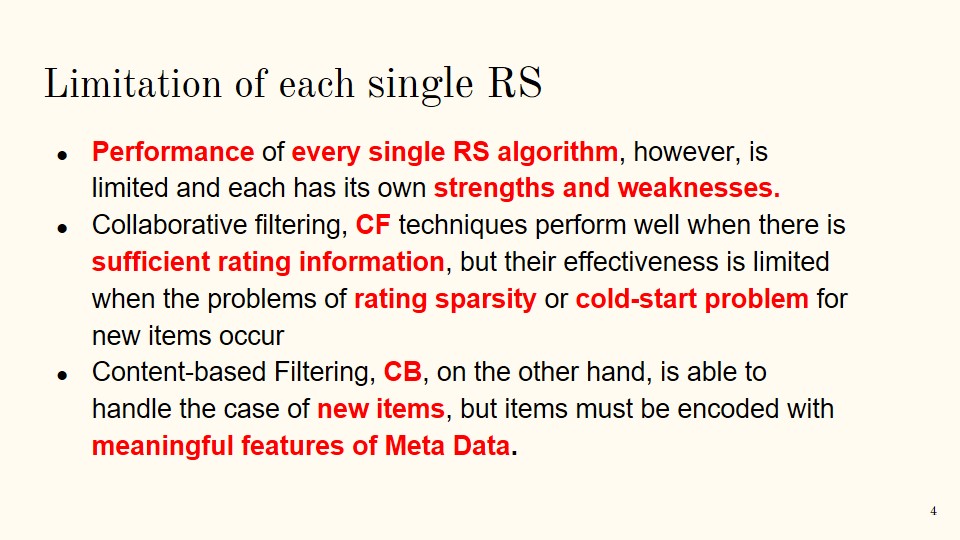 |
<above>
Limitation of each single RS: Recommendation System
The performance of every single recommendation algorithm, however, is limited and each has its own strengths and weaknesses.
Collaborative filtering techniques perform well when there is sufficient rating information, but their effectiveness is limited when the problems of rating sparsity or cold-start problem for new items occur
CBF, on the other hand, is able to handle the case of new items, but items must be encoded with meaningful features.
|
ICACT20200192 Slide.03
[Big slide for presentation]
[YouTube] |
Chrome Text-to-Speach Click!! |
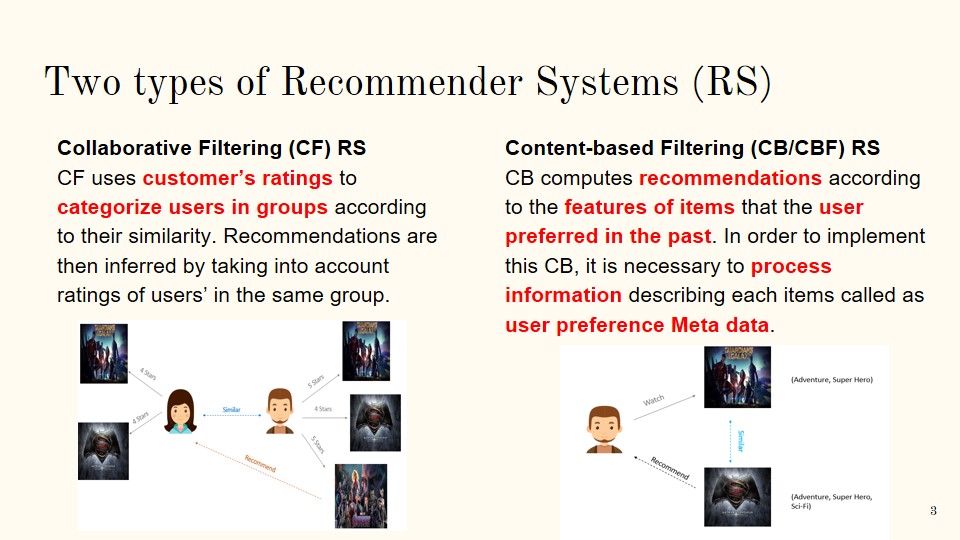 |
Two of the most familiar and successful recommendation techniques are probably Collaborative filtering (CF) and Content-based filtering (CBF).
- Collaborative filtering recommender systems is the most mature and the most commonly implemented in different application areas, for example Amazon since 1998. The filtering uses customer’s ratings to categorize users in groups according to their similarity. Recommendations are then inferred by taking into account ratings of users’ in the same group.
- In contrast to CF, Content-based recommender systems computes recommendations according to the features of items that the user preferred in the past.
The main difference compared to collaborative filtering is that the content based approach offers the recommendation based on similarity not only by rating, but also the information from the products i.e., the movie title, the year, the actors, the genre, story. Thus, in order to implement this CB, it is necessary to process information describing each item.
|
ICACT20200192 Slide.02
[Big slide for presentation]
[YouTube] |
Chrome Text-to-Speach Click!! |
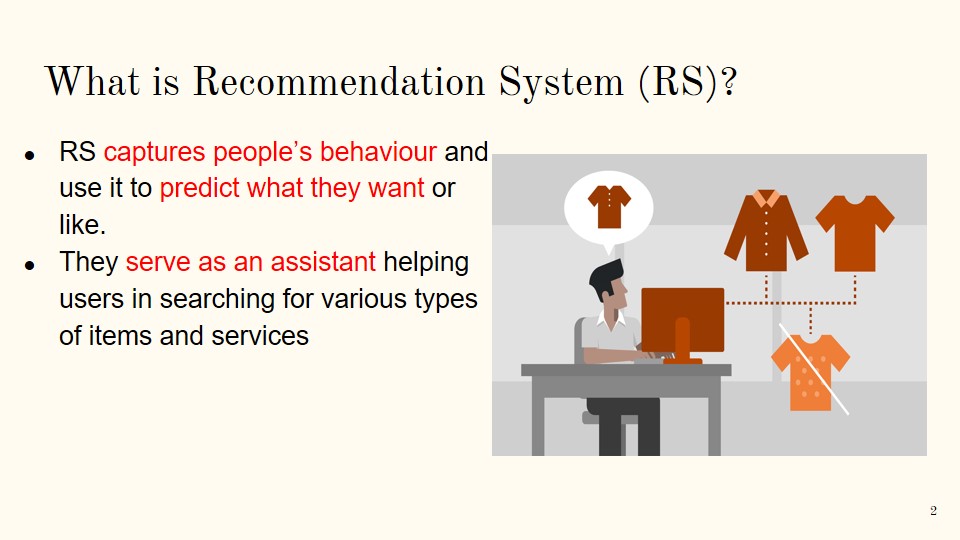 |
With the exponential growth
of the digital information
in the recent decades,
recommender systems are now an integral significant part
of any modern system.
Recommender systems capture the pattern
of people’s behaviour
and use it to predict
what else they might want
or like.
They serve as an assistant
helping users
in searching for various types
of items and services.
|
ICACT20200192 Slide.01
[Big slide for presentation]
[YouTube] |
Chrome Text-to-Speach Click!! |
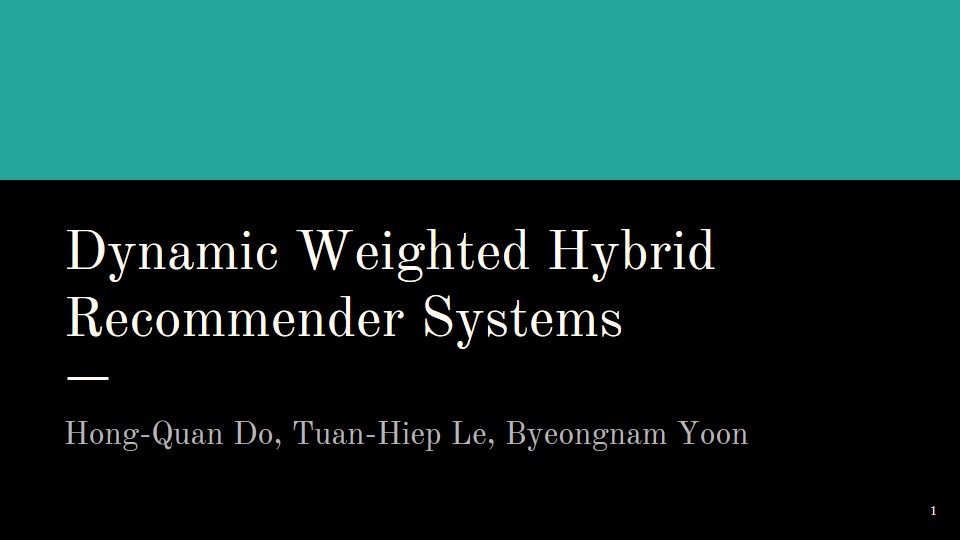 |
Hi everybody, my name is Byeongnam Yoon. For ICACT 2020, I have cooperated with two partners from ITI VNU. Mr. Do Hong Quan and FPT University, Vietnam. and Mr. Le Tuan Hiep.
Our presentation today is about a solution for Recommender Systems, that we called Dynamic Weighted Hybrid Recommender Systems.
|